Applying artificial intelligence to the use of ultrasound as an educational tool: A focus on ultrasound-guided regional anesthesia.
Anatomical sciences education(2023)
摘要
I view it [AI] as the most profound technology humanity will ever work on … If you think about fire, electricity or the internet, it's like that but I think even more profound. Sundar Pichai, Alphabet/Google CEO1 Anatomy has long been a keystone of medical and surgical education. Applied anatomical knowledge can explain the phenomenon of referred pain during myocardial infarction, guide surgical intervention, and inform the interpretation of diagnostic imaging. Ultrasound (US) is a rapid, cheap, painless, non-invasive, and non-ionizing imaging modality.2 Its use is spreading beyond radiology in secondary care, to multiple other in-hospital specialties, pre-hospital emergency medicine, primary care, and even global public health and disaster medicine.3-5 It is a versatile technique, which can be used diagnostically and therapeutically.5 Modern cheaper and portable machines allow use at the point of care.6, 7 For these reasons, and due to its ability to reveal structures in their living context, it is being rapidly adopted within anatomy education.8 Though advances in US technology continue to provide enhanced image resolution,9 there can be identification errors of soft tissue structures.10 Progress in image acquisition and interpretation may not have matched developments in image generation.11 Recent technological advances in artificial intelligence (AI) may enhance the skill of interpreting US images.12 This article explores the role of AI in clinical anatomical education, through the medium of US. A specific example will be discussed, of which the authors have in-depth knowledge; the application to US-guided regional anesthesia (UGRA). Whilst anatomy remains fundamental to the practice of medicine, medical curricula have expanded with an ever-greater body of clinical knowledge,13 correlating with the emergence of shorter medical degrees. These constraints have reduced the time allocated for anatomical study and placed an emphasis on efficiency and integration of anatomy knowledge.14, 15 However, United Kingdom (UK) medical graduates have been reported to believe that their anatomy teaching is insufficient and would welcome additional instruction.16 Learning the necessary information during postgraduate training presents a challenge for busy clinicians. Concerns have been reported that the anatomical knowledge of medical graduates can be too limited, with associated safety issues related to the poor application of this knowledge.17 Thus, in the context of medical education, anatomy is in need of re-invigoration. Ultrasound is increasingly utilized within multi-modal anatomy teaching, by providing detailed imaging information in a non-invasive and repeatable manner.8, 18-21 As US becomes widely used in both undergraduate and graduate courses,7, 22-25 vertical integration is used to link early teaching and later clinical applications.18, 24 Students find it engaging and rewarding,21, 26, 27 and it can foster group learning.25 US combines anatomy with clinical relevance while developing a practical skill for future use.28, 29 Furthermore, innovative assessment processes employ US to examine students' knowledge.22, 25 However, US interpretation is highly subjective, and the US machine is a user-dependent tool.2 Acquiring and interpreting optimal US images is challenging and medical image interpretation is fallible.30-32 A further difficulty for teaching is the availability of appropriately skilled faculty.7, 33 As a result of all the above, students may obtain a varied learning experience and knowledge in US-based sessions. Accordingly, it is important to establish standardized educational objectives22 and identify methods which enable both the learner and teacher in these practices. The need for a consistent approach to US teaching has been recognized in recent years, with increasing global interest resulting in an international consensus group developing recommendations for an undergraduate US education.34 A sound understanding of the relevant anatomy is important to both skills, though available evidence does not always provide reassurance with respect to anesthesiologists' anatomical knowledge.38 Despite endeavors to promote anatomical knowledge amongst those training in the specialty,39 innovative educational approaches are needed when knowledge and performance are limited by the frailties discussed above. One such educational innovation in anatomy learning may be through the use of AI. AI is “the ability of a computer programme to perform processes associated with human intelligence”.40 It is a novel and rapidly evolving field that already surrounds us in everyday life - from guiding internet searches, and companies checking