Group conditional validity via multi-group learning
arxiv(2023)
摘要
We consider the problem of distribution-free conformal prediction and the criterion of group conditional validity. This criterion is motivated by many practical scenarios including hidden stratification and group fairness. Existing methods achieve such guarantees under either restrictive grouping structure or distributional assumptions, or they are overly-conservative under heteroskedastic noise. We propose a simple reduction to the problem of achieving validity guarantees for individual populations by leveraging algorithms for a problem called multi-group learning. This allows us to port theoretical guarantees from multi-group learning to obtain obtain sample complexity guarantees for conformal prediction. We also provide a new algorithm for multi-group learning for groups with hierarchical structure. Using this algorithm in our reduction leads to improved sample complexity guarantees with a simpler predictor structure.
更多查看译文
关键词
conditional validity,learning,multi-group
AI 理解论文
溯源树
样例
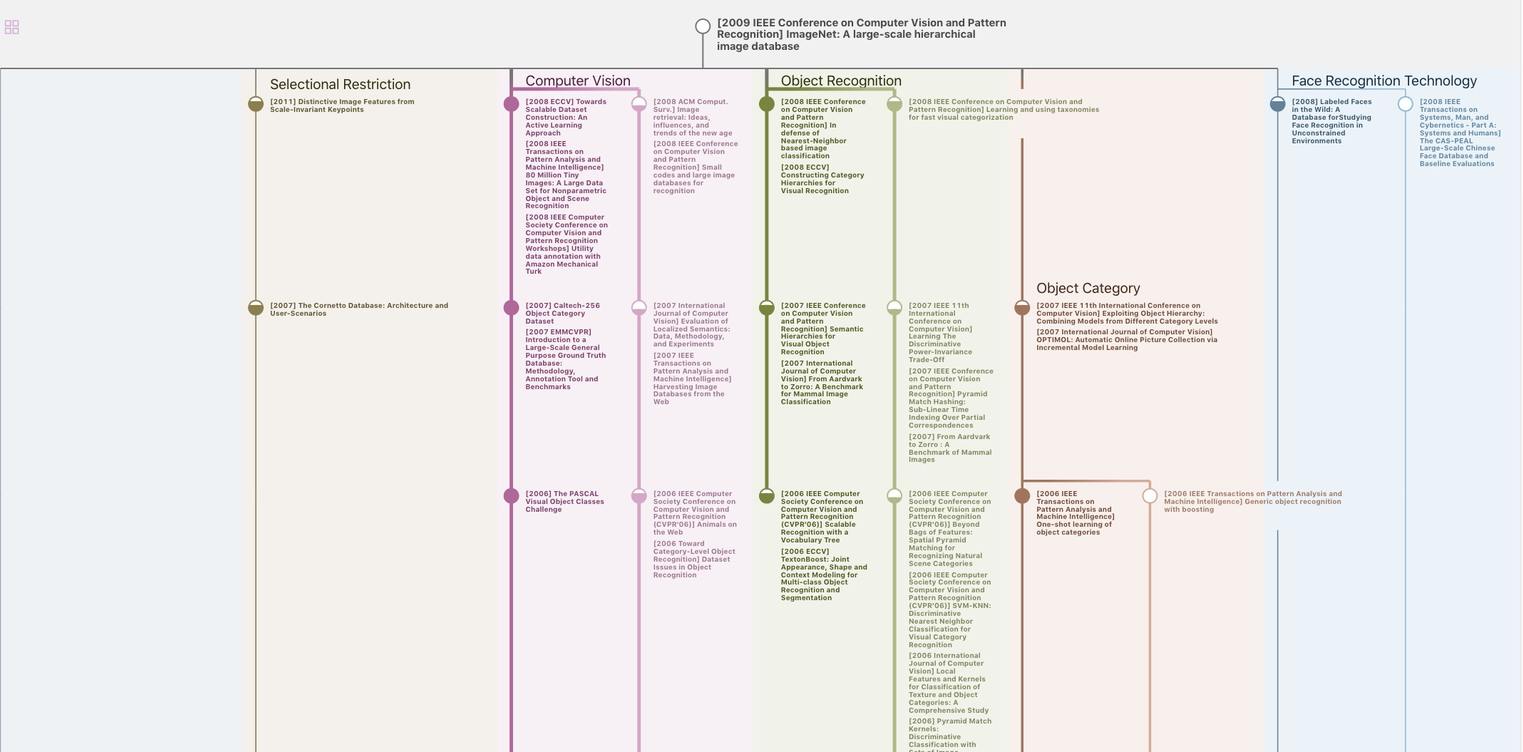
生成溯源树,研究论文发展脉络
Chat Paper
正在生成论文摘要