Near Optimal Memory-Regret Tradeoff for Online Learning
2023 IEEE 64TH ANNUAL SYMPOSIUM ON FOUNDATIONS OF COMPUTER SCIENCE, FOCS(2023)
摘要
In the experts problem, on each of T days, an agent needs to follow the advice of one of n "experts". After each day, the loss associated with each expert's advice is revealed. A fundamental result in learning theory says that the agent can achieve vanishing regret, i.e. their cumulative loss is within o(T) of the cumulative loss of the best-in-hindsight expert. Can the agent perform well without sufficient space to remember all the experts? We extend a nascent line of research on this question in two directions: 1) We give a new algorithm against the oblivious adversary, improving over the memory-regret tradeoff obtained by [PZ23], and nearly matching the lower bound of [SWXZ22]. 2) We also consider an adaptive adversary who can observe past experts chosen by the agent. In this setting we give both a new algorithm and a novel lower bound, proving that roughly root n memory is both necessary and sufficient for obtaining o(T) regret.
更多查看译文
关键词
Online learning
AI 理解论文
溯源树
样例
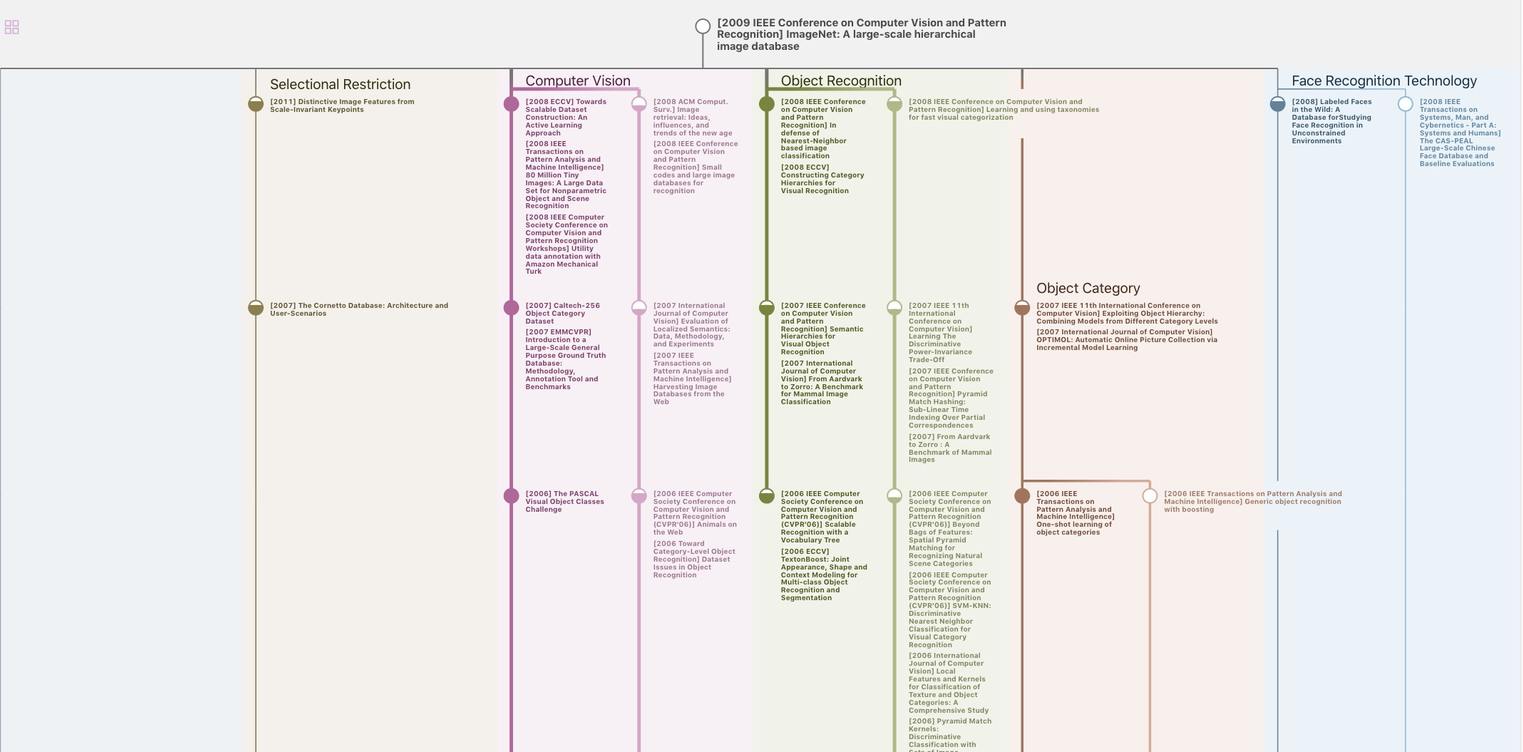
生成溯源树,研究论文发展脉络
Chat Paper
正在生成论文摘要