your credit rating, to self-driving cars. The terms machine learning and deep learning are often used interchangeably with AI: machine learning is a technique within the AI field, and deep learning is a subset of machine learning. Machine learning uses algorithms, which are rule-based problem-solving instructions implemented by a computer,12 to enable computers to perform specific tasks. The algorithm is exposed to training data, such as a bank of medical US images, and can improve task performance by making statistical correlations within the input training data and the desired output. In supervised machine learning, the algorithm is instructed what the desired output is (e.g., labeling sections of an US image as “nerve” or “muscle”). In unsupervised machine learning, the algorithm makes correlations autonomously by grouping regions of the data with common characteristics. Deep learning is particularly suited to image analysis.12 This approach uses artificial neurons, arranged in a network of layers, called a convolutional neural network.41, 42 Typically, each neuron is connected to the neurons of the layer below and above. There is an input layer, followed by multiple processing layers, and a final output layer. Neurons in each layer analyze the input data and draw out specific features—early layers identify simple features (e.g., straight lines), whilst deeper layers assess more complex features. From each layer of neurons, a map of the features is produced, resulting in the production of the overall analysis.41 By presenting the convolutional neural network with large volumes of input data, the network will learn to produce a fine-grained analysis of the original image.43 For example, the input data may be a basic US image, and the desired outcome could be correct labeling of a nerve. When trained, the algorithm can subsequently generate the desired outcome on new, previously unseen, input data. This domain of AI is often referred to as computer vision as it utilizes deep learning to allow computers to interpret the visual world. This is one example of a complex field. It is not yet clear when or how AI can be incorporated into all medical curricula, or even when the use of ultrasound will become routine, despite the increasing prevalence of the modality. However, it has been suggested that clinicians should be digitally literate and trained in the basics of AI.45-47 It is anticipated that AI will become entwined with future medical practice in a way that may change professional identities,45, 46, 48 and there are considerations around AI that should be understood. For example, there are fears that AI could influence medical care in becoming reductive, with AI not necessarily able to recognize the nuances of complex tasks.48, 50 AI can be something of a black box, so how a given system makes its prediction is unknown,51 and there should, therefore, be caution around any biases inherent within the system.52 Recent advances in AI technology have been noted within medical education, as it may support individualized learning with access to a large body of information.52 AI applications typically give rapid feedback,53 and would not suffer from fatigue or distraction.48 Proposed ideas include interactive training materials, such as a chat program,49 intelligent tutoring,53 and image bank algorithms.54 AI could become involved in student assessments to provide impartiality.51 Such innovative ideas have been welcomed in the context of distance learning,55 though are yet to be widely implemented within medical courses.47 Artificial intelligence, primarily in the form of deep learning, has been rapidly adopted in medical imaging. Examples include chest x-rays and optical coherence tomography.56, 57 Within US, assessment of the musculoskeletal system is common,58 such as assessing metacarpal cartilages59 and determining the diameter of the median nerve, with a future target of diagnosing carpal tunnel syndrome.60 Cardiology applications include assessing cardiac valves61 and detecting coronary artery abnormalities.62 One aim is to reduce repetitive tasks associated with analyzing echocardiograms.63 Obstetrics is also recognized as an area of potential application,40 with uses so far including obstetric measurements of the fetal head.64 Automated deep vein thrombosis assessment has also been developed.65 AI has been used in elucidating spinal anatomy where it can be challenging to accurately identify specific intervertebral spaces from surface landmarks, particularly when obesity complicates the surface anatomy.66, 67 This is of interest to anesthetists performing neuraxial anesthesia, as attempting a lumbar spinal injection superior to the cauda equina risks damage to the spinal cord.67 Ultrasound can assist with intervertebral space identification, and several AI algorithms have been developed to