Deep learning-enabled volumetric cone photoreceptor segmentation in adaptive optics optical coherence tomography images of normal and diseased eyes.
Biomedical optics express(2023)
摘要
Objective quantification of photoreceptor cell morphology, such as cell diameter and outer segment length, is crucial for early, accurate, and sensitive diagnosis and prognosis of retinal neurodegenerative diseases. Adaptive optics optical coherence tomography (AO-OCT) provides three-dimensional (3-D) visualization of photoreceptor cells in the living human eye. The current gold standard for extracting cell morphology from AO-OCT images involves the tedious process of 2-D manual marking. To automate this process and extend to 3-D analysis of the volumetric data, we propose a comprehensive deep learning framework to segment individual cone cells in AO-OCT scans. Our automated method achieved human-level performance in assessing cone photoreceptors of healthy and diseased participants captured with three different AO-OCT systems representing two different types of point scanning OCT: spectral domain and swept source.
更多查看译文
关键词
volumetric cone photoreceptor segmentation,optical coherence tomography images,optical coherence tomography,adaptive optics,diseased eyes,learning-enabled
AI 理解论文
溯源树
样例
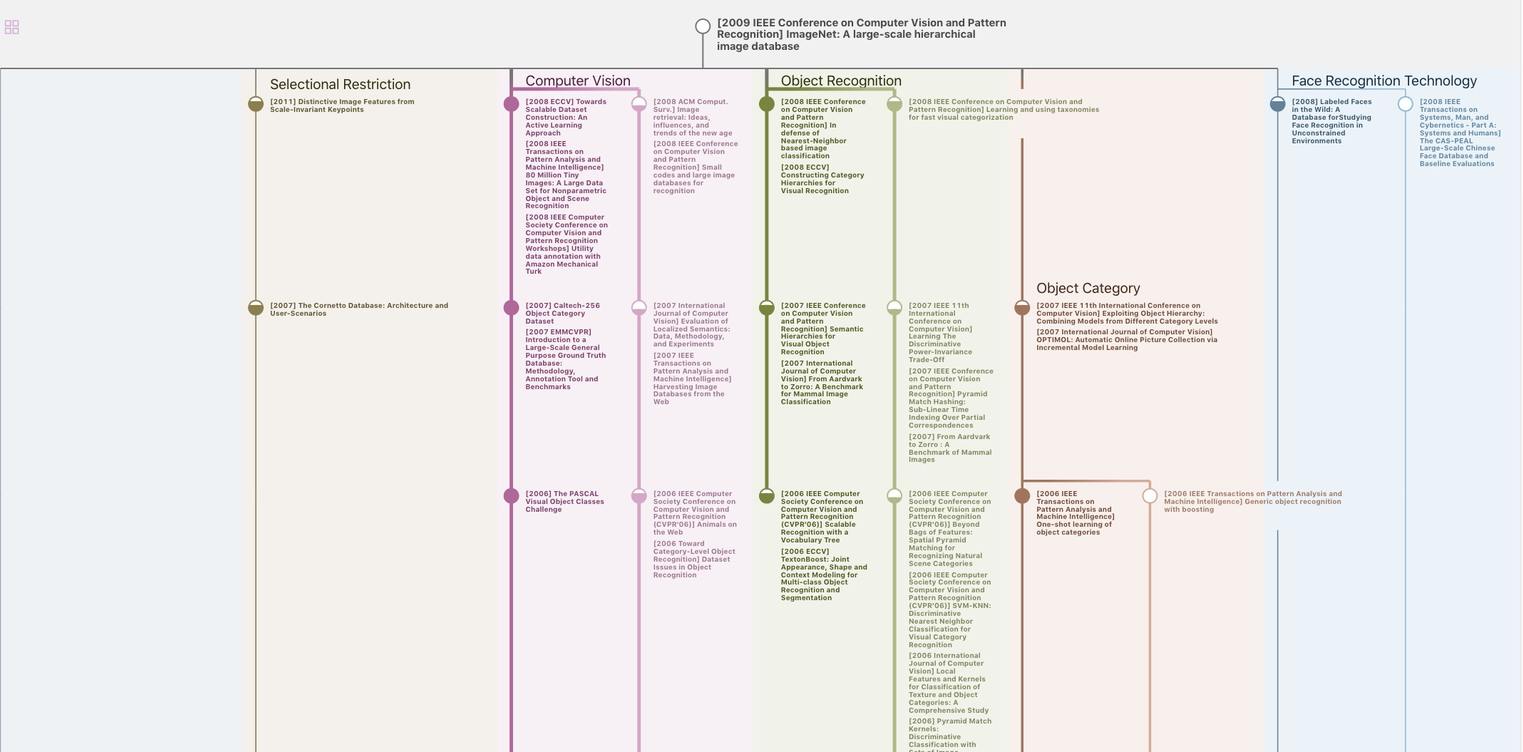
生成溯源树,研究论文发展脉络
Chat Paper
正在生成论文摘要