Fast and Expandable ANN-Based Compact Model and Parameter Extraction for Emerging Transistors
IEEE Journal of the Electron Devices Society(2023)
摘要
In this paper, we present a fast and expandable artificial neural network (ANN)-based compact model and parameter extraction flow to replace the existing complicated compact model implementation and model parameter extraction (MPE) method. In addition to nanosheet FETs (NSFETs), our published ANN-based compact modeling framework is easily extended to negative capacitance NSFETs (NC-NSFETs), which are attracting attention as next-generation devices. Each device is designed using a technology computer-aided design (TCAD) simulator. Using device structure parameters, temperature, and channel doping depth as input variables, we construct a dataset of electrical properties used for machine learning (ML)-based modeling. The accuracy of predicting device electrical characteristics with the proposed ANN-based compact model is less than a 1% error compared to TCAD, and simulation results of digital and analog circuits using the proposed compact model show less than a 3% error. This allows the ANN-based modeling framework to achieve accurate DC, AC, and transient simulations without restrictions on device technology. In particular, temperature and process variables such as channel doping depth, which are not defined in the compact model parameters, are easily added to the previously presented five key parameters. Instead of conventional complex compact modeling and MPE work, we propose a method to create fast, accurate, flexible, and expandable ML-based Verilog-A SPICE models with design technology co-optimization (DTCO) capabilities.
更多查看译文
关键词
Artificial neural network (ANN),machine learning (ML),compact model,modelparameter extraction (MPE),nanosheet FET (NSFET),negative capacitance NSFET (NC-NSFET),SPICE
AI 理解论文
溯源树
样例
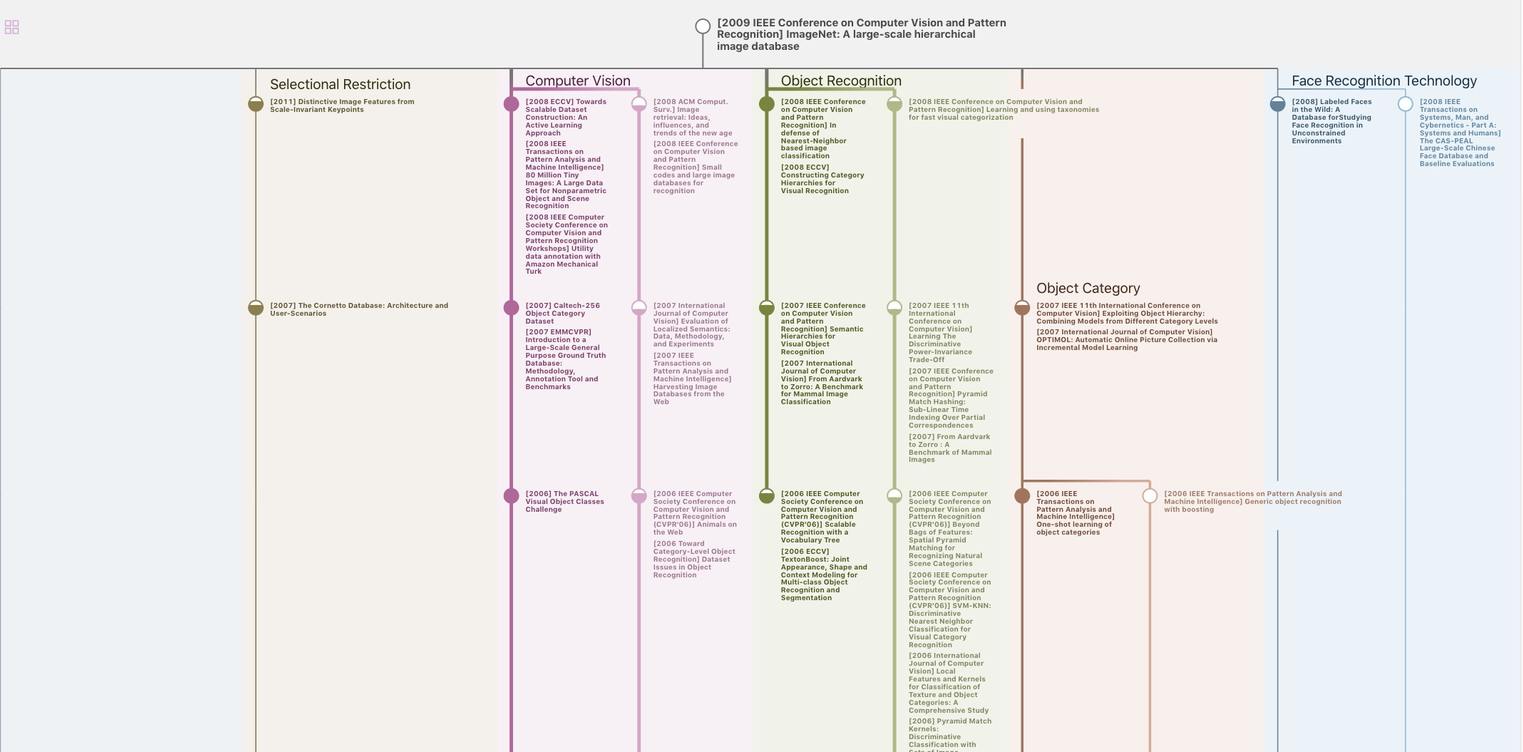
生成溯源树,研究论文发展脉络
Chat Paper
正在生成论文摘要