begin to address this area.68, 69 An example of applying these AI principles to ultrasound and clinical anatomy is to augment the identification of anatomical structures on US when learning or performing UGRA. ScanNav Anatomy PNB (v1.1, Intelligent Ultrasound, Cardiff, UK) is a device that can draw feed from an US display to apply an AI color overlay on the image in real-time (Figure 1 and Video File A). This is achieved through the use of a convolutional neural network, which has been trained using a supervised machine learning technique, on over 1,000,000 still-frame ultrasound images from nine PNB regions. Each training image has been presented to the convolutional neural network with a corresponding manually labeled color overlay. Through exposure to a high volume of training images, the network is now able to identify the structures of interest in the unmodified US presented in real time (Figure 2). Each US still frame is analyzed independently and every pixel in the frame is given a probability of belonging to a particular structure classification (e.g., nerve, artery, muscle, bone, pleura, peritoneum). The color of the structure class with the highest probability is then applied to each pixel (e.g., red for artery). The overall structure labels are then made up by the constellation of pixels with any given class. This color overlay refreshes at a rate of approximately 15 frames per second—only slightly slower than the US which streams still frames at 25–30 frames per second—providing near-continuous labeling of the US video. The aim of ScanNav Anatomy PNB is to aid in identifying structures of interest. Validation work has demonstrated the device to be accurate in identifying relevant structures in 93.5–99% of cases.31, 70 This is of particular use as nerves change their appearance along their course,71 which can be challenging for novice users to recognize. The device has been used in teaching to augment US image interpretation. It can support the acquisition of knowledge of the underlying anatomy and aid in the identification of structures on the US display. In a recent study,72 21 anesthetists underwent formal teaching to identify 20 key structures on ultrasound across six different PNBs. On later assessment, participants were randomized to perform a scan of three regions with AI assistance and three without. Those using the AI system were better able to correctly identify the anatomical structures on ultrasound (88.8% vs. 77.4%, p = 0.002). The device could help to overcome another common issue in UGRA teaching: articulating the expert's understanding of the US image to a learner. Understanding a greyscale US image, through intuitive to an expert, is not easy to a novice.27 Conveying one's interpretation and understanding can be hard, with the lack of readily described landmarks in a two-dimensional, dynamic greyscale image. Thus, an anatomically accurate overlay can provide color landmarks to reference during teaching and allow for independent study. The use of AI in teaching UGRA has been specifically evaluated.11 Thirty anesthesiologists, half experts, and half non-experts in UGRA, were asked to perform a series of ultrasound scans for specific PNB regions. Half of the scans were done with the aid of ScanNav Anatomy PNB, and half without. After each AI-assisted scan, users were asked to assess the utility of the device. In at least half of the cases, experts found it to be useful in teaching (30/60 scans, 50.0%), whilst at least half of the non-experts found it to be a useful aid to structure identification (31/60, 51.7%), learning the scanning technique (36/60; 60.0%), their training (37/60; 61.7%) and to improve their confidence in scanning (31/60; 51.7%). Negative responses to these questions were infrequent (0–4/60; 0%–6.7%), with the rest neutral, though the threshold of positive response rate for acceptance is not firmly established. Use in the clinical setting expands the role of such technology, from the pre-clinical university environment to the postgraduate clinical setting where medical professionals can augment their training. Translating anatomical knowledge into practical skills is challenging,73 and innovation is needed to deliver the benefits to training and medical practice. Other similar AI systems include Nerveblox (SmartAlpha Teknologi San. Ve. Tic. AS., Ankara, Turkey), which applies a color overlay to real-time ultrasound.74, 75 NerveTrack™ (Samsung Medison, Seoul, South Korea) applies a bounding box around the median and ulnar nerves in the forearm.76 These systems appear to be earlier in their lifecycle than ScanNav Anatomy PNB and there are fewer published data on their performance or utility, particularly as educational devices. Despite enthusiasm among students and apparent educational efficacy, there can be challenges to adopting US in anatomical education