A Hybrid Deep Cascade-ResNet Model for Detecting Alzheimer's Stages in MR Images
2022 International Engineering Conference on Electrical, Energy, and Artificial Intelligence (EICEEAI)(2022)
摘要
Neuroimaging is used for diagnosing neuropathological disorders, such as Alzheimer's disease (AD). In this study, a hybrid convolutional neural network (CNN) model is evaluated for its ability to improve AD diagnosis. Accordingly, we determine that using the CascadeNet and ResNet algorithms, we can precisely classify Alzheimer's disease stages in magnetic resonance imaging (MRI). Based on Kaggle datasets, we validate the proposed architecture and compare its performance with that of similar deep learning methods. We achieved 99.02% accuracy using quaternary classification. There was an accuracy of quaternary classification between all AD subtypes in the proposed model. Cascade-ResNet has better classification accuracy and processing complexity than other deep CNNs. The proposed model outperforms the current state-of-the-art deep learning methods when applied to MRI. Furthermore, the hybrid Cascade-ResNet performed well without the need for feature extraction and was insensitive to existing noise in the imaging. Consequently, untrained operators are able to apply the proposed model to digital patient imaging data. By distinguishing between AD patients and healthy individuals, this research can enhance value-based care in clinical settings.
更多查看译文
关键词
Alzheimer's disease,deep learning,magnetic resonance imaging,CascadeNet,ResNet,convolutional neural network
AI 理解论文
溯源树
样例
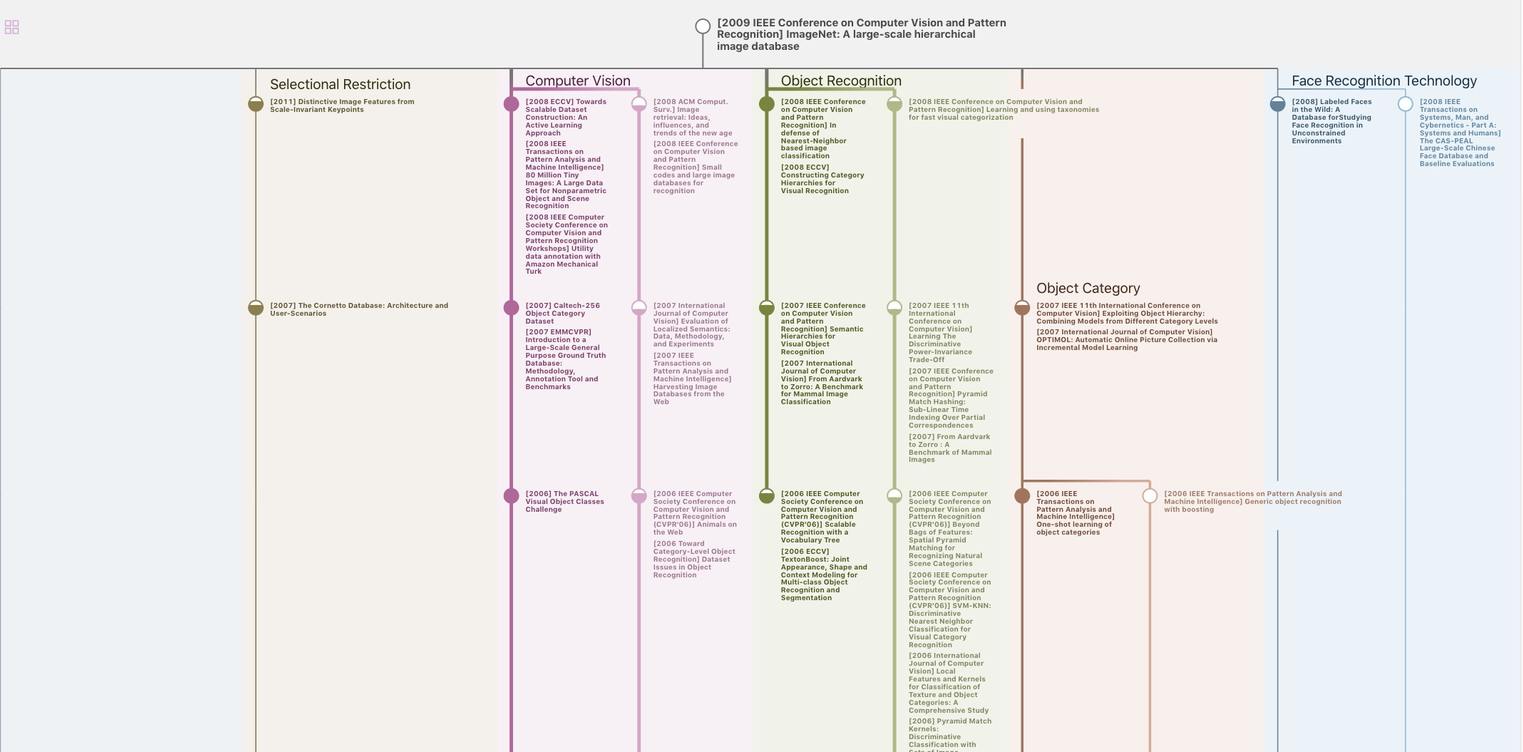
生成溯源树,研究论文发展脉络
Chat Paper
正在生成论文摘要