ST-KeyS: Self-Supervised Transformer for Keyword Spotting in Historical Handwritten Documents
arxiv(2023)
摘要
Keyword spotting (KWS) in historical documents is an important tool for the initial exploration of digitized collections. Nowadays, the most efficient KWS methods are relying on machine learning techniques that require a large amount of annotated training data. However, in the case of historical manuscripts, there is a lack of annotated corpus for training. To handle the data scarcity issue, we investigate the merits of the self-supervised learning to extract useful representations of the input data without relying on human annotations and then using these representations in the downstream task. We propose ST-KeyS, a masked auto-encoder model based on vision transformers where the pretraining stage is based on the mask-and-predict paradigm, without the need of labeled data. In the fine-tuning stage, the pre-trained encoder is integrated into a siamese neural network model that is fine-tuned to improve feature embedding from the input images. We further improve the image representation using pyramidal histogram of characters (PHOC) embedding to create and exploit an intermediate representation of images based on text attributes. In an exhaustive experimental evaluation on three widely used benchmark datasets (Botany, Alvermann Konzilsprotokolle and George Washington), the proposed approach outperforms state-of-the-art methods trained on the same datasets.
更多查看译文
关键词
keyword spotting,documents,st-keys,self-supervised
AI 理解论文
溯源树
样例
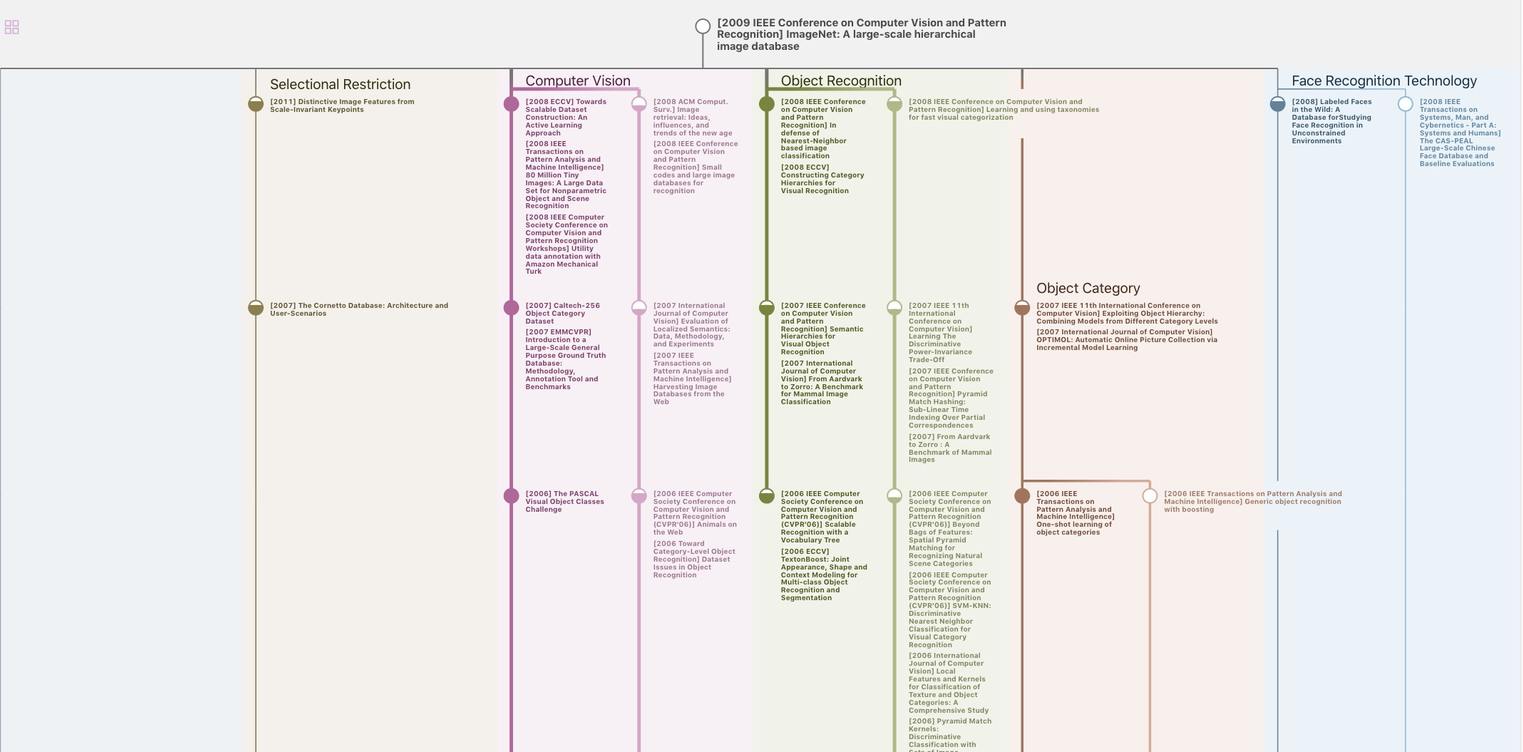
生成溯源树,研究论文发展脉络
Chat Paper
正在生成论文摘要