Pose Relations: Learning Physical Properties for the Digital Buildability Validation of Fasteners
2022 7th International Conference on Robotics and Automation Engineering (ICRAE)(2022)
摘要
Validating the buildability of a complex product on the basis of its digital geometric prototype is essential in order to detect problems early during development. In general, overlaping components are potentially problematic. Especially fasteners cause many of these intersections. To validate an intersection involving a fastener requires to check the relative position of the fastener to its counterpart (pose relation). The pose relation is uncritical if the fastener is sufficiently centered in the corresponding hole of its counterpart. This goes far beyond a geometric question because the tolerances with regard to centering depend on the individual fastener and its physical properties. Since this validation requires a high degree of technical know-how it is currently done manually by engineers with high effort. In this paper, we present an automated solution for the classification of pose relations involving fasteners. Our approach maps the components to images using cylindrical projections. These are passed to a Convolutional Neural Network (CNN) which classifies the pose relation into critical or non-critical. During training, the labeling of the images implicitly gives the CNN the physical knowledge about the individual tolerances of the fasteners. In our experiments, we show that the CNN is able to generalize the physical properties and to transfer them to pose relations involving new fasteners, not seen in training.
更多查看译文
关键词
digital buildability validation,automation,3D classification,supervised learning
AI 理解论文
溯源树
样例
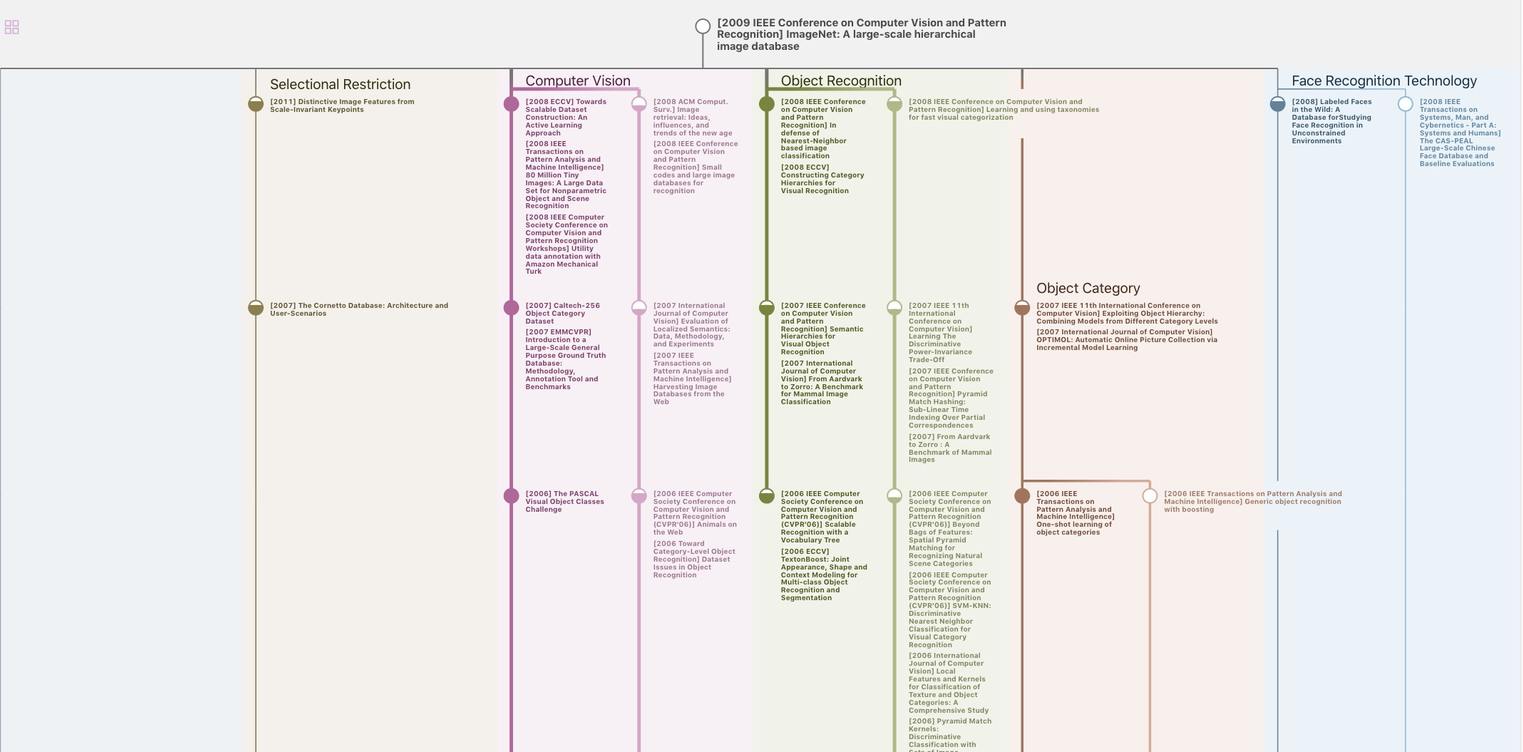
生成溯源树,研究论文发展脉络
Chat Paper
正在生成论文摘要