Glucose Transformer: Forecasting Glucose Level and Events of Hyperglycemia and Hypoglycemia
IEEE Journal of Biomedical and Health Informatics(2023)
摘要
To avoid the adverse consequences from abrupt increases in blood glucose, diabetic inpatients should be closely monitored. Using blood glucose data from type 2 diabetes patients, we propose a deep learning model-based framework to forecast blood glucose levels. We used continuous glucose monitoring (CGM) data collected from inpatients with type 2 diabetes for a week. We adopted the Transformer model, commonly used in sequence data, to forecast the blood glucose level over time and detect hyperglycemia and hypoglycemia in advance. We expected the attention mechanism in Transformer to reveal a hint of hyperglycemia and hypoglycemia, and performed a comparative study to determine whether Transformer was effective in the classification and regression of glucose. Hyperglycemia and hypoglycemia rarely occur and this results in an imbalance in the classification. We built a data augmentation model using the generative adversarial network. Our contributions are as follows. First, we developed a deep learning framework utilizing the encoder part of Transformer to perform the regression and classification under a unified framework. Second, we adopted a data augmentation model using the generative adversarial network suitable for time-series data to solve the data imbalance problem and to improve performance. Third, we collected data for type 2 diabetic inpatients for mid-time. Finally, we incorporated transfer learning to improve the performance of regression and classification.
更多查看译文
关键词
Type 2 diabetes,continuous glucose monitoring,hyperglycemia,hypoglycemia,deep learning,Transformer
AI 理解论文
溯源树
样例
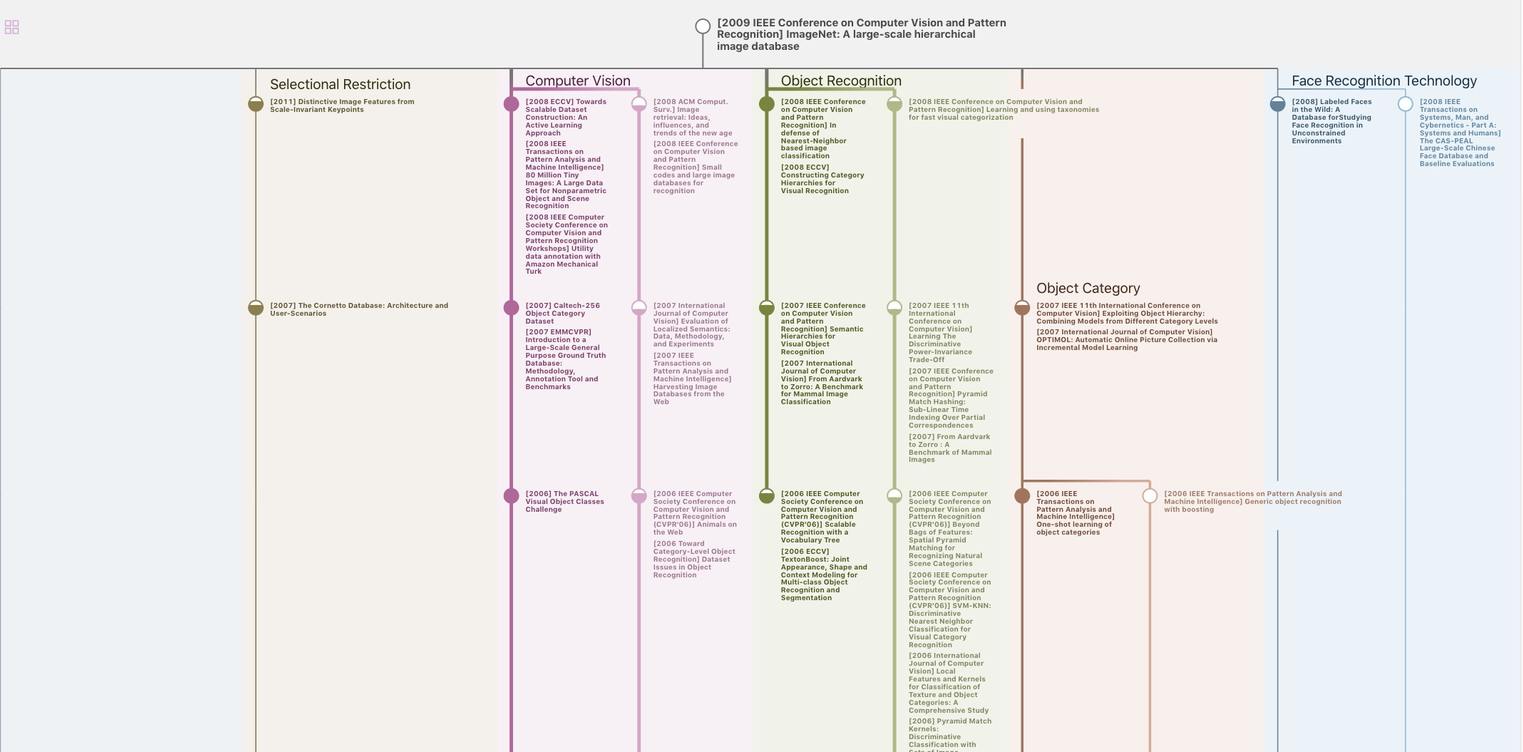
生成溯源树,研究论文发展脉络
Chat Paper
正在生成论文摘要