in addition to identifying sufficient faculty. Some non-clinicians view US as too difficult a skill to acquire.7 This may be partly due to challenges operating US machines, as this can initially provide a high cognitive load77 or due to concerns over image quality. However, US technology has progressed quickly, and traditional views on this may rapidly become outdated.7 Nevertheless, US machines can be expensive, with cart-based machines commonly costing over $50,000, although handheld devices can be less than $10,000. Stand-alone devices such as ScanNav Anatomy PNB add further expense, typically costing slightly more than a handheld device. AI devices add significant expense to already stretched departmental budgets—a challenge that is unlikely to diminish until such technology becomes widespread. Artificial intelligence devices may reduce perceived barriers by making US more accessible. The systems may reduce the dependence on the availability of an appropriate supervisor. Use of feedback provided by the AI (e.g., color identification of relevant structures) could help to facilitate independent learning and so liberate learners from dependence upon experts. The AI device could be an additional resource to allow revision of previously taught sessions, permitting additional practice when supervisors may not be readily available. An appropriate initial introduction to a topic and orientation to the AI device should be given. Conversely, the color overlay could be displayed to a remote supervisor, who could then use that image to provide guidance. However, after using an AI device to assist with independent learning, currently, a student's progress should still be assessed using standard assessment processes. Whilst there is enthusiasm for AI, it has not yet been validated as a tool for skill acquisition, with further work needed to establish the role for AI. Training AI models can be time-consuming63, 44 and human error may affect training data acquisition or ground truth labeling. AI systems can also have difficulty identifying aberrant anatomy, leading to poorer performance.60 Anatomical variants are recognized within UGRA30 and can influence PNB success. This is an important issue which should be acknowledged as a potential challenge. Anatomical knowledge underpins medical practice, although it is challenging to acquire this basic science due to the learning pressures applied by an expanding body of medical knowledge. Interest in using ultrasound to augment the teaching of this fundamental knowledge base is rapidly increasing, although barriers remain. The use of innovative AI technology alongside ultrasound offers the potential to supplement learning, with many potential applications. Here, we have provided one example, of ultrasound-guided regional anesthesia, where radiological and anatomical learning is integrated with the development of a clinical skill. Structured goals may be met by incorporating AI into US education and practice, with numerous future clinical applications. The senior author (J.S.B.) is a Senior Clinical Advisor for Intelligent Ultrasound Limited (Cardiff, U.K.), receiving research funding and honoraria. Other authors report no conflict of interest. Emma Jacobs, B.Med.Sc. (Hons.), M.B.Ch.B., P.G.Cert., is an Anesthesia Core Trainee in the Department of Anesthesia, Royal Gwent Hospital at the Aneurin Bevan University Health Board in Newport, United Kingdom. She has an interest in medical education. Bruce Wainman, Ph.D., F.A.A.A., is a professor of Pathology and Molecular Medicine, and the Director of the Education Program in Anatomy and the Surgical Skills Laboratory at McMaster University, Hamilton, Ontario, Canada. He teaches anatomy, physiology, and pharmacology to various programs at the undergraduate and graduate levels. His academic interests are in the role of artificial intelligence and extended reality in anatomy education, interprofessional education, and the ethics of body donation. James Bowness, B.Sc. (Hons.), M.B.B.Chir. (Cantab.), M.R.C.S., F.R.C.A., is a consultant anesthetist in the Department of Anesthesia, Royal Gwent Hospital, at the Aneurin Bevan University Health Board in Newport, United Kingdom. He is also a graduate (Ph.D.) student in the OxSTaR Center, Nuffield Division of Anesthetics, Nuffield Department of Clinical Neurosciences, University of Oxford, Oxford, United Kingdom. He has taught anatomy at the University of St. Andrews and the Royal College of Surgeons (Edinburgh), and his research interests include anatomy, ultrasound, regional anesthesia, and artificial intelligence. Video S1 Video S2 Data S1 Please note: The publisher is not responsible for the content or functionality of any supporting information supplied by the authors. Any queries (other than missing content) should be directed to the corresponding author for the article.
