Real-Time Gait Phase Detection on Wearable Devices for Real-World Free-Living Gait.
IEEE J. Biomed. Health Informatics(2023)
摘要
Detecting gait phases with wearables unobtrusively and reliably in real-time is important for clinical gait rehabilitation and early diagnosis of neurological diseases. Due to hardware limitations of microcontrollers in wearable devices (e.g., memory and computation power), reliable real-time gait phase detection on the microcontrollers remains a challenge, especially for long-term real-world free-living gait. In this work, a novel algorithm based on a reduced support vector machine (RSVM) and a finite state machine (FSM) is developed to address this. The RSVM is developed by exploiting the cascaded K-means clustering to reduce the model size and computation time of a standard SVM by 88% and a factor of 36, with only minor degradation in gait phase prediction accuracy of around 4%. For each gait phase prediction from the RSVM, the FSM is designed to validate the prediction and correct misclassifications. The developed algorithm is implemented on a microcontroller of a wearable device and its real-time (on the fly) classification performance is evaluated by twenty healthy subjects walking along a predefined real-world route with uncontrolled free-living gait. It shows a promising real-time performance with an accuracy of 91.51%, a sensitivity of 91.70%, and a specificity of 95.77%. The algorithm also demonstrates its robustness with varying walking conditions.
更多查看译文
关键词
Real-time gait phase detection,embedded system algorithms,wearable sensors,gait rehabilitation,real-world free-living walking
AI 理解论文
溯源树
样例
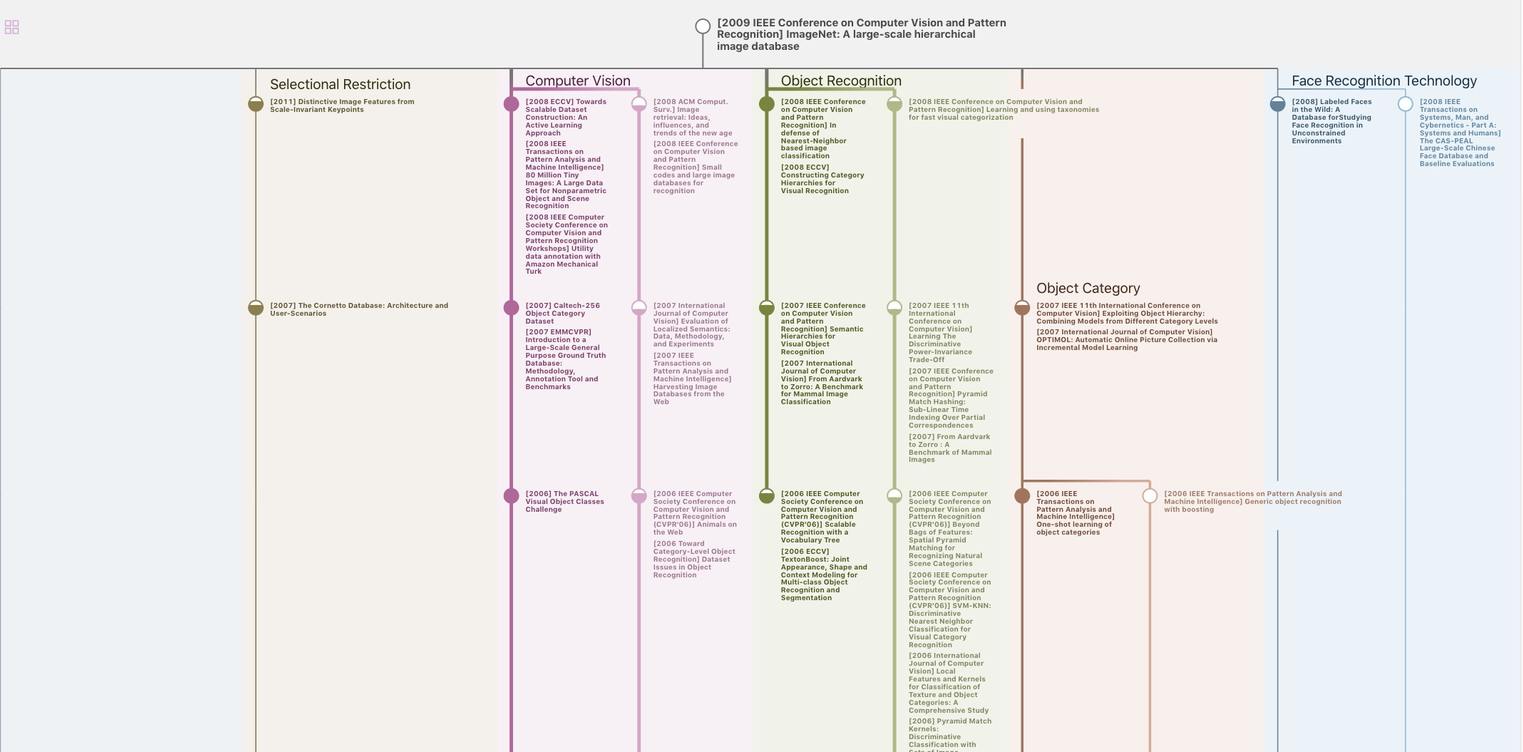
生成溯源树,研究论文发展脉络
Chat Paper
正在生成论文摘要