M-EBM: Towards Understanding the Manifolds of Energy-Based Models
arxiv(2023)
摘要
Energy-based models (EBMs) exhibit a variety of desirable properties in predictive tasks, such as generality, simplicity and compositionality. However, training EBMs on high-dimensional datasets remains unstable and expensive. In this paper, we present a Manifold EBM (M-EBM) to boost the overall performance of unconditional EBM and Joint Energy-based Model (JEM). Despite its simplicity, M-EBM significantly improves unconditional EBMs in training stability and speed on a host of benchmark datasets, such as CIFAR10, CIFAR100, CelebA-HQ, and ImageNet 32x32. Once class labels are available, label-incorporated M-EBM (M-JEM) further surpasses M-EBM in image generation quality with an over 40% FID improvement, while enjoying improved accuracy. The code can be found at https://github.com/sndnyang/mebm.
更多查看译文
关键词
models,manifolds,m-ebm,energy-based
AI 理解论文
溯源树
样例
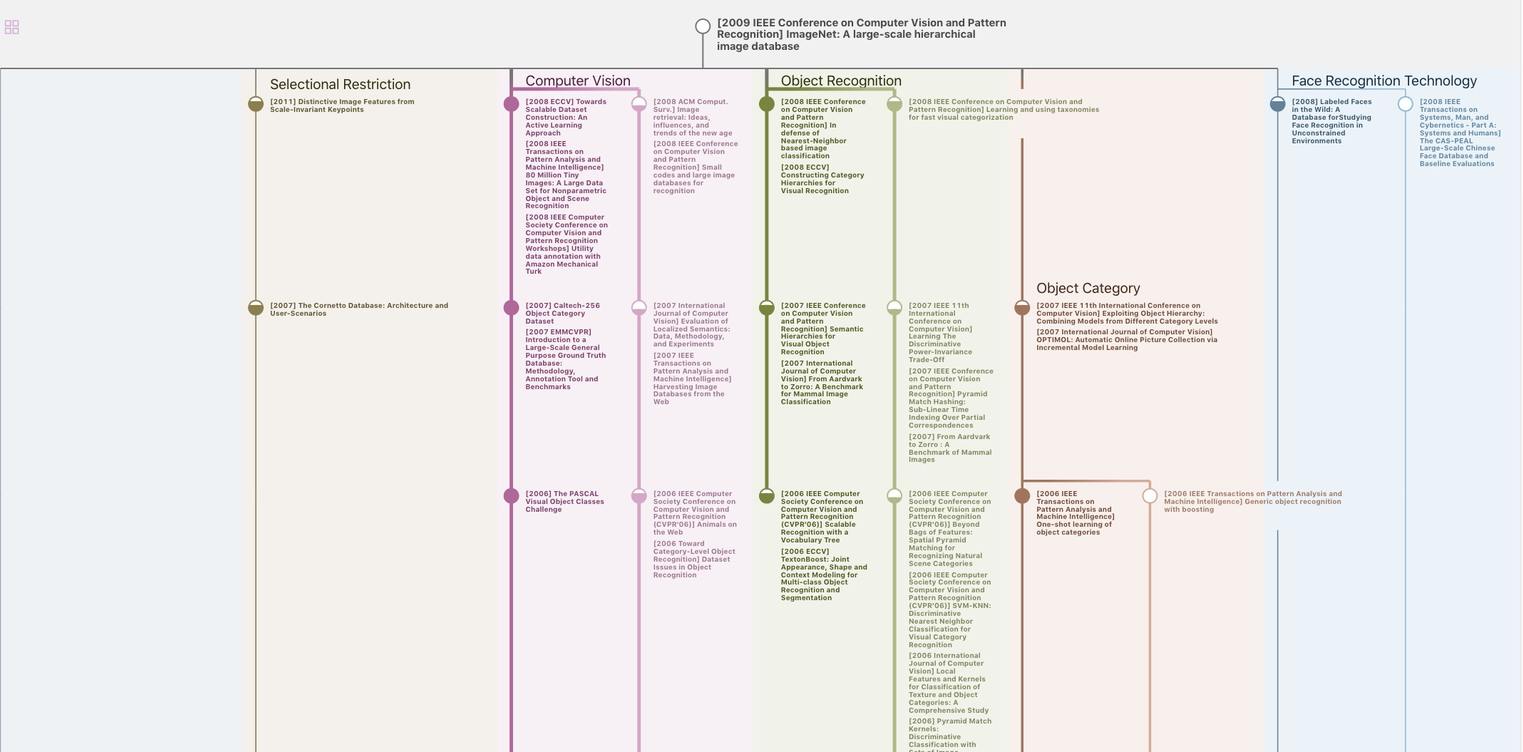
生成溯源树,研究论文发展脉络
Chat Paper
正在生成论文摘要