An Enhanced DFFNN for Location-Based Services of Indoor Device-Free Submissive Localization
2022 5th Information Technology for Education and Development (ITED)(2022)
摘要
With no associated devices, device-free localization (DFL) uses wireless sensor networks to find a target. DFL has created comprehensive applications, smart cities and the Internet of Things (IoT), among other things. This technique has attracted significant attention from various fields, increasing the demand for tracking indoor location-based services. The critical challenge in DFL is a way to retrieve essential characteristics to illustrate raw signals with various locations linked with diverse patterns. The complexity of an indoor environment with limited space has created low indoor positioning reliability and effectiveness problems. Therefore, this study proposes and formulated an image classification problem for the DFL problem by initially transforming the receiving signal strength (RSS) inputs into picture frames. The feature extraction from raw signals was performed using Deep Feed-Forward Neural Network (DFFNN) and deep auto-encoder (DAE) to fine-turning for classification. The DAE combined DFFNN were used for signal reconstruction and feature learning to present the DFL better. The findings revealed an accuracy of 100% using real-world data collected, and a signal-to-noise ratio over −5dB, 0dB, and 5dB was used to measure the react to noisy data. Moreover, in IoT applications, its time cost is very fast in single activity by 5ms for classification. The proposed method is better in noiseless and noisy situations, localization accuracy, and other related techniques.
更多查看译文
关键词
Device-free localization,Wireless sensor networks,Deep Feed-Forward Neural Network,Deep auto-encoder,Received signal strength,Classification,Indoor environment
AI 理解论文
溯源树
样例
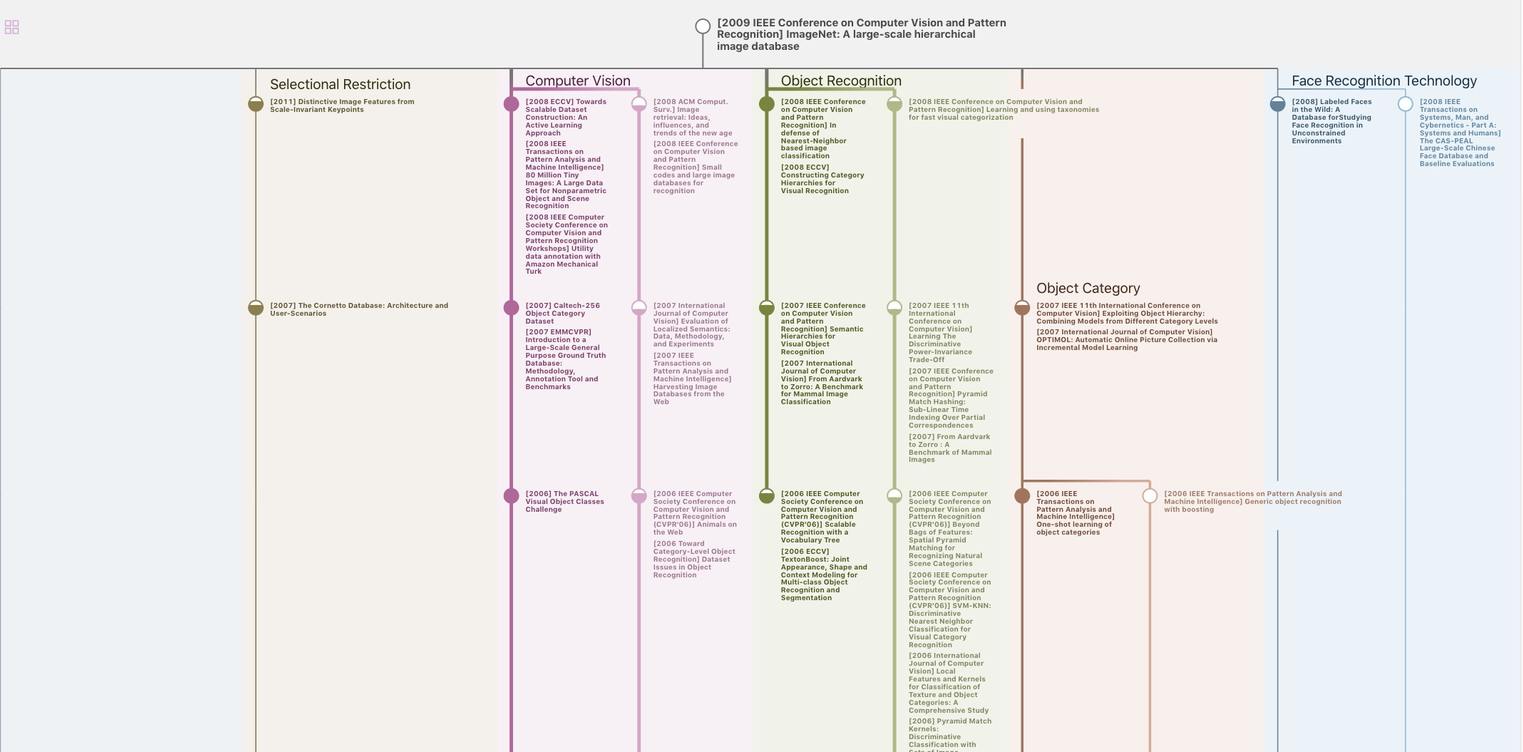
生成溯源树,研究论文发展脉络
Chat Paper
正在生成论文摘要