更多查看译文
关键词
ultrasound,anesthesia,artificial intelligence,educational tool
AI 理解论文
溯源树
样例
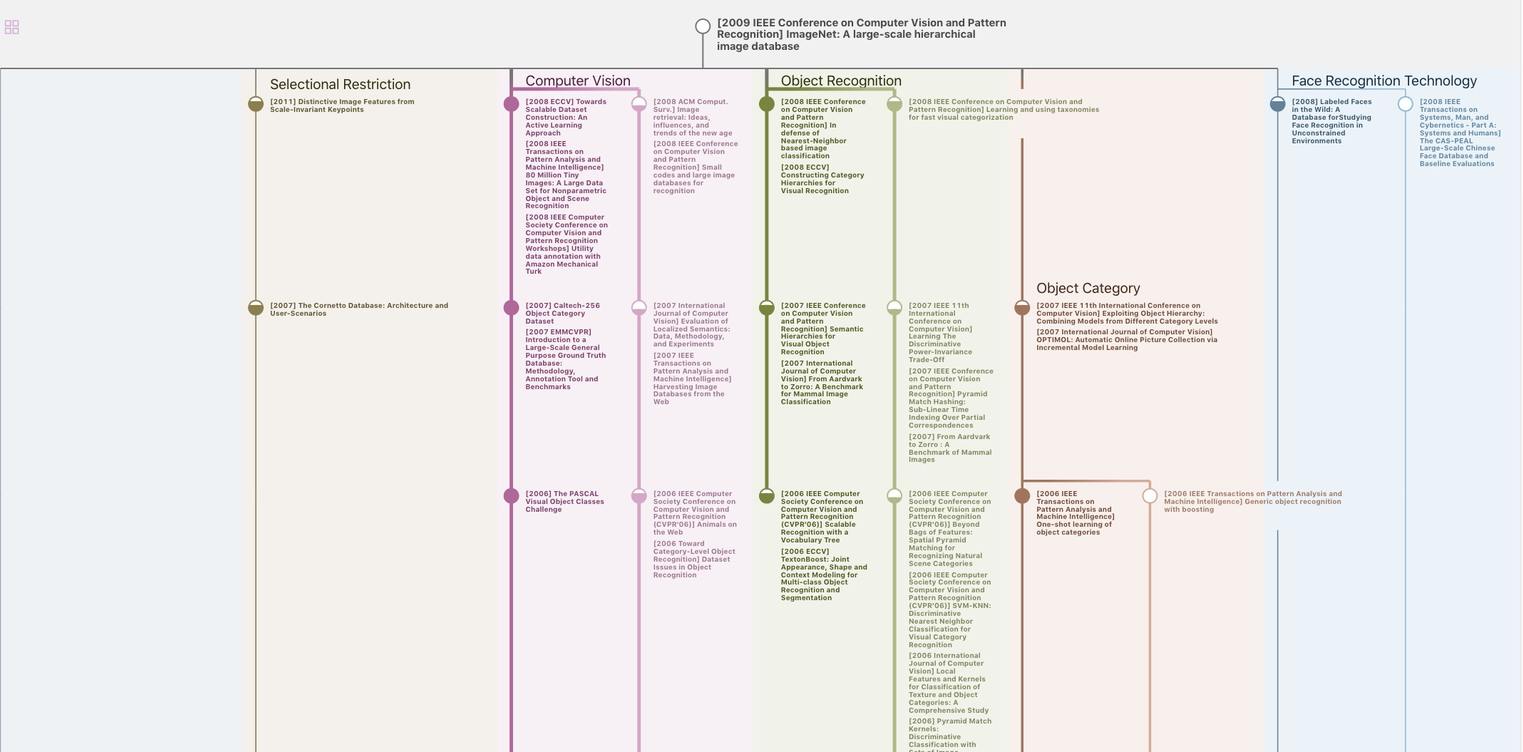
生成溯源树,研究论文发展脉络
Chat Paper
正在生成论文摘要