Implementation of network embedding strategy on proteome datasets from multi-source cancers to demonstrate marker proteins of cancers
AUSTRALIAN JOURNAL OF CHEMISTRY(2023)
摘要
The rapid production of high-throughput cancer omics data provides valuable data resources for revealing the pathogenesis, prognosis prediction and treatment strategies of cancers. However, the huge data scale brings great challenges to data analysis. Therefore, we applied the represen-tation learning method to the joint analysis of biomedical network and omics data. According to the protein expression profile of patients with early-stage hepatocellular carcinoma, 15 dimen-sional embedding vectors of 101 samples were obtained. Unsupervised learning was then used to cluster the embedded vectors of the samples, and we found that the clustering of the embedded vectors of the samples was consistent with the clustering of the original data. Therefore, the spatial distribution of embedded vectors can maintain the similarity of samples. New pan-cancer subtypes were obtained by joint embedding the expression profile of pan-cancer proteomic and pathway network data. Nine hunded and forty four proteins such as KIF2C, AURKA, ATP1B1, BDH1 and C6ORF106 were found to be significantly related to these subtypes, and 143 biological pathways or processes such as p53 signaling pathway, nucleotide synthesis, immune diseases, metabolism, cholesterol synthesis and transportation were found to be significantly related to these subtypes. These results show that the representation learning system developed can realize the seamless connection between the omics data and the pathway network. Our method is expected to help mine the biological knowledge contained in the omics data and provide a new perspective for further explanation of the molecular mechanism.
更多查看译文
关键词
biological pathway,network embedding,pan-cancer analysis,proteomics,representation learning
AI 理解论文
溯源树
样例
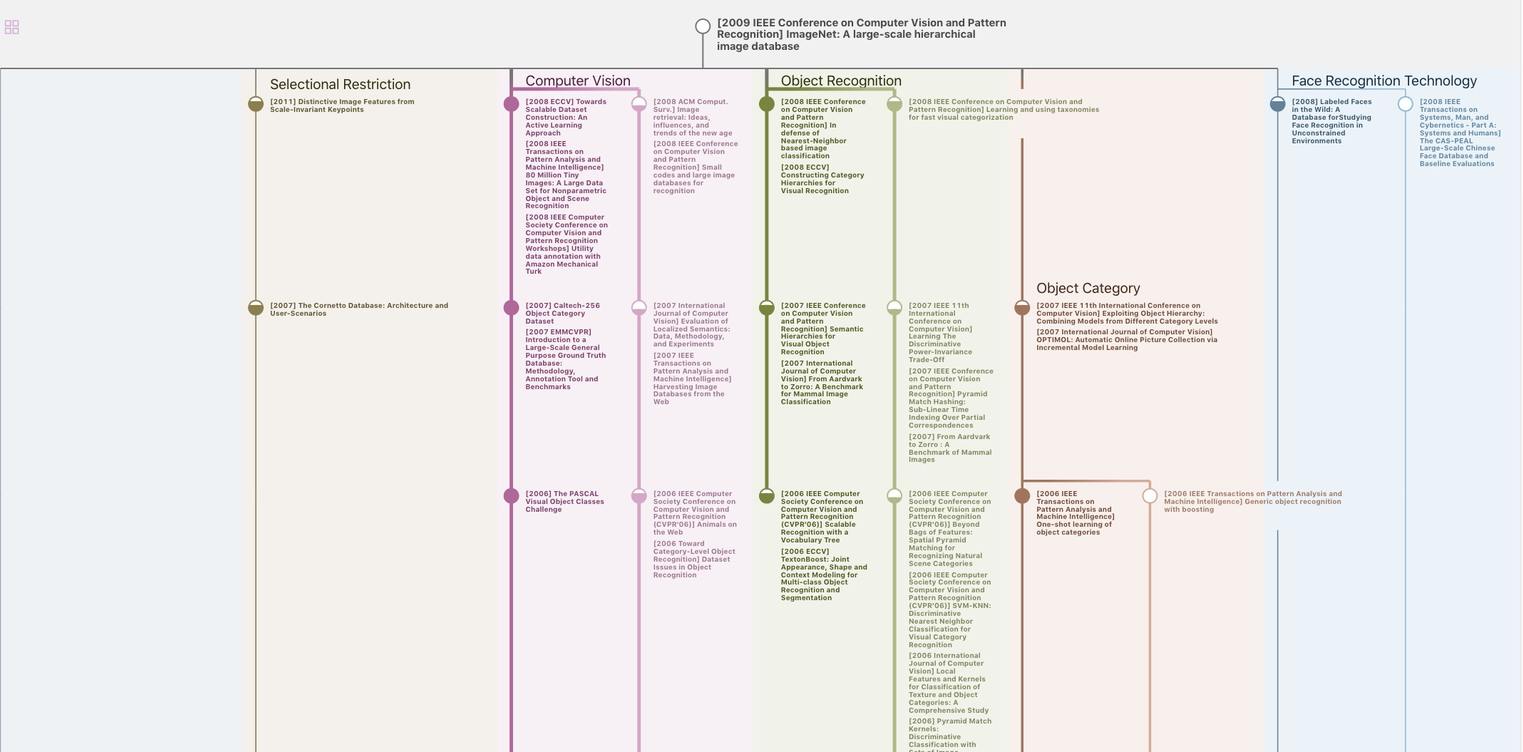
生成溯源树,研究论文发展脉络
Chat Paper
正在生成论文摘要