Parallel Hybrid Networks: an interplay between quantum and classical neural networks
arxiv(2023)
摘要
Quantum neural networks represent a new machine learning paradigm that has recently attracted much attention due to its potential promise. Under certain conditions, these models approximate the distribution of their dataset with a truncated Fourier series. The trigonometric nature of this fit could result in angle-embedded quantum neural networks struggling to fit the non-harmonic features in a given dataset. Moreover, the interpretability of neural networks remains a challenge. In this work, we introduce a new, interpretable class of hybrid quantum neural networks that pass the inputs of the dataset in parallel to 1) a classical multi-layered perceptron and 2) a variational quantum circuit, and then the outputs of the two are linearly combined. We observe that the quantum neural network creates a smooth sinusoidal foundation base on the training set, and then the classical perceptrons fill the non-harmonic gaps in the landscape. We demonstrate this claim on two synthetic datasets sampled from periodic distributions with added protrusions as noise. The training results indicate that the parallel hybrid network architecture could improve the solution optimality on periodic datasets with additional noise.
更多查看译文
关键词
quantum,neural networks,hybrid,parallel
AI 理解论文
溯源树
样例
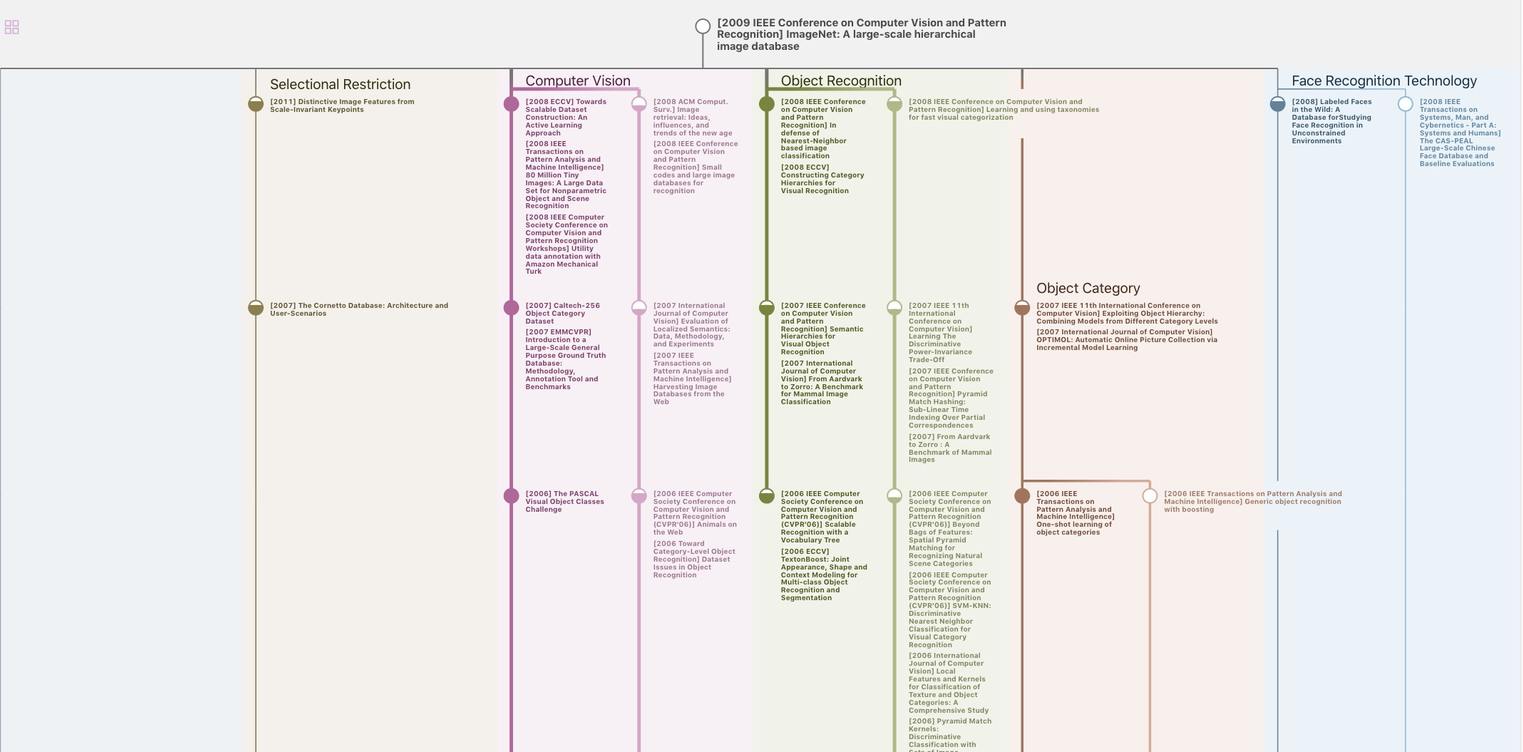
生成溯源树,研究论文发展脉络
Chat Paper
正在生成论文摘要