Achieving Counterfactual Fairness for Anomaly Detection
arxiv(2023)
摘要
Ensuring fairness in anomaly detection models has received much attention recently as many anomaly detection applications involve human beings. However, existing fair anomaly detection approaches mainly focus on association-based fairness notions. In this work, we target counterfactual fairness, which is a prevalent causation-based fairness notion. The goal of counterfactually fair anomaly detection is to ensure that the detection outcome of an individual in the factual world is the same as that in the counterfactual world where the individual had belonged to a different group. To this end, we propose a counterfactually fair anomaly detection (CFAD) framework which consists of two phases, counterfactual data generation and fair anomaly detection. Experimental results on a synthetic dataset and two real datasets show that CFAD can effectively detect anomalies as well as ensure counterfactual fairness.
更多查看译文
关键词
counterfactual fairness,detection
AI 理解论文
溯源树
样例
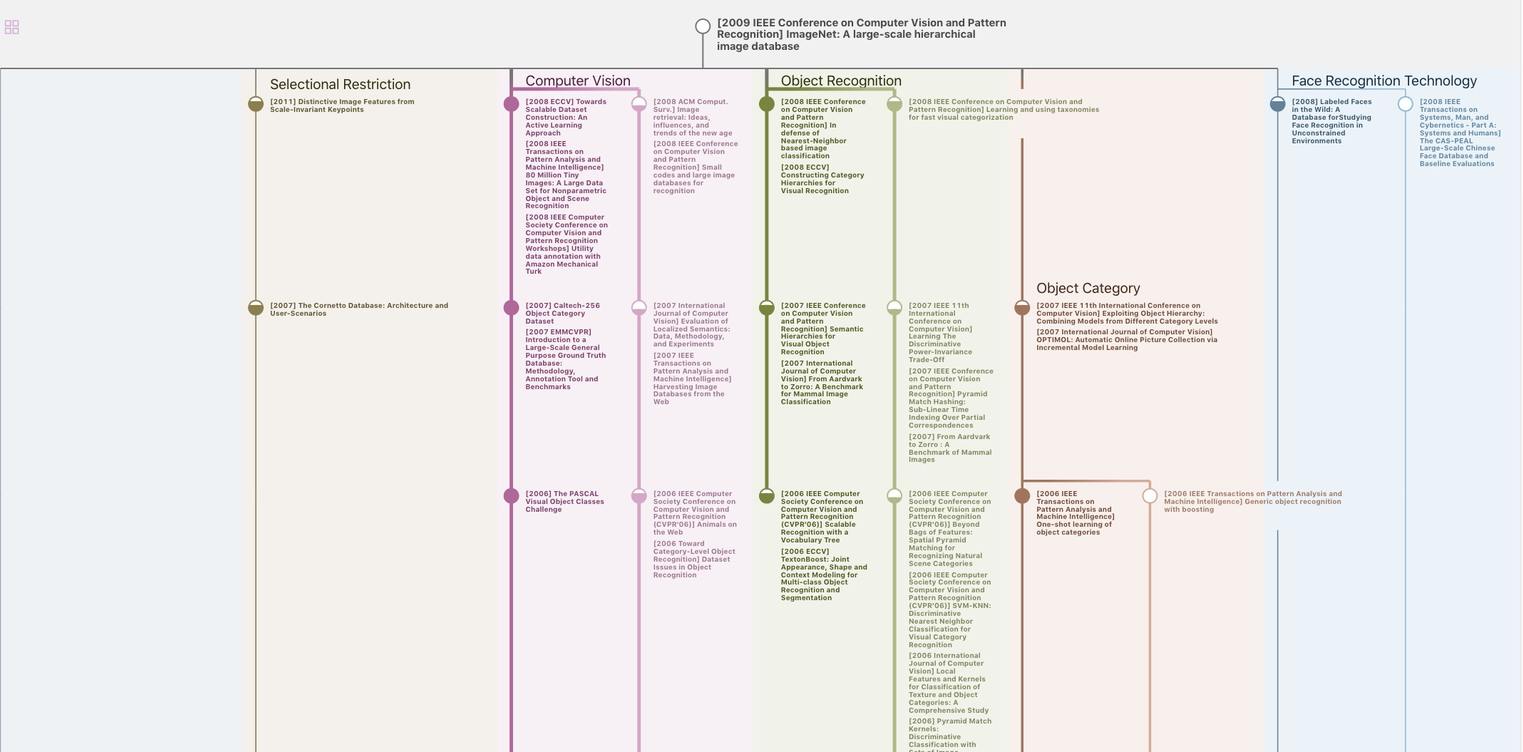
生成溯源树,研究论文发展脉络
Chat Paper
正在生成论文摘要