WiSE: W hen Learning Assi sts Resolving S TT-MRAM E fficiency Challenges
IEEE Transactions on Emerging Topics in Computing(2023)
摘要
Spin Transfer Torque Magnetic RAM (STT-MRAM) is one of the most promising on-chip technologies, which delivers high density, non-volatility, and near-zero leakage power. However, Spin Transfer Torque Magnetic RAM (STT-MRAM) suffers from three reliability issues, namely, read disturbance, write failure, and retention failure, that present significant challenges to its use as a reliable on-chip memory. All of these three reliability challenges become even more threatening with any increase in STT-MRAM cell temperature. Write operations are regarded as the main source of heat generation and temperature increase in STT-MRAM on-chip memories. This article first presents experiments to show how the heat generated by consecutive writes affects the reliability of an STT-MRAM on-chip cache. Then, it proposes the WiSE framework, an approach to reduce the STT-MRAM-based cache memory temperature and improve its reliability. WiSE utilizes the Reinforcement Learning (Reinforcement Learning (RL)) technique to detect high-density write operation patterns in STT-MRAM cache. To manage the write operations across the STT-MRAM caches, WiSE introduces a new temperature-aware replacement policy. The simulation results show that while WiSE imposes only about 1% performance overhead, it improves retention failure rate, read disturbance rate and write failure rate by 64%, 57%, and 47%, respectively, compared to Least Recently Used (Least Recently Used (LRU)) replacement policy.
更多查看译文
关键词
Reinforcement learning,reliability,replacement policy,STT-MRAM,heat accumulation
AI 理解论文
溯源树
样例
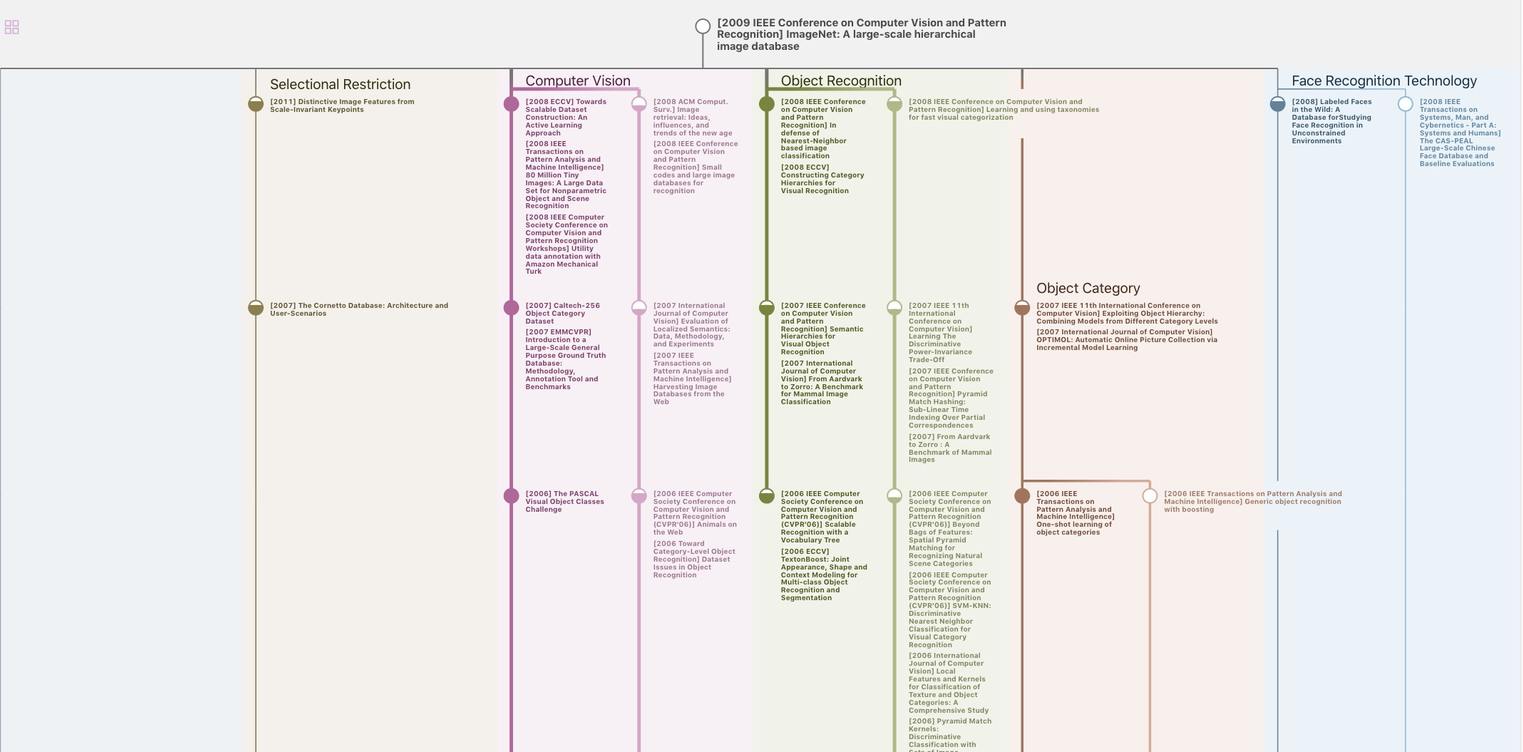
生成溯源树,研究论文发展脉络
Chat Paper
正在生成论文摘要