Reward Shaping-Based Actor–Critic Deep Reinforcement Learning for Residential Energy Management
IEEE Transactions on Industrial Informatics(2023)
摘要
Residential energy consumption continues to climb steadily, requiring intelligent energy management strategies to reduce power system pressures and residential electricity bills. However, it is challenging to design such strategies due to the random nature of electricity pricing, appliance demand, and user behavior. This article presents a novel reward shaping (RS)-based actor–critic deep reinforcement learning (ACDRL) algorithm to manage the residential energy consumption profile with limited information about the uncertain factors. Specifically, the interaction between the energy management center and various residential loads is modeled as a Markov decision process that provides a fundamental mathematical framework to represent the decision-making in situations where outcomes are partially random and partially influenced by the decision-maker control signals, in which the key elements containing the agent, environment, state, action, and reward are carefully designed, and the electricity price is considered as a stochastic variable. An RS-ACDRL algorithm is then developed, incorporating both the actor and critic network and an RS mechanism, to learn the optimal energy consumption schedules. Several case studies involving real-world data are conducted to evaluate the performance of the proposed algorithm. Numerical results demonstrate that the proposed algorithm outperforms state-of-the-art RL methods in terms of learning speed, solution optimality, and cost reduction.
更多查看译文
关键词
Deep deterministic policy gradient,deep reinforcement learning,demand response,residential energy management,reward shaping (RS)
AI 理解论文
溯源树
样例
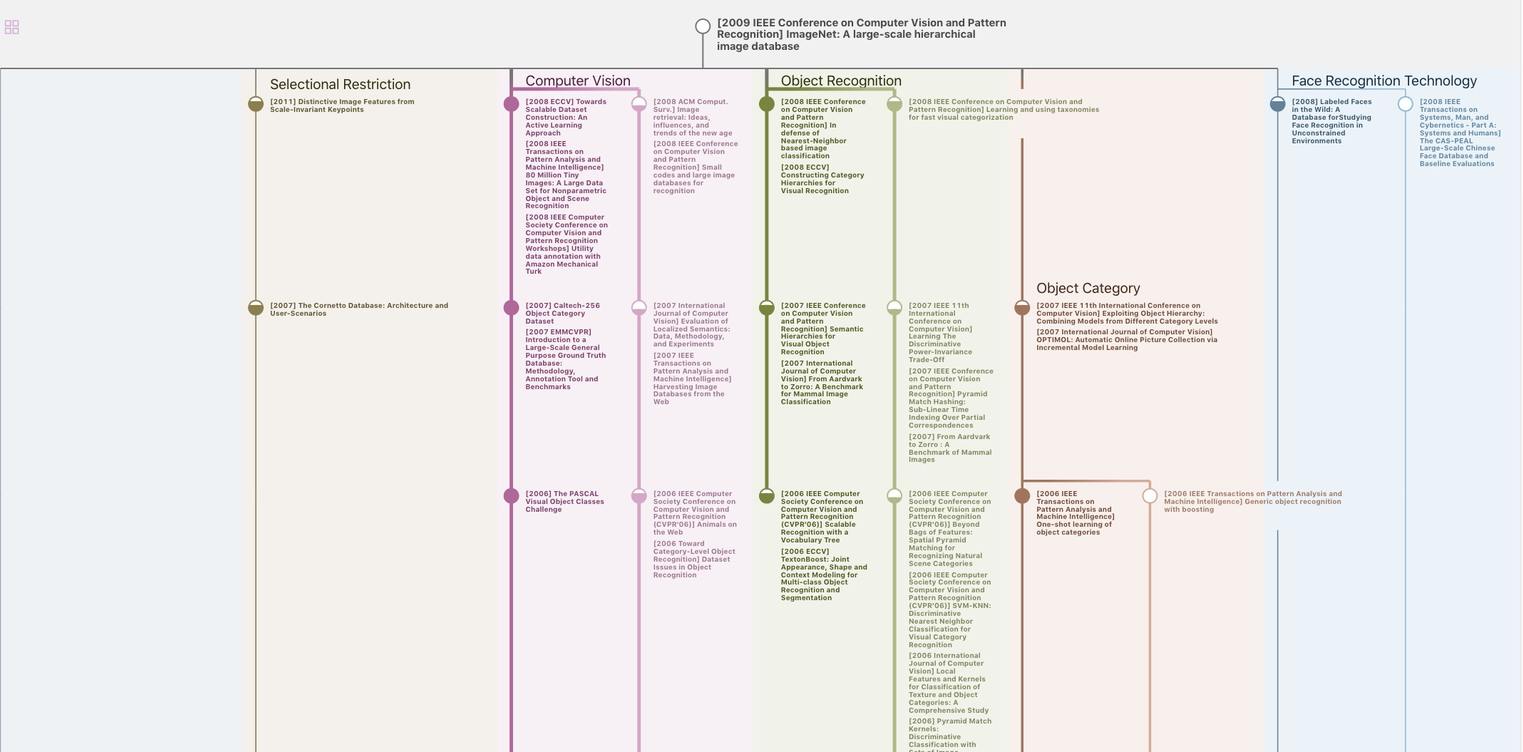
生成溯源树,研究论文发展脉络
Chat Paper
正在生成论文摘要