Towards Zero-Shot Functional Compositionality of Language Models
arxiv(2023)
摘要
Large Pre-trained Language Models (PLM) have become the most desirable starting point in the field of NLP, as they have become remarkably good at solving many individual tasks. Despite such success, in this paper, we argue that current paradigms of working with PLMs are neglecting a critical aspect of modeling human intelligence: functional compositionality. Functional compositionality - the ability to compose learned tasks - has been a long-standing challenge in the field of AI (and many other fields) as it is considered one of the hallmarks of human intelligence. An illustrative example of such is cross-lingual summarization, where a bilingual person (English-French) could directly summarize an English document into French sentences without having to translate the English document or summary into French explicitly. We discuss why this matter is an important open problem that requires further attention from the field. Then, we show that current PLMs (e.g., GPT-2 and T5) don't have functional compositionality yet and it is far from human-level generalizability. Finally, we suggest several research directions that could push the field towards zero-shot functional compositionality of language models.
更多查看译文
关键词
functional,language,models,zero-shot
AI 理解论文
溯源树
样例
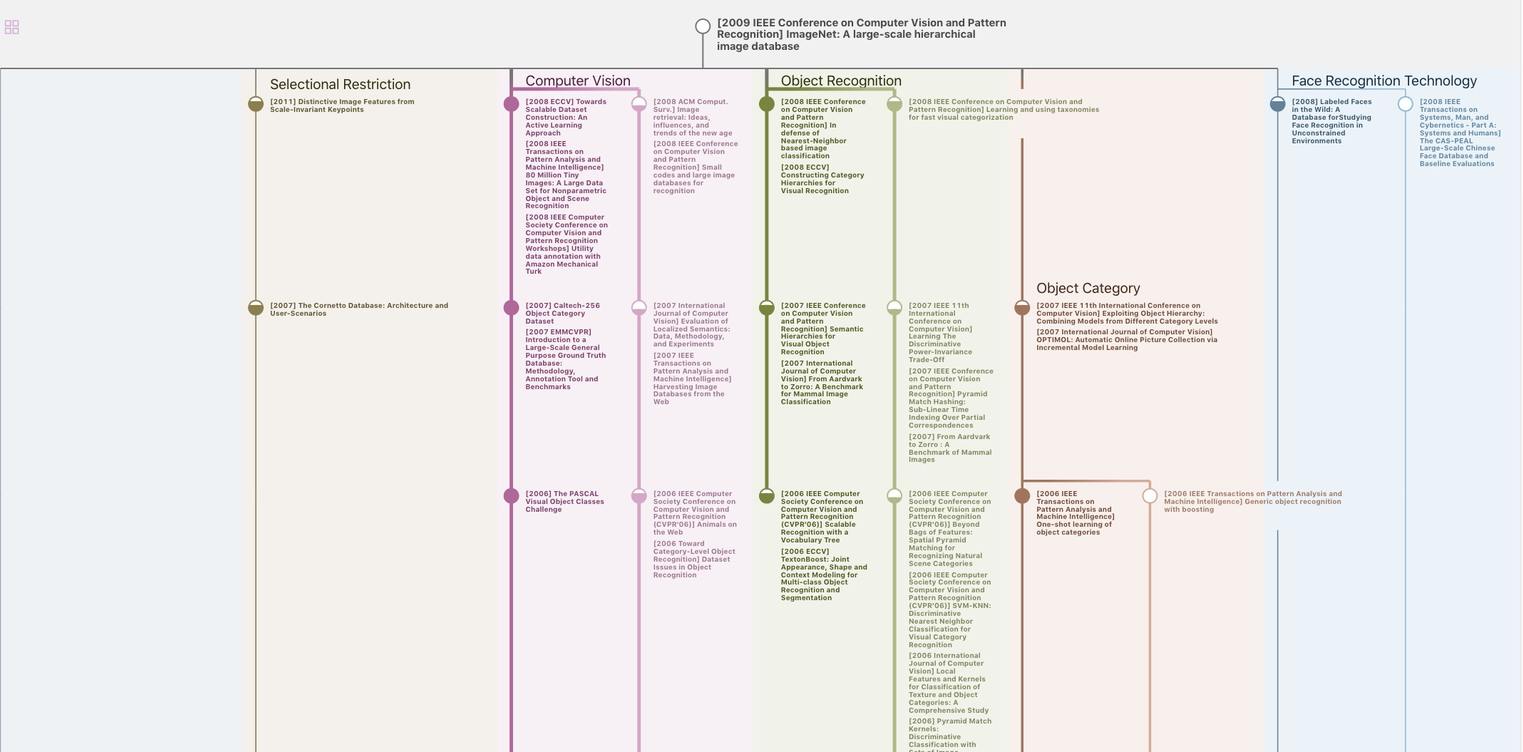
生成溯源树,研究论文发展脉络
Chat Paper
正在生成论文摘要