A Bayesian approach to estimating COVID-19 incidence and infection fatality rates.
Biostatistics (Oxford, England)(2023)
摘要
Naive estimates of incidence and infection fatality rates (IFR) of coronavirus disease 2019 suffer from a variety of biases, many of which relate to preferential testing. This has motivated epidemiologists from around the globe to conduct serosurveys that measure the immunity of individuals by testing for the presence of SARS-CoV-2 antibodies in the blood. These quantitative measures (titer values) are then used as a proxy for previous or current infection. However, statistical methods that use this data to its full potential have yet to be developed. Previous researchers have discretized these continuous values, discarding potentially useful information. In this article, we demonstrate how multivariate mixture models can be used in combination with post-stratification to estimate cumulative incidence and IFR in an approximate Bayesian framework without discretization. In doing so, we account for uncertainty from both the estimated number of infections and incomplete deaths data to provide estimates of IFR. This method is demonstrated using data from the Action to Beat Coronavirus erosurvey in Canada.
更多查看译文
关键词
Bayesian analysis,COVID-19,Mixture model,Modular inference,Post-stratification
AI 理解论文
溯源树
样例
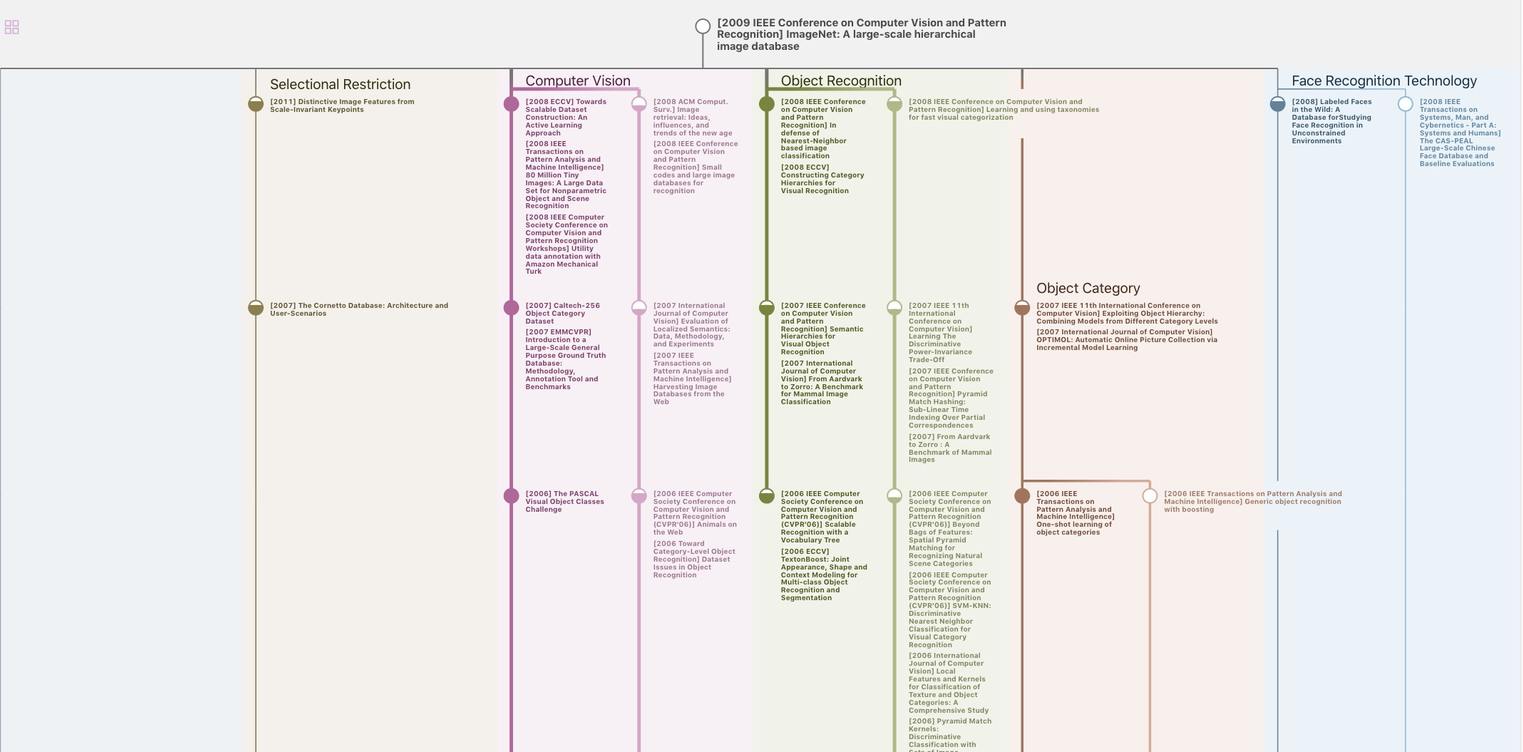
生成溯源树,研究论文发展脉络
Chat Paper
正在生成论文摘要