High-Throughput FPGA-Based Inference of Gradient Tree Boosting Models for Position Estimation in PET Detectors
IEEE Transactions on Radiation and Plasma Medical Sciences(2023)
摘要
In modern large-scale positron emission tomography (PET) systems, transferring the digitized raw detector data at high count rates to a centralized processing unit is a challenge. Processing data on field programmable gate arrays (FPGAs) close to the detectors can reduce data early on and improve scalability of the PET system. We present and evaluate an FPGA implementation of gradient tree boosting (GTB) for 1-D position estimation of gamma interactions in the scintillator. GTB is a supervised machine learning algorithm based on building ensembles of binary decision trees. Models were trained offline and inferred in an FPGA (XC7K410T-2FFG676 Kintex-7). Input features and GTB parameters influencing both positioning performance and model size were varied while evaluating the inferred models concerning data throughput and FPGA resource consumption as well as positioning performance. We achieved throughputs per detector between
$2.94\times 10^{6}$
and
$4.55\times 10^{6}$
gamma interactions per second. For an optimized GTB model, resource consumption could be reduced by factors of 17 and 10 to less than 1% (
$2.51\times 10^{3}$
look-up tables) of available logic and 1.26% (20 BRAMs) of memory resources, while maintaining a positioning performance of 98.63% when compared to the model with the best positioning performance. The presented framework can be easily adapted to other photosensors and scintillator influencing.
更多查看译文
关键词
Data processing,decision trees,field programmable gate arrays (FPGAs),gradient tree boosting (GTB),positron emission tomography (PET)
AI 理解论文
溯源树
样例
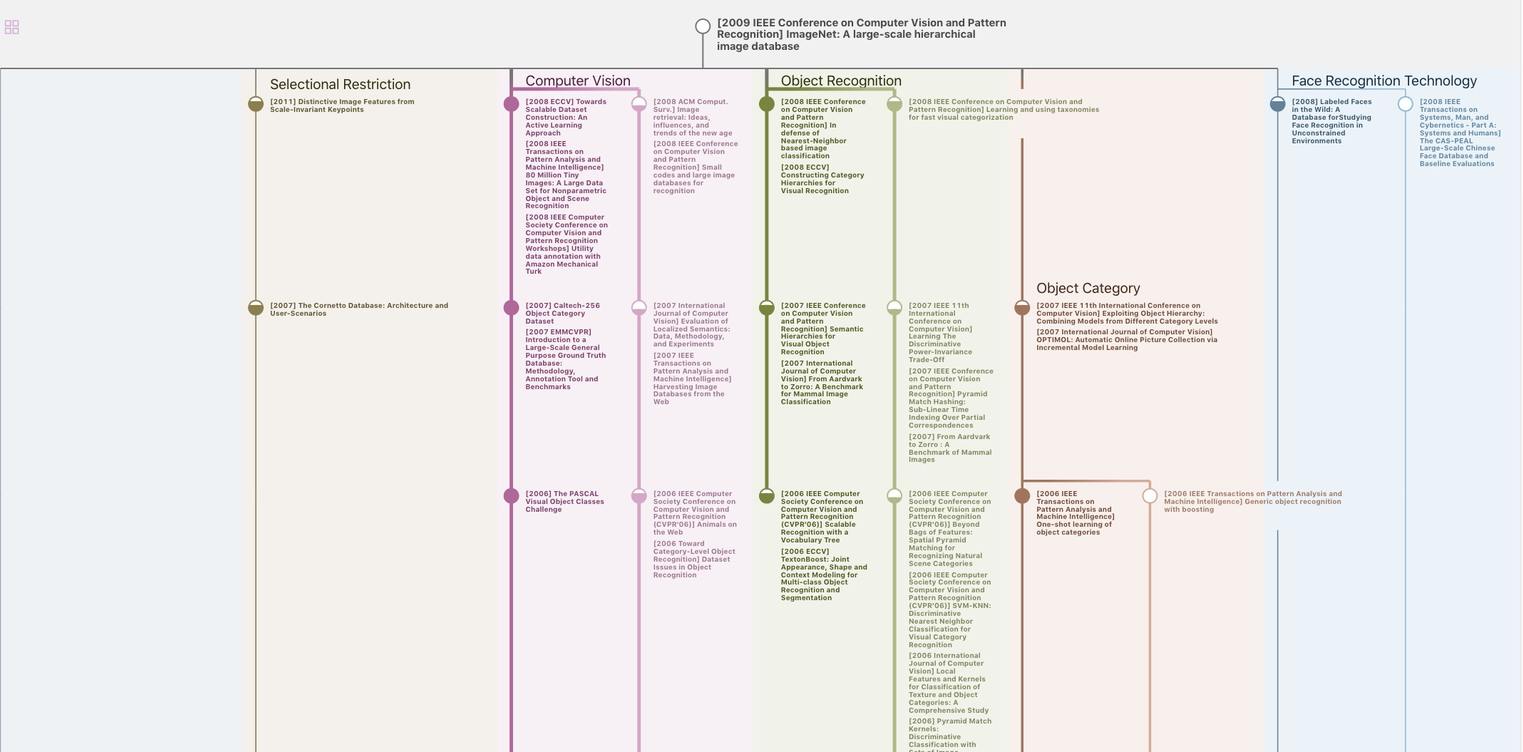
生成溯源树,研究论文发展脉络
Chat Paper
正在生成论文摘要