Model Predictive Control with Gaussian-Process-Supported Dynamical Constraints for Autonomous Vehicles
IFAC PAPERSONLINE(2023)
摘要
We propose a model predictive control approach for autonomous vehicles that exploits learned Gaussian processes (GPs) for predicting human driving behavior. The proposed approach employs the uncertainty about the GP's prediction to achieve safety. The multi-mode predictive control approach considers the possible intentions of the human drivers. While the intentions are represented by different Gaussian processes, their probabilities foreseen in the observed behaviors are determined by a suitable online classification. Intentions below a certain probability threshold are neglected to improve performance. The proposed multi-mode model predictive control approach with Gaussian process regression support enables probabilistic constraint satisfaction with high probability. The approach is underlined in simulation, considering real-world measurements for training the Gaussian processes. Copyright (c) 2023 The Authors.
更多查看译文
关键词
Gaussian Process based Identification and Control,Nonlinear Model Predictive Control,Gaussian Processes,Robust Control,Intelligent Autonomous Vehicles
AI 理解论文
溯源树
样例
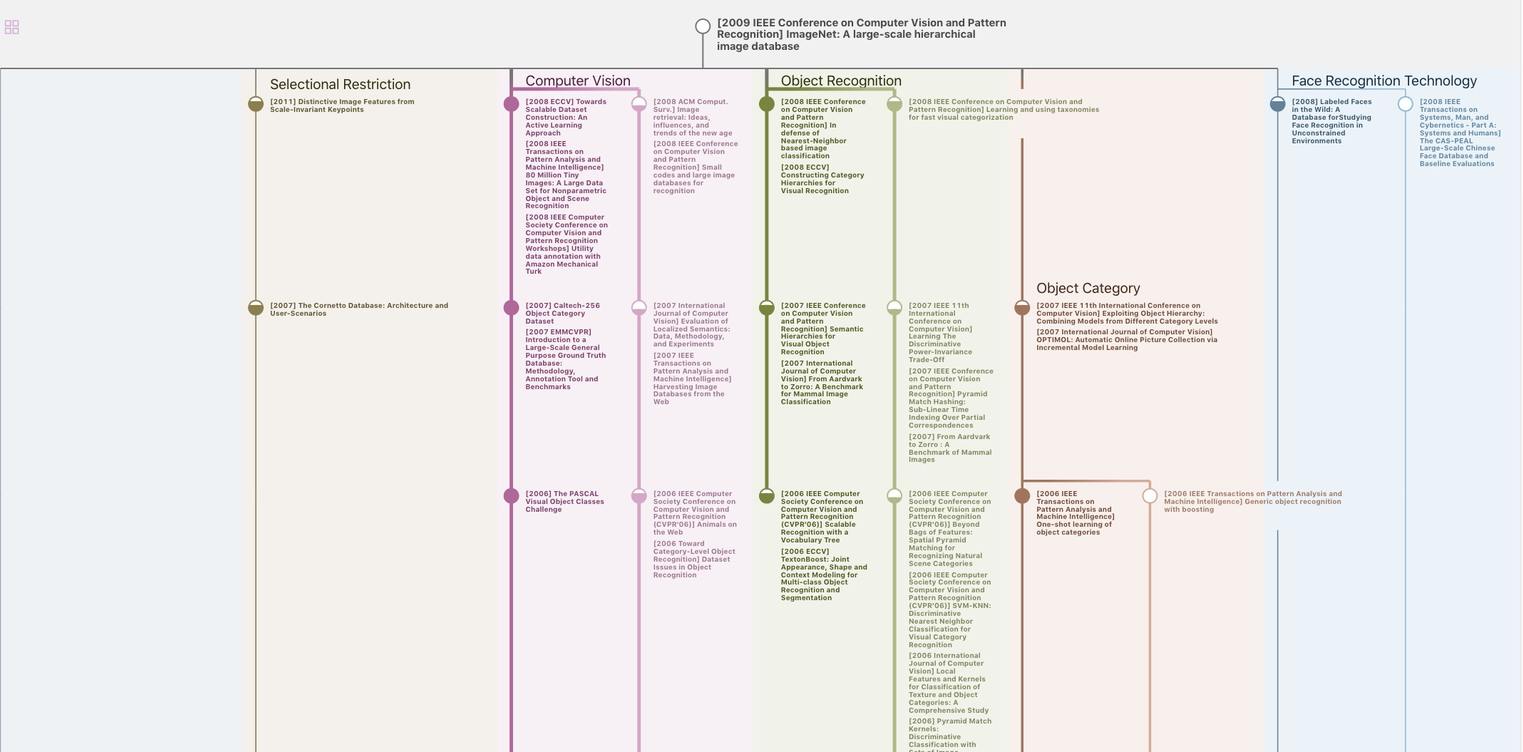
生成溯源树,研究论文发展脉络
Chat Paper
正在生成论文摘要