A Multi-Modal Heterogeneous Graph Forest to Predict Lymph Node Metastasis of Non-Small Cell Lung Cancer
IEEE Journal of Biomedical and Health Informatics(2023)
摘要
Lymph node metastasis (LNM) is critical for treatment decision-making for cancer patients, but it is difficult to diagnose accurately before surgery. Machine learning can learn nontrivial knowledge from multi-modal data to support accurate diagnosis. In this paper, we proposed a Multi-modal Heterogeneous Graph Forest (MHGF) approach to extract the deep representations of LNM from multi-modal data. Specifically, we first extracted the deep image features from CT images to represent the pathological anatomic extent of the primary tumor (pathological T stage) using a ResNet-Trans network. And then, a heterogeneous graph with six vertices and seven bi-directional relations was defined by medical experts to describe the possible relations between the clinical and image features. After that, we proposed a graph forest approach to construct the sub-graphs by removing each vertex in the complete graph iteratively. Finally, we used graph neural networks to learn the representations of each sub-graph in the forest to predict LNM and averaged all the prediction results as final results. We conducted experiments on 681 patients' multi-modal data. The proposed MHGF achieves the best performances with a 0.806 AUC value and 0.513 AP value compared with state-of-art machine learning and deep learning methods. The results indicate that the graph method can explore the relations between different types of features to learn effective deep representations for LNM prediction. Moreover, we found that the deep image features about the pathological anatomic extent of the primary tumor are useful for LNM prediction. And the graph forest approach can further improve the generalization ability and stability of the LNM prediction model.
更多查看译文
关键词
Electronic medical records,hetero- geneous graph,lymph node metastasis prediction,multi-modal data fusion
AI 理解论文
溯源树
样例
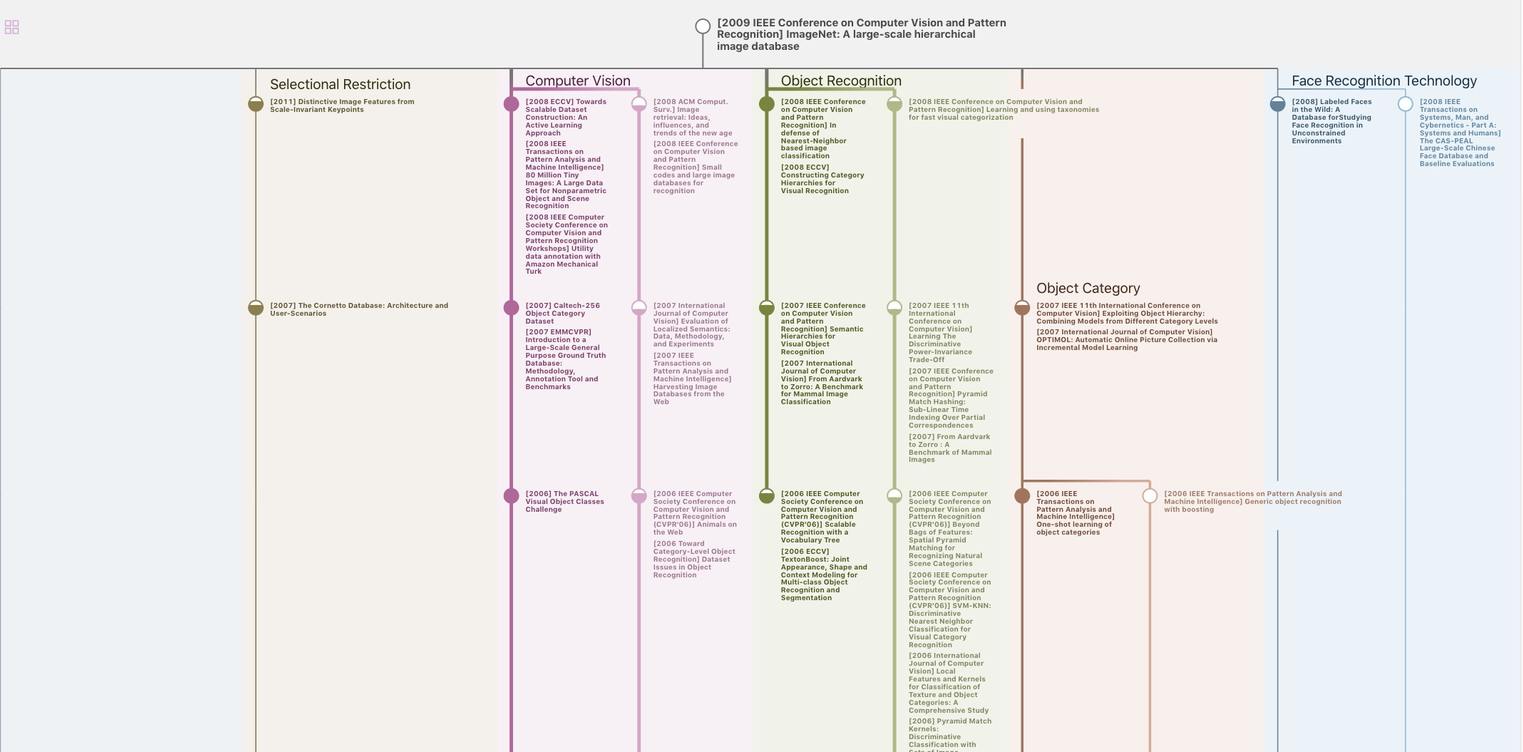
生成溯源树,研究论文发展脉络
Chat Paper
正在生成论文摘要