Scenario-Agnostic Zero-Trust Defense with Explainable Threshold Policy: A Meta-Learning Approach
arxiv(2023)
摘要
The increasing connectivity and intricate remote access environment have made traditional perimeter-based network defense vulnerable. Zero trust becomes a promising approach to provide defense policies based on agent-centric trust evaluation. However, the limited observations of the agent's trace bring information asymmetry in the decision-making. To facilitate the human understanding of the policy and the technology adoption, one needs to create a zero-trust defense that is explainable to humans and adaptable to different attack scenarios. To this end, we propose a scenario-agnostic zero-trust defense based on Partially Observable Markov Decision Processes (POMDP) and first-order Meta-Learning using only a handful of sample scenarios. The framework leads to an explainable and generalizable trust-threshold defense policy. To address the distribution shift between empirical security datasets and reality, we extend the model to a robust zero-trust defense minimizing the worst-case loss. We use case studies and real-world attacks to corroborate the results.
更多查看译文
关键词
Zero-trust security,meta learning,scenario-agnostic,threshold policy
AI 理解论文
溯源树
样例
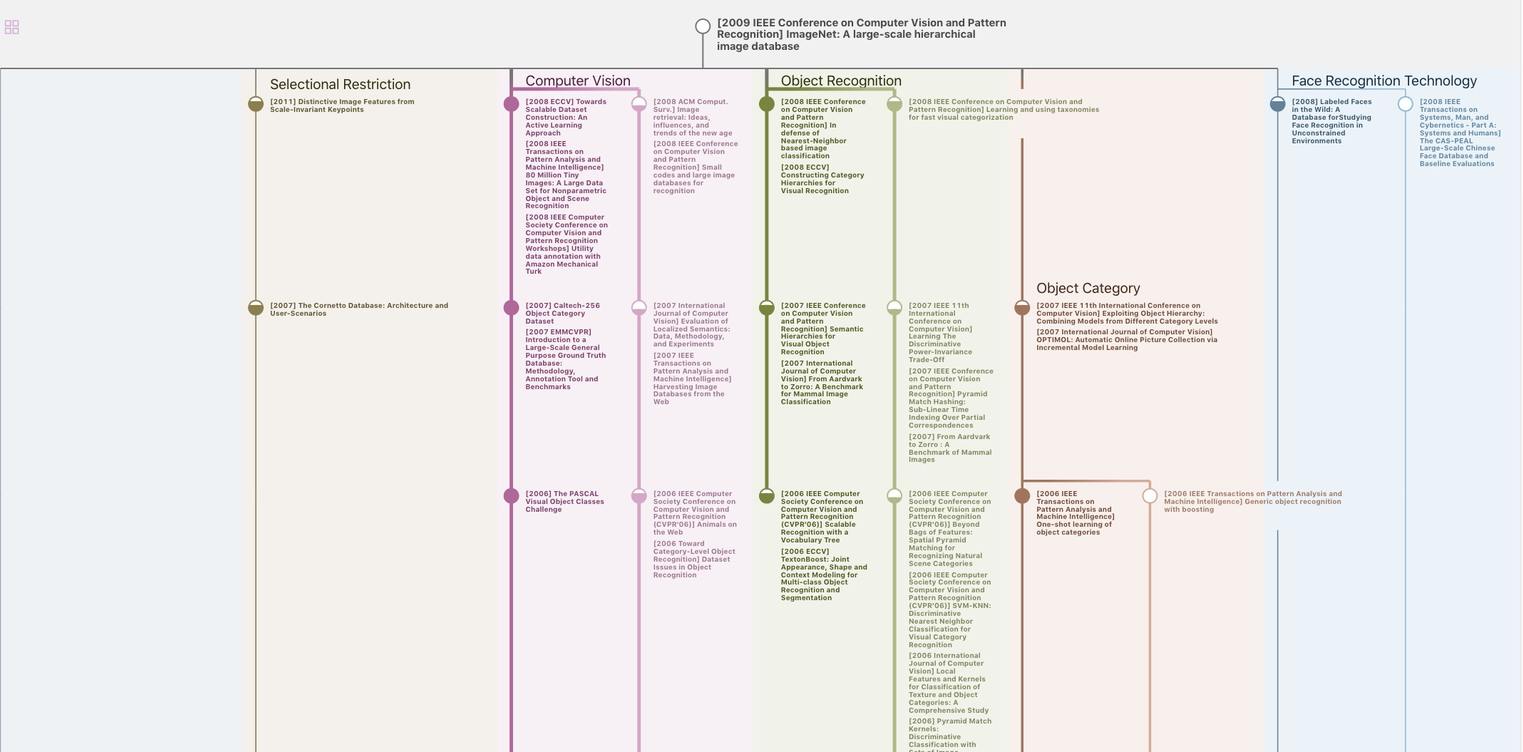
生成溯源树,研究论文发展脉络
Chat Paper
正在生成论文摘要