Low-Complexity Audio Embedding Extractors
arxiv(2023)
摘要
Solving tasks such as speaker recognition, music classification, or semantic audio event tagging with deep learning models typically requires computationally demanding networks. General-purpose audio embeddings (GPAEs) are dense representations of audio signals that allow lightweight, shallow classifiers to tackle various audio tasks. The idea is that a single complex feature extractor would extract dense GPAEs, while shallow MLPs can produce task-specific predictions. If the extracted dense representations are general enough to allow the simple downstream classifiers to generalize to a variety of tasks in the audio domain, a single costly forward pass suffices to solve multiple tasks in parallel. In this work, we try to reduce the cost of GPAE extractors to make them suitable for resource-constrained devices. We use efficient MobileNets trained on AudioSet using Knowledge Distillation from a Transformer ensemble as efficient GPAE extractors. We explore how to obtain high-quality GPAEs from the model, study how model complexity relates to the quality of extracted GPAEs, and conclude that low-complexity models can generate competitive GPAEs, paving the way for analyzing audio streams on edge devices w.r.t. multiple audio classification and recognition tasks.
更多查看译文
关键词
low-complexity
AI 理解论文
溯源树
样例
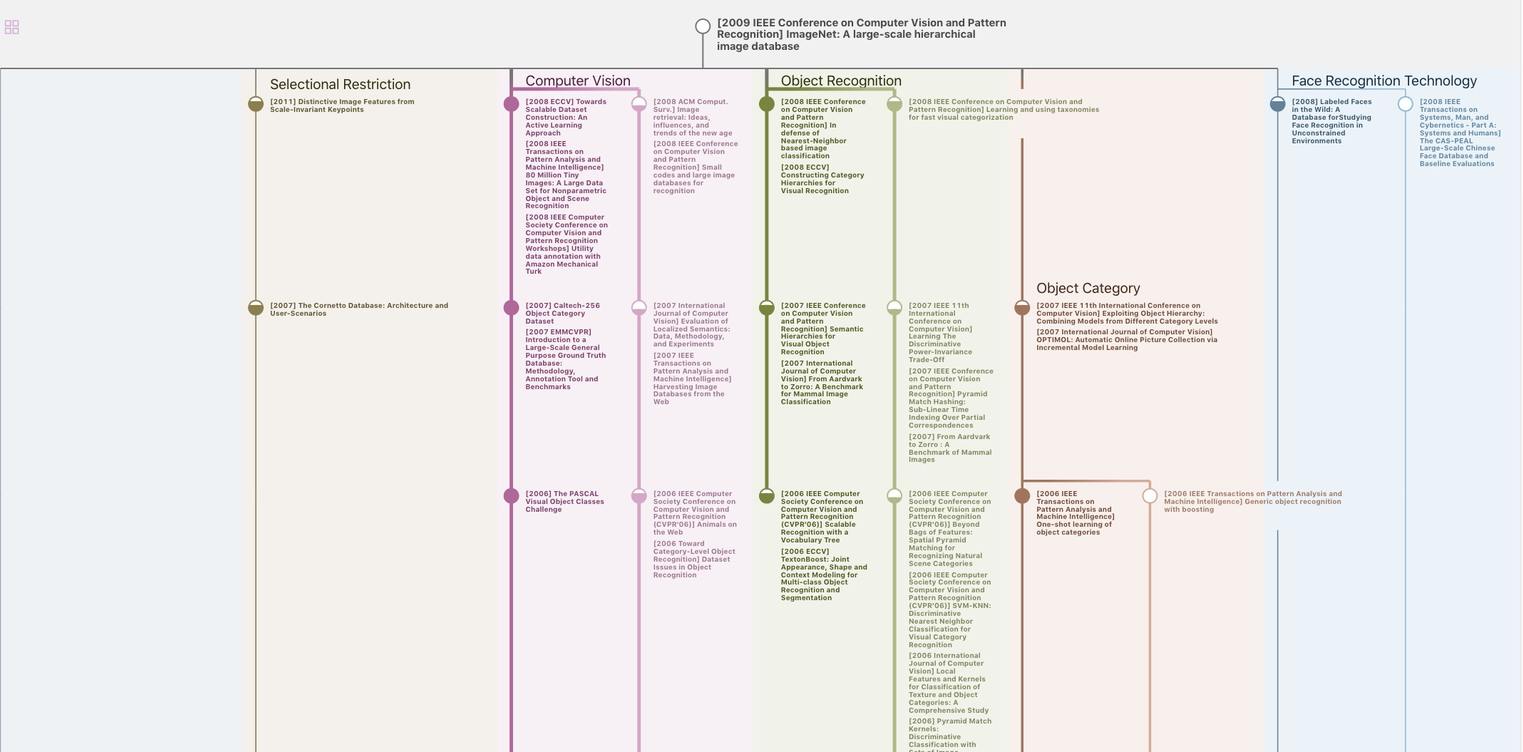
生成溯源树,研究论文发展脉络
Chat Paper
正在生成论文摘要