One-Bit Source Number Detection via Neural Network
2022 5th International Conference on Information Communication and Signal Processing (ICICSP)(2022)
摘要
Accurate source number detection with one-bit quantized data is of considerable interest in array signal processing, as one-bit quantization offers low cost and low complexity in practical systems. Conventional model-driven methods, e.g., Akaikes information criterion and minimum description length, suffer from severe performance degradation when one-bit quantization is implemented with only the sign information of signal available. In this paper, the well-known Arcsine Law is explored to recover a normalized covariance matrix, and then a model-aided neural network is established to estimate the source number. Specially, two eigenvalues-based networks, an eigenvalues-based regression neural network (ERNN) and an eigenvalues-based classification neural network (ECNN), are trained, while considering part of the eigenvalues of normalized covariance matrix as input data and source number as the corresponding output label of the neural network, respectively, for source number detection. Experimental results show that the proposed method outperforms the state-of-the-art approaches in terms of accuracy.
更多查看译文
关键词
One-bit,source number detection,neural network,model-driven
AI 理解论文
溯源树
样例
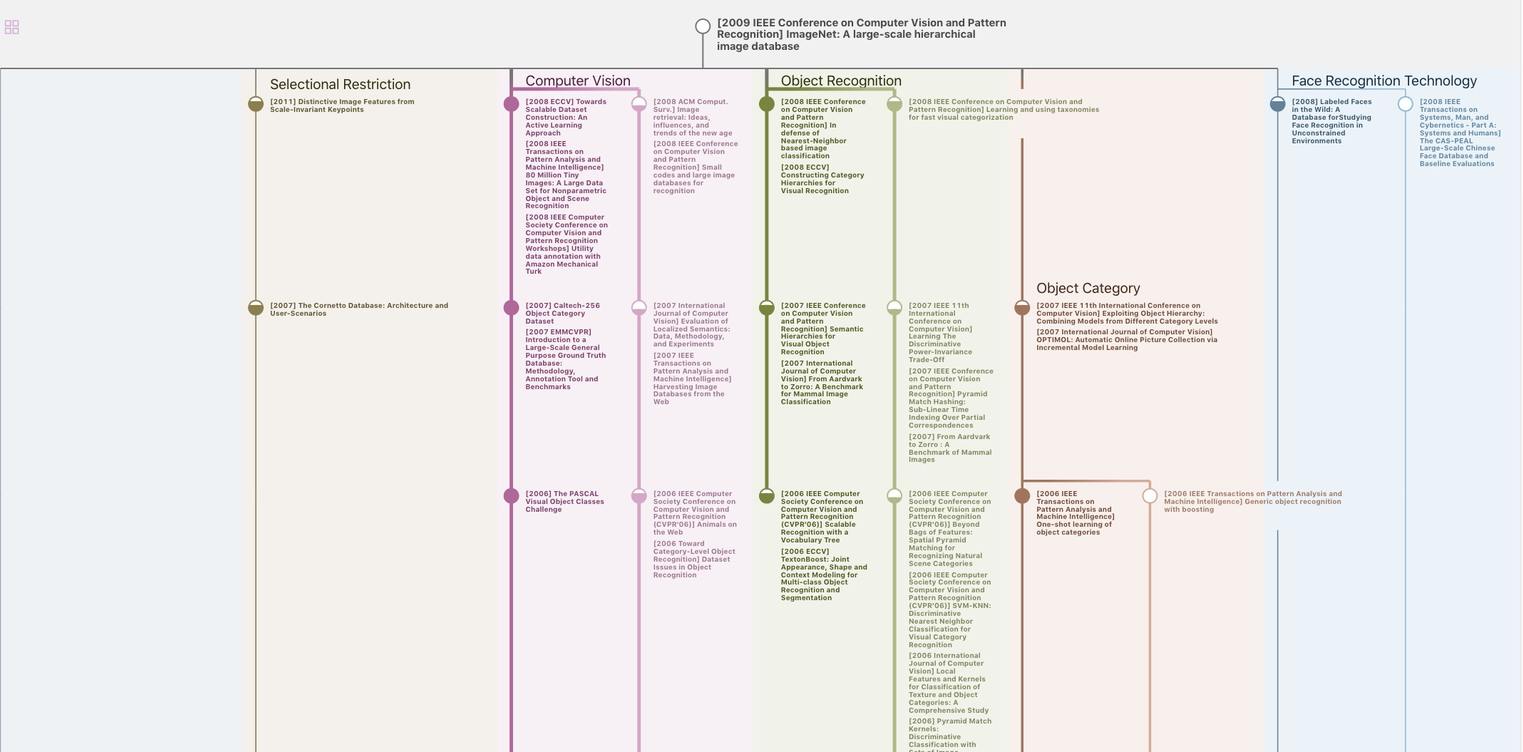
生成溯源树,研究论文发展脉络
Chat Paper
正在生成论文摘要