Towards Reconfigurable CNN Accelerator for FPGA Implementation
IEEE Transactions on Circuits and Systems II: Express Briefs(2023)
摘要
Convolutional Neural Networks (CNNs) have revolutionized many applications in recent years, especially in image classification, video processing, and pattern recognition. This success of CNNs has been a motivating factor for solving even more complex problems involving multiple data modalities. Traditionally, a single CNN accelerator has been optimized for just one task or has been used to perform correlated tasks. We leverage the CNNs capability to learn patterns and use one accelerator to perform multiple uncorrelated tasks from different modalities and achieve an average accuracy above 90%, which would otherwise require three accelerators. Two types of CNN architectures (i.e., fused and branched) are evaluated for three distinct tasks based on accuracy, quantization, pruning, hardware resource utilization, power, and latency. Capitalizing on this, we have further proposed a runtime reconfigurable CNN accelerator supporting fault-tolerant (FT), high-performance (HP), and de-stress (DS) modes.
更多查看译文
关键词
Multi-task learning,multi-modal learning,FPGAs,convolutional neural network,reliability,reconfigurability
AI 理解论文
溯源树
样例
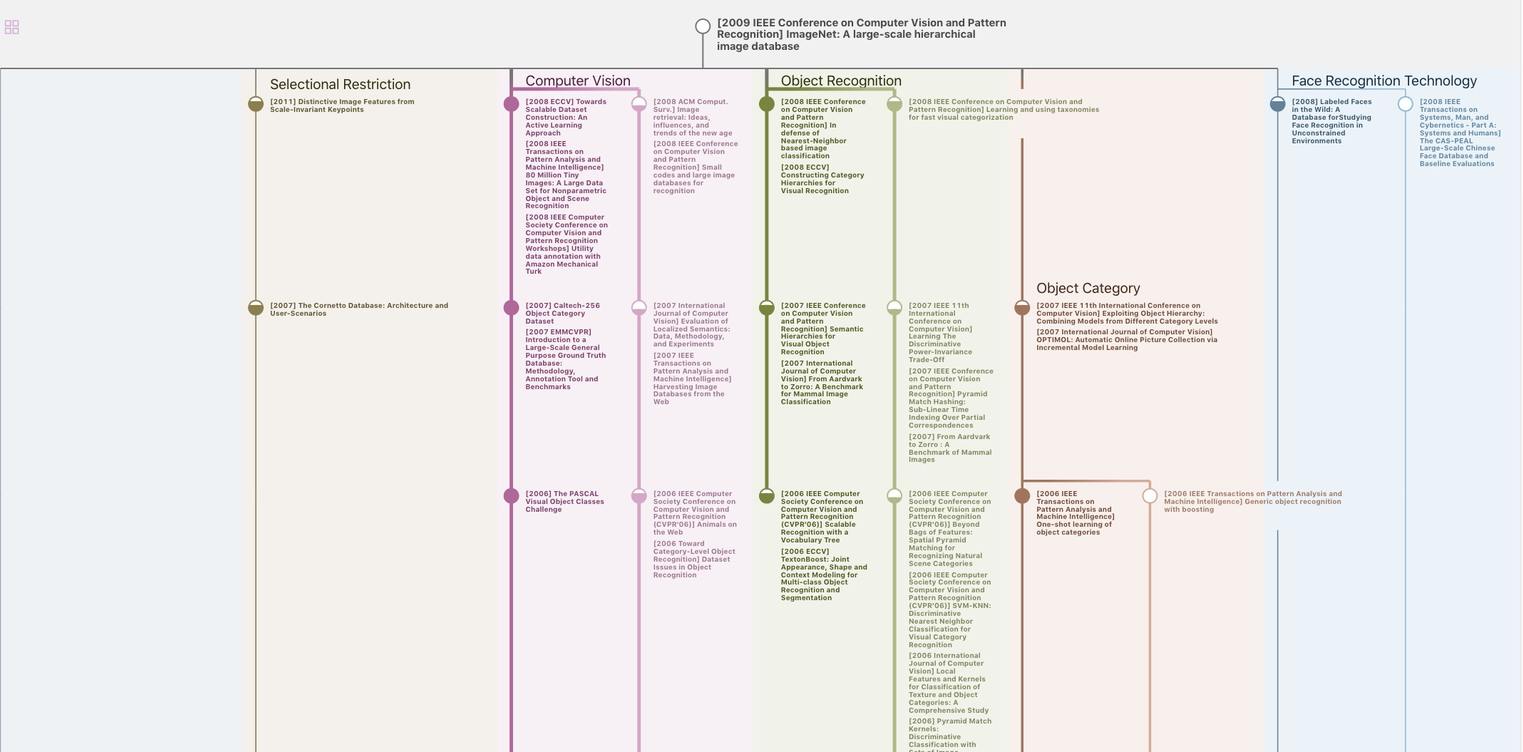
生成溯源树,研究论文发展脉络
Chat Paper
正在生成论文摘要