Using classification trees to identify psychotherapy patients at risk for poor treatment adherence
PSYCHOTHERAPY RESEARCH(2024)
摘要
ObjectiveTo determine the relative importance of a wide variety of personality and psychopathology variables in influencing patients' adherence to psychotherapy treatment.MethodTwo classification trees were trained to predict patients' (1) treatment utilization (i.e., their likelihood of missing a given appointment) and (2) termination status (i.e., their likelihood of dropping out of therapy prematurely). Each tree was then validated in an external dataset to examine performance accuracy.ResultsPatients' social detachment was most influential in predicting their treatment utilization, followed by affective instability and activity/energy levels. Patients' interpersonal warmth was most influential in predicting their termination status, followed by levels of disordered thought and resentment. The overall accuracy rating for the tree for termination status was 71.4%, while the tree for treatment utilization had a 38.7% accuracy rating.ConclusionClassification trees are a practical tool for clinicians to determine patients at risk of premature termination. More research is needed to develop trees that predict treatment utilization with high accuracy across different types of patients and settings.
更多查看译文
关键词
classification trees,attendance,machine learning,dropout,psychotherapy
AI 理解论文
溯源树
样例
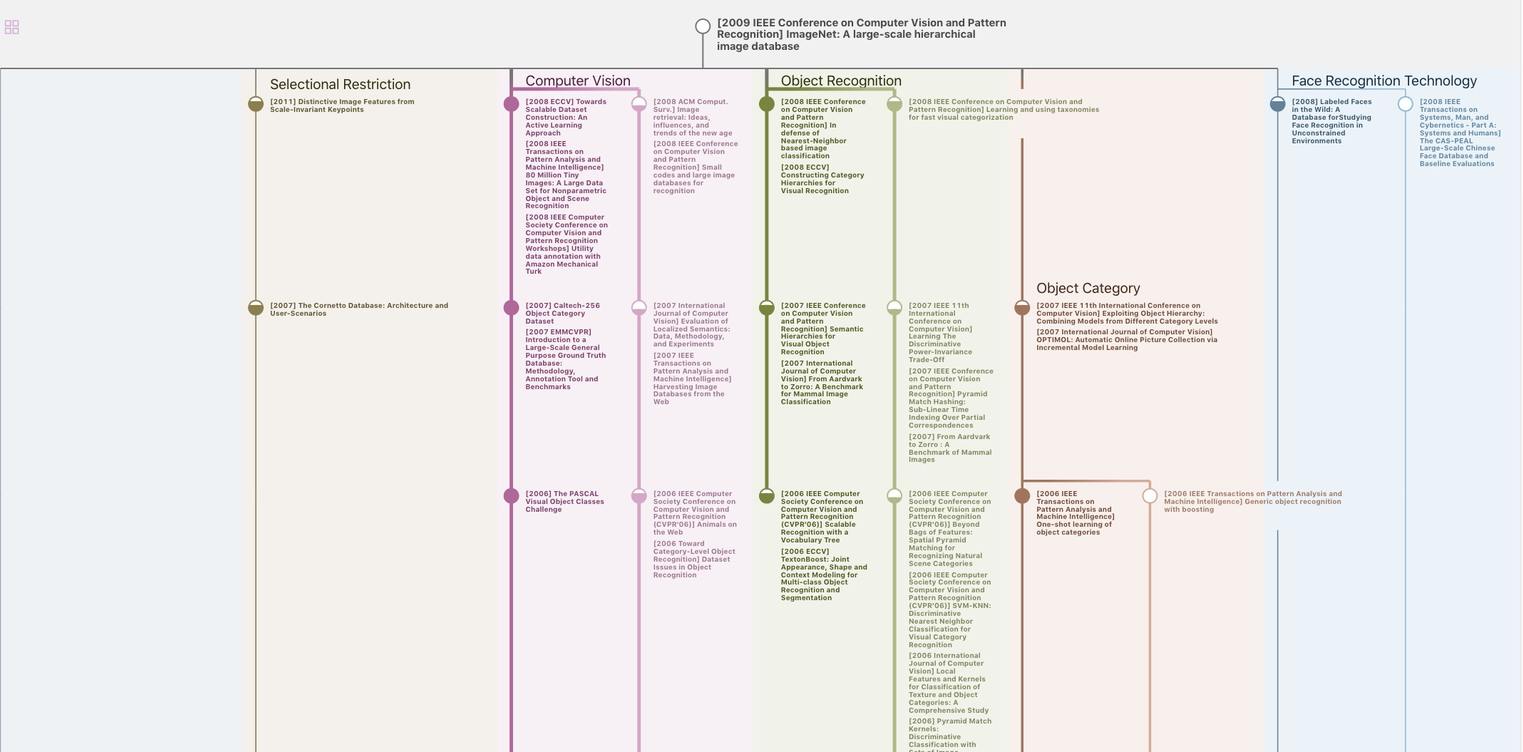
生成溯源树,研究论文发展脉络
Chat Paper
正在生成论文摘要