Effective Incomplete Multi-View Clustering via Low-Rank Graph Tensor Completion
MATHEMATICS(2023)
摘要
In the past decade, multi-view clustering has received a lot of attention due to the popularity of multi-view data. However, not all samples can be observed from every view due to some unavoidable factors, resulting in the incomplete multi-view clustering (IMC) problem. Up until now, most efforts for the IMC problem have been made on the learning of consensus representations or graphs, while many missing views are ignored, making it impossible to capture the information hidden in the missing view. To overcome this drawback, we first analyzed the low-rank relationship existing inside each graph and among all graphs, and then propose a novel method for the IMC problem via low-rank graph tensor completion. Specifically, we first stack all similarity graphs into a third-order graph tensor and then exploit the low-rank relationship from each mode using the matrix nuclear norm. In this way, the connection hidden between the missing and available instances can be recovered. The consensus representation can be learned from all completed graphs via multi-view spectral clustering. To obtain the optimal multi-view clustering result, incomplete graph recovery and consensus representation learning are integrated into a joint framework for optimization. Extensive experimental results on several incomplete multi-view datasets demonstrate that the proposed method can obtain a better clustering performance in comparison with state-of-the-art incomplete multi-view clustering methods.
更多查看译文
关键词
incomplete multi-view clustering,common representation,low-rank completion,similarity graph
AI 理解论文
溯源树
样例
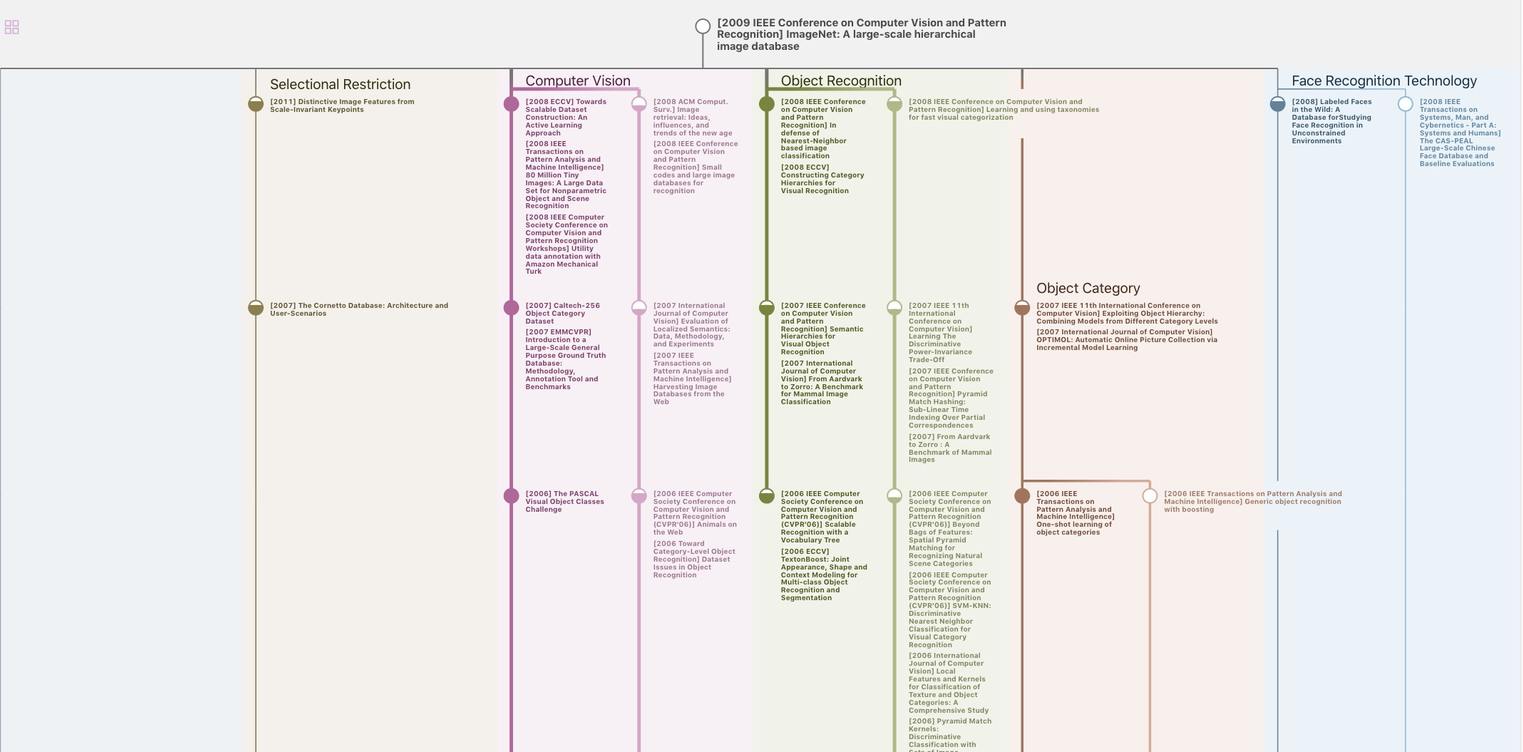
生成溯源树,研究论文发展脉络
Chat Paper
正在生成论文摘要