New Challenges in (Bio)Analytical Sample Treatment Procedures for Clinical Applications
SEPARATIONS(2023)
摘要
The primary cause of poor and ambiguous results obtained from the bioanalytical process is the sample pre-treatment, especially in clinical analysis because it involves dealing with complex sample matrices, such as whole blood, urine, saliva, serum, and plasma. So, the aim of this review is to focus attention on the classical and new techniques of pre-treatment for biological samples used in the bioanalytical process. We discussed the methods generally used for these types of complex samples. Undoubtedly, it is a daunting task to deal with biological samples because the analyst may encounter a substantial loss of the analytes of interest, or the overall analysis may be too time-consuming. Nowadays, we are inclined to use green solvents for the environment, but without sacrificing analytical performance and selectivity. All the characteristics mentioned above should be added to the difficulty of the withdrawal of samples like blood because it can be an invasive practice. For these reasons, now we can also find in the literature the use of saliva as alternative biological samples and new techniques that do not require substantial sample pre-treatment, such as fabric phase sorptive extraction (FPSE). The text has been divided into the following two distinct parts: firstly, we described clinical applications under different subsections, such as anticancer drugs, antibiotics, vitamins, antivirals, non-steroidal anti-inflammatory drugs, statin, imidazoles, and triazoles. The second part is dedicated to sample preparation techniques for diagnostic purposes and is divided into the following different sample preparation techniques: solid-phase microextraction (SPME), microextraction by packed sorbent (MEPS), dispersive liquid-liquid microextraction (DDLME), and fabric phase sorptive extraction (FPSE).
更多查看译文
关键词
clinical analytical chemistry,drug analysis,sample treatment,Green Analytical Chemistry
AI 理解论文
溯源树
样例
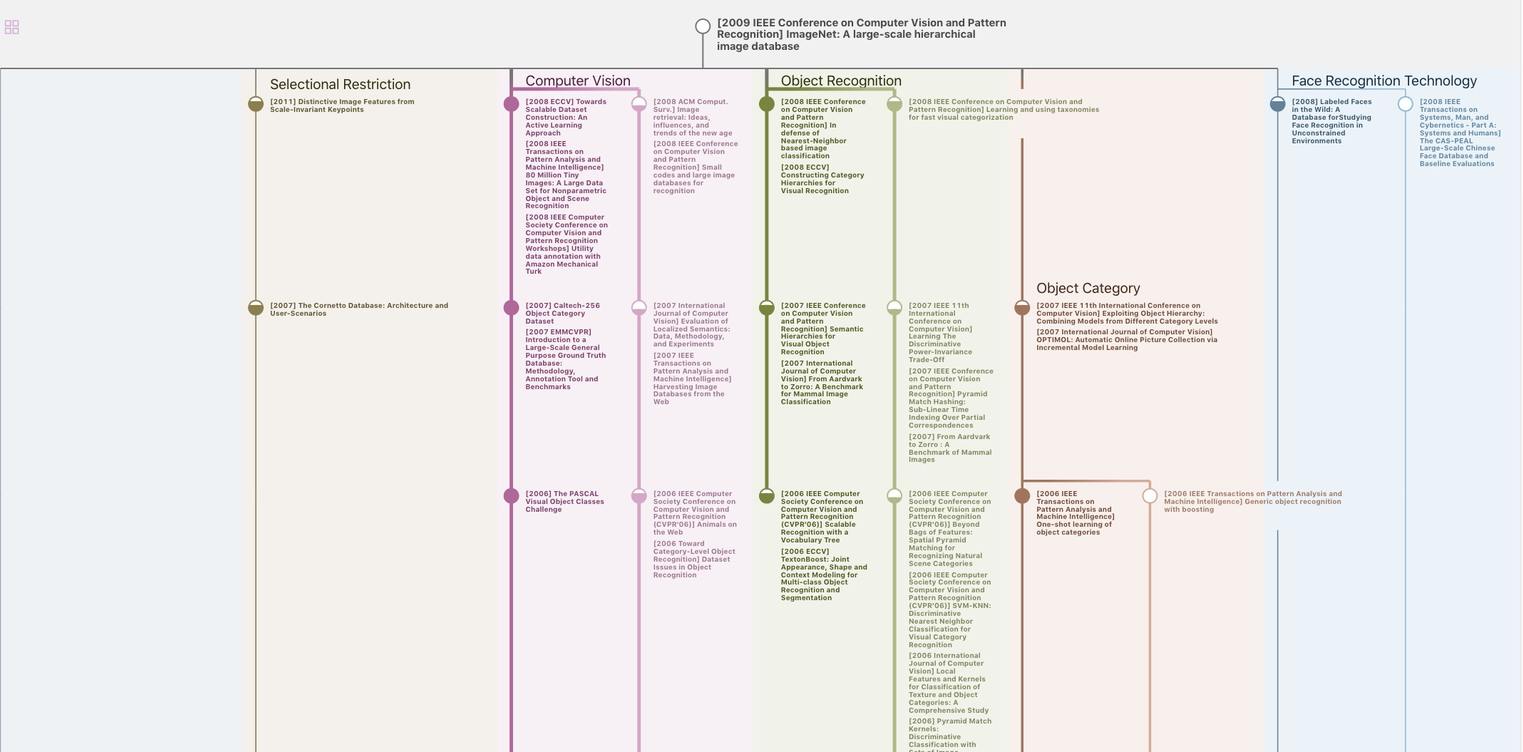
生成溯源树,研究论文发展脉络
Chat Paper
正在生成论文摘要