Learning to Discriminate Adversarial Examples by Sensitivity Inconsistency in IoHT Systems.
Journal of healthcare engineering(2023)
摘要
Deep neural networks (DNNs) have been widely adopted in many fields, and they greatly promote the Internet of Health Things (IoHT) systems by mining health-related information. However, recent studies have shown the serious threat to DNN-based systems posed by adversarial attacks, which has raised widespread concerns. Attackers maliciously craft adversarial examples (AEs) and blend them into the normal examples (NEs) to fool the DNN models, which seriously affects the analysis results of the IoHT systems. Text data is a common form in such systems, such as the patients' medical records and prescriptions, and we study the security concerns of the DNNs for textural analysis. As identifying and correcting AEs in discrete textual representations is extremely challenging, the available detection techniques are still limited in performance and generalizability, especially in IoHT systems. In this paper, we propose an efficient and structure-free adversarial detection method, which detects AEs even in attack-unknown and model-agnostic circumstances. We reveal that sensitivity inconsistency prevails between AEs and NEs, leading them to react differently when important words in the text are perturbed. This discovery motivates us to design an adversarial detector based on adversarial features, which are extracted based on sensitivity inconsistency. Since the proposed detector is structure-free, it can be directly deployed in off-the-shelf applications without modifying the target models. Compared to the state-of-the-art detection methods, our proposed method improves adversarial detection performance, with an adversarial recall of up to 99.7% and an 1-score of up to 97.8%. In addition, extensive experiments have shown that our method achieves superior generalizability as it can be generalized across different attackers, models, and tasks.
更多查看译文
关键词
discriminate adversarial examples,sensitivity inconsistency,learning
AI 理解论文
溯源树
样例
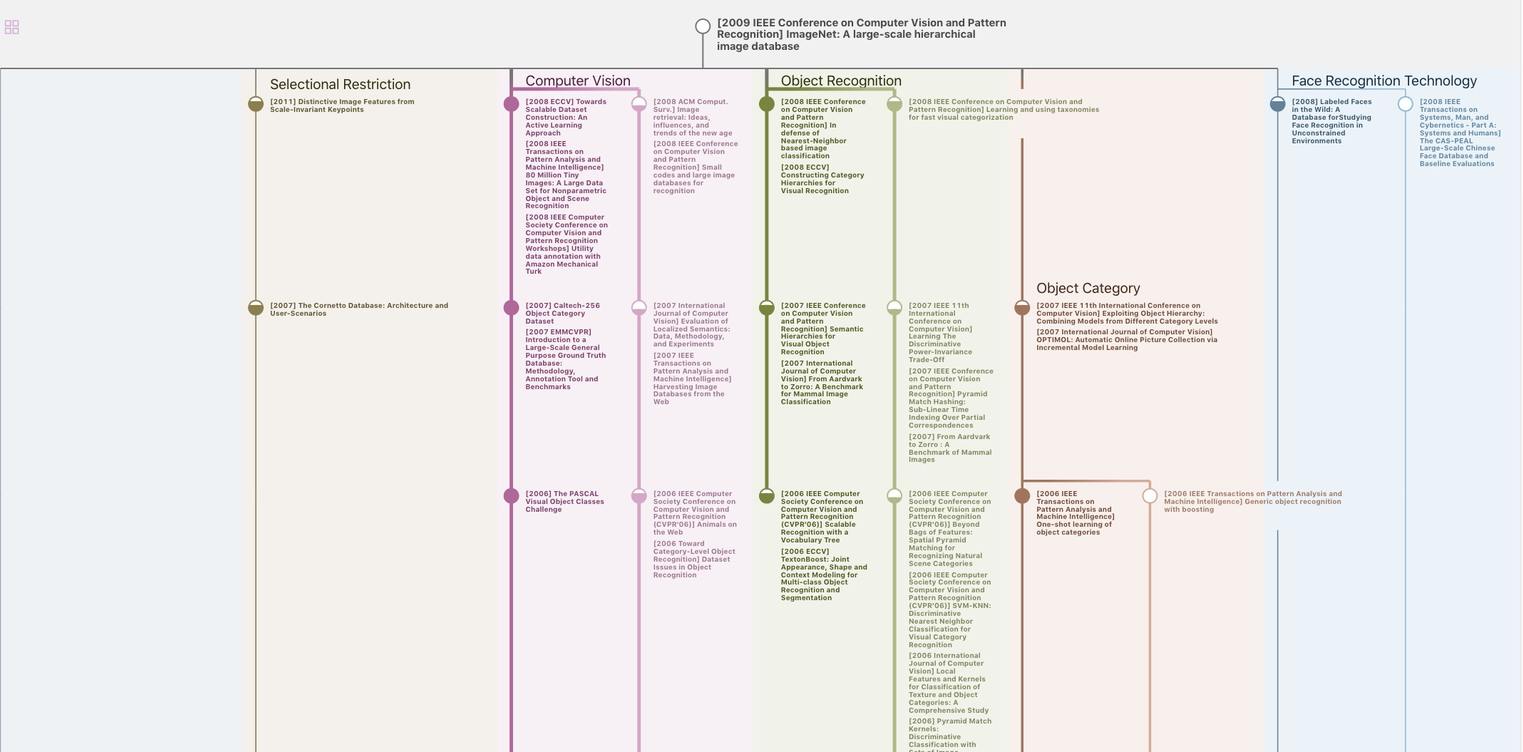
生成溯源树,研究论文发展脉络
Chat Paper
正在生成论文摘要