Dynamic Scenario Representation Learning for Motion Forecasting with Heterogeneous Graph Convolutional Recurrent Networks
arxiv(2023)
摘要
Due to the complex and changing interactions in dynamic scenarios, motion forecasting is a challenging problem in autonomous driving. Most existing works exploit static road graphs to characterize scenarios and are limited in modeling evolving spatio-temporal dependencies in dynamic scenarios. In this paper, we resort to dynamic heterogeneous graphs to model the scenario. Various scenario components including vehicles (agents) and lanes, multi-type interactions, and their changes over time are jointly encoded. Furthermore, we design a novel heterogeneous graph convolutional recurrent network, aggregating diverse interaction information and capturing their evolution, to learn to exploit intrinsic spatio-temporal dependencies in dynamic graphs and obtain effective representations of dynamic scenarios. Finally, with a motion forecasting decoder, our model predicts realistic and multi-modal future trajectories of agents and outperforms state-of-the-art published works on several motion forecasting benchmarks.
更多查看译文
关键词
Computer vision for automation,intelligent transportation systems,deep learning methods
AI 理解论文
溯源树
样例
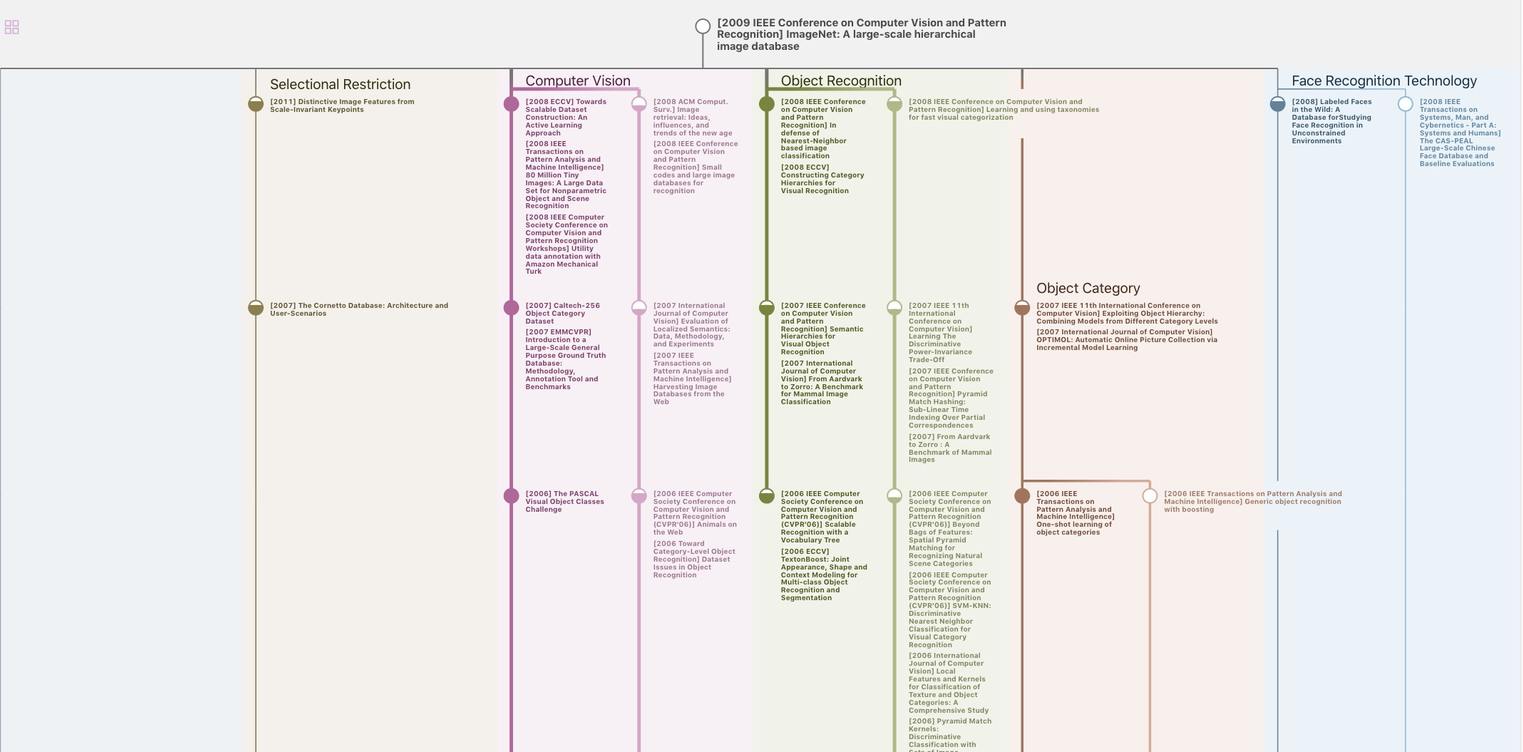
生成溯源树,研究论文发展脉络
Chat Paper
正在生成论文摘要