A data-efficient deep learning tool for scRNA-Seq label transfer in neuroscience
biorxiv(2023)
摘要
Large single-cell RNA datasets have contributed to unprecedented biological insight. Often, these take the form of cell atlases and serve as a reference for automating cell labeling of newly sequenced samples. Yet, classification algorithms have lacked the capacity to accurately annotate cells, particularly in complex datasets. Here we present SIMS (Scalable, Interpretable Machine Learning for Single-Cell), an end-to-end data-efficient machine learning pipeline for discrete classification of single-cell data that can be applied to new datasets with minimal coding. We benchmarked SIMS against common single-cell label transfer tools and demonstrated that it performs as well or better than state of the art algorithms. We then use SIMS to classify cells in one of the most complex tissues: the brain. We show that SIMS classifies cells of the adult cerebral cortex and hippocampus at a remarkably higher accuracy than state-of-the-art single cell classifiers. This accuracy is maintained in trans-sample label transfers of the adult human cerebral cortex. We then apply SIMS to classify cells in the developing brain and demonstrate a high level of accuracy at predicting neuronal subtypes, even in periods of fate refinement. Finally, we apply SIMS to single cell datasets of cortical organoids to predict cell identities in previously unclassified cells and to uncover genetic variations in the developmental trajectories of organoids derived from different pluripotent stem cell lines. Altogether, we show that SIMS is a versatile and robust tool for cell-type classification from single-cell datasets.
### Competing Interest Statement
J.L., V.D.J. and M.A.M.-R. have submitted provisional patents relating to the work described in this manuscript. The authors declare no other competing interest.
更多查看译文
关键词
neuroscience,deep learning,data-efficient,scrna-seq
AI 理解论文
溯源树
样例
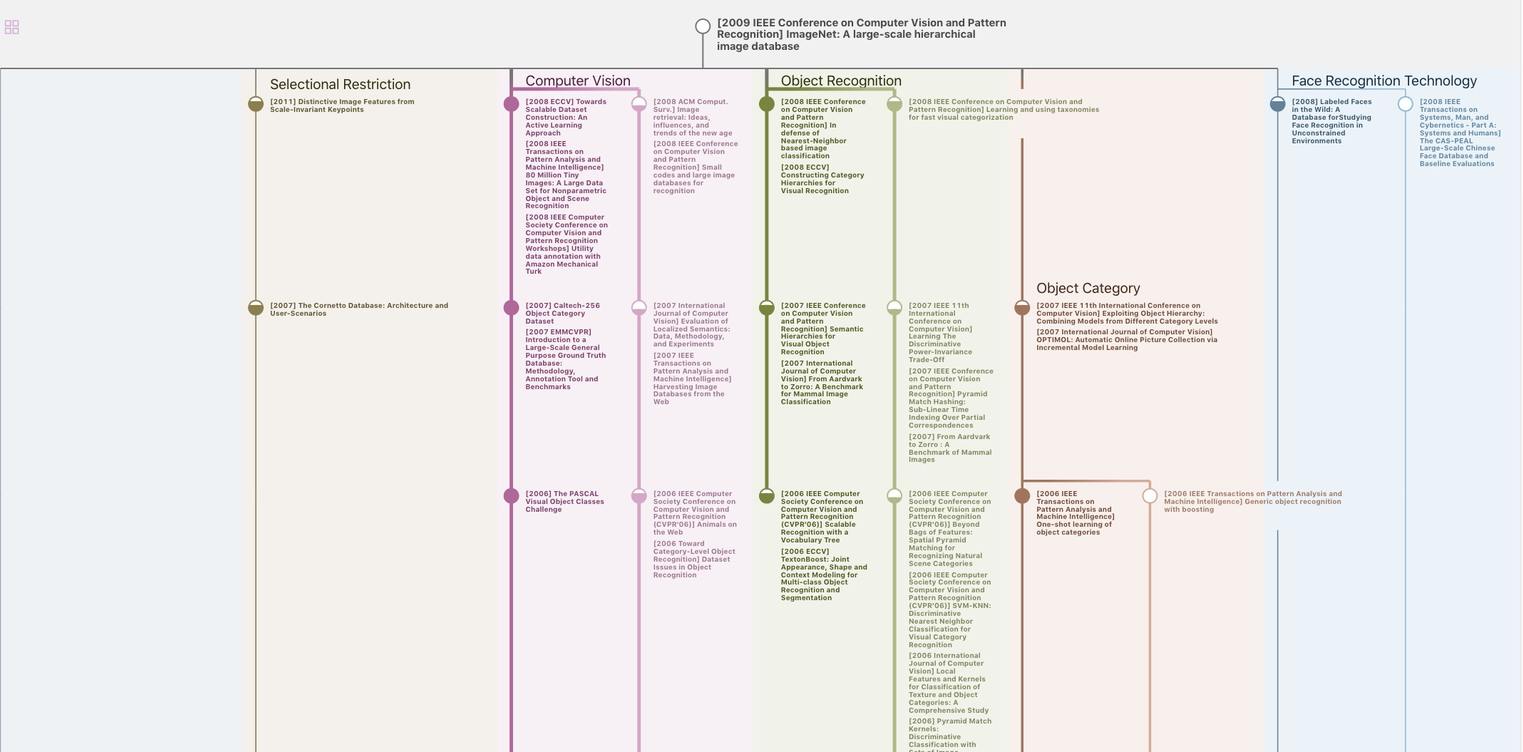
生成溯源树,研究论文发展脉络
Chat Paper
正在生成论文摘要