Smoothed Analysis of Sequential Probability Assignment
arXiv (Cornell University)(2023)
摘要
We initiate the study of smoothed analysis for the sequential probability assignment problem with contexts. We study information-theoretically optimal minmax rates as well as a framework for algorithmic reduction involving the maximum likelihood estimator oracle. Our approach establishes a general-purpose reduction from minimax rates for sequential probability assignment for smoothed adversaries to minimax rates for transductive learning. This leads to optimal (logarithmic) fast rates for parametric classes and classes with finite VC dimension. On the algorithmic front, we develop an algorithm that efficiently taps into the MLE oracle, for general classes of functions. We show that under general conditions this algorithmic approach yields sublinear regret.
更多查看译文
关键词
assignment
AI 理解论文
溯源树
样例
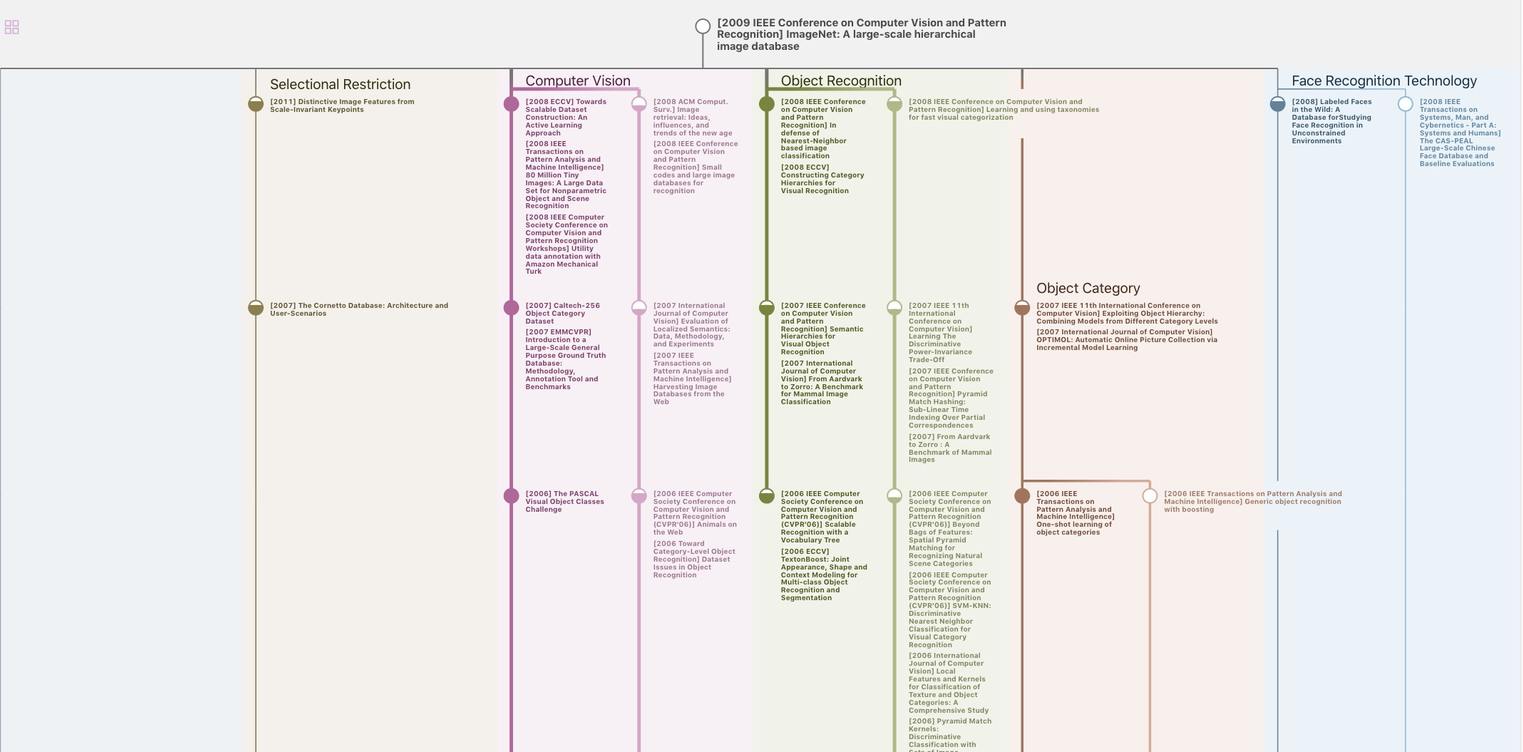
生成溯源树,研究论文发展脉络
Chat Paper
正在生成论文摘要