Interpretable deep dictionary learning for sound speed profiles with uncertainties.
The Journal of the Acoustical Society of America(2023)
摘要
Uncertainties abound in sound speed profiles (SSPs) measured/estimated by modern ocean observing systems, which impede the knowledge acquisition and downstream underwater applications. To reduce the SSP uncertainties and draw insights into specific ocean processes, an interpretable deep dictionary learning model is proposed to cater for uncertain SSP processing. In particular, two kinds of SSP uncertainties are considered: measurement errors, which generally exist in the form of Gaussian noises; and the disturbances/anomalies caused by potential ocean dynamics, which occur at some specific depths and durations. To learn the generative patterns of these uncertainties while maintaining the interpretability of the resulting deep model, the adopted scheme first unrolls the classical K-singular value decomposition algorithm into a neural network, and trains this neural network in a supervised learning manner. The training data and model initializations are judiciously designed to incorporate the environmental properties of ocean SSPs. Experimental results demonstrate the superior performance of the proposed method over the classical baseline in mitigating noise corruptions, detecting, and localizing SSP disturbances/anomalies.
更多查看译文
关键词
interpretable deep dictionary learning,sound speed profiles,uncertainties
AI 理解论文
溯源树
样例
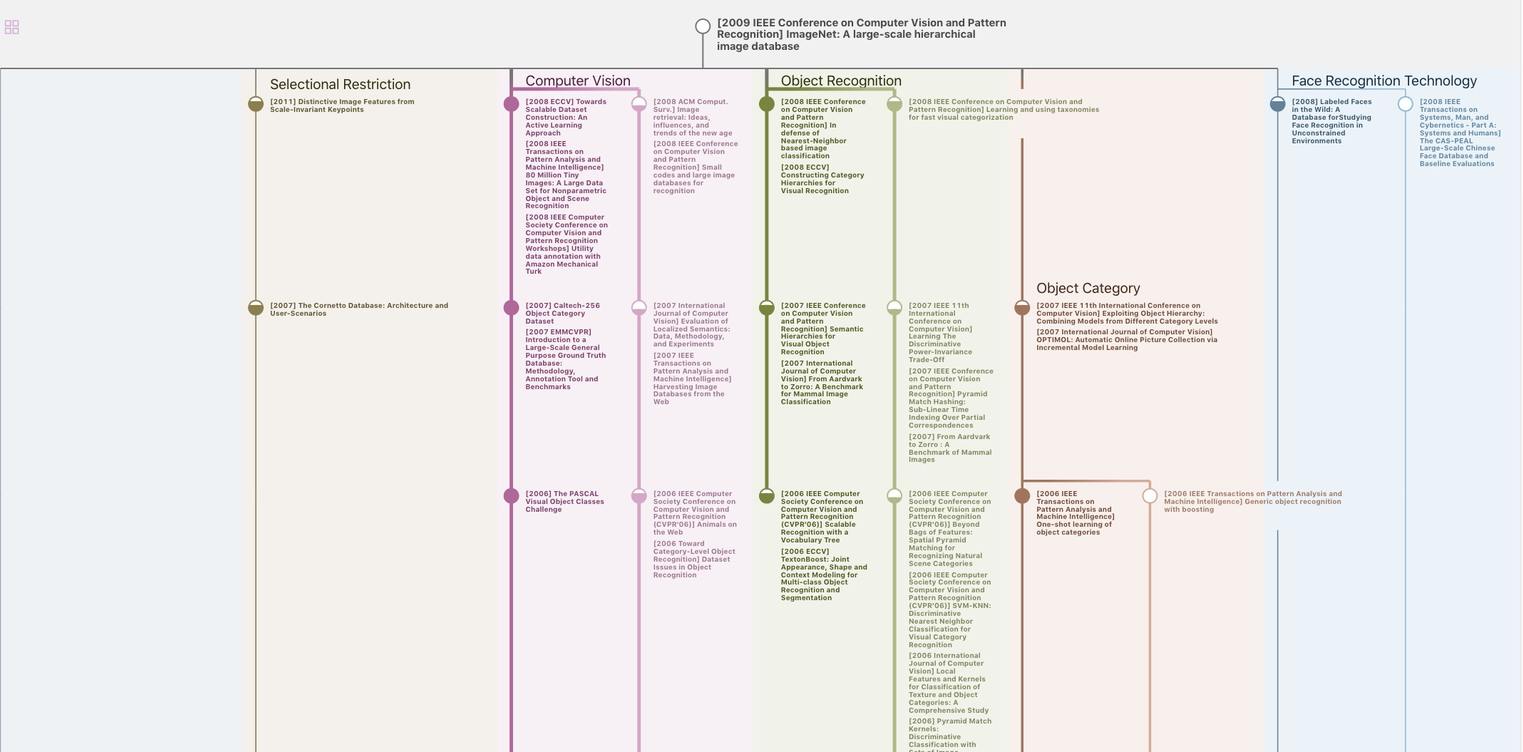
生成溯源树,研究论文发展脉络
Chat Paper
正在生成论文摘要