COVID-19 diagnosis prediction using classical-to-quantum ensemble model with transfer learning for CT scan images
IMAGING SCIENCE JOURNAL(2021)
摘要
At present, there are problems of low detection efficiency and accuracy in chest CT images of COVID-19 as well as limited computational power of deep learning model training. Developing a classical-to-quantum (CQ) ensemble model with transfer learning to efficiently detect patients with COVID-19 using chest CT images.: Attributes were extracted from chest CT scans using pre-trained networks ResNet50, VGG16 and AlexNet, while dressed quantum circuits were used as classifiers. The overall accuracy of the CQ method based on three aforementioned networks on the chest CT dataset is 83.2%, 86.2% and 85.0%, respectively. The proposed ensemble model has a precision of 89.0% for pneumonia samples, an overall accuracy of 88.6% and a pneumonia class recall rate of 83.0%. In addition, to further verify the robustness of the ensemble model, breast ultrasound and brain tumour images were used in it. The suggested ensemble approach is effective for classifying and detecting medical pictures with complicated features, particularly for detecting COVID-19 patients using chest CT images.
更多查看译文
关键词
COVID-19,deep learning,transfer learning,dressed quantum circuits,ensemble model
AI 理解论文
溯源树
样例
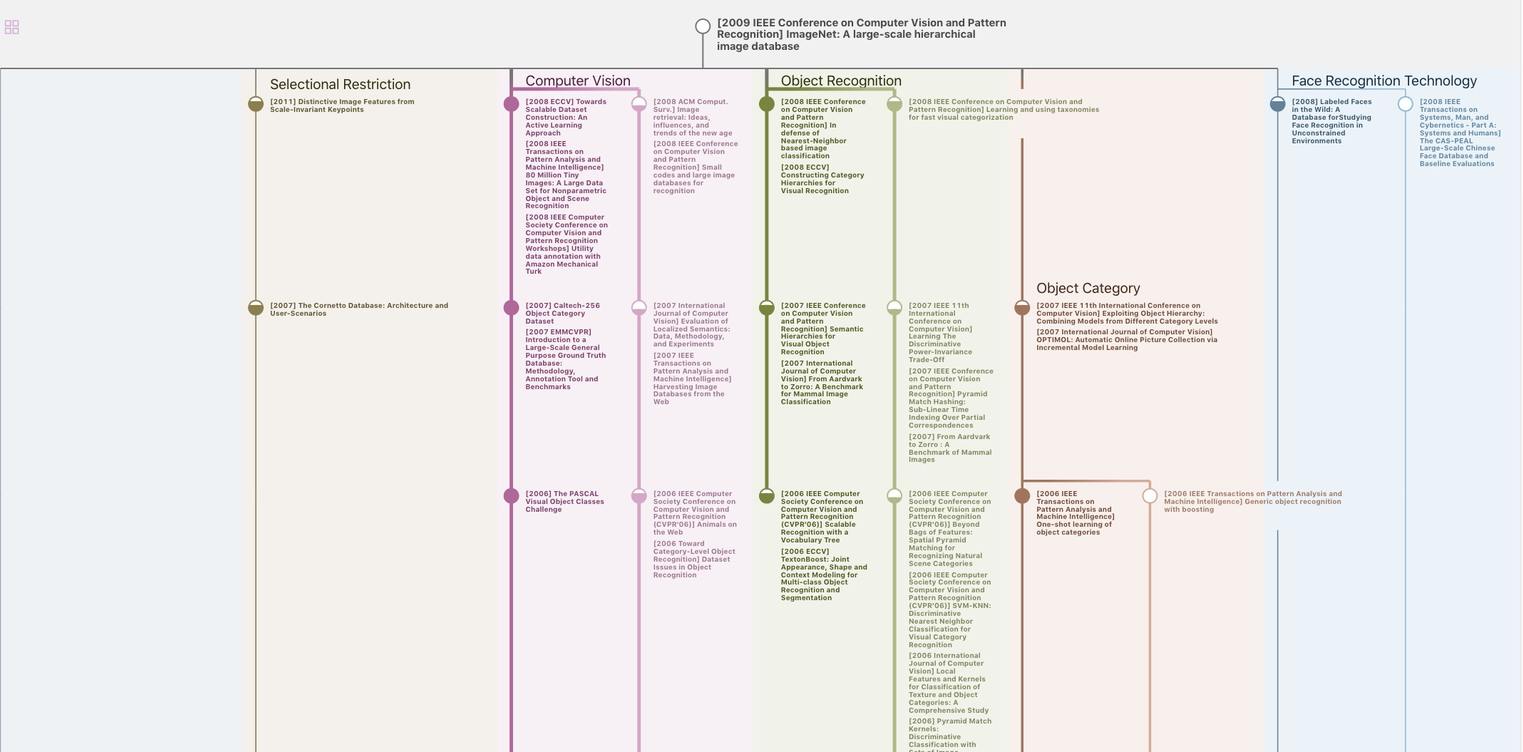
生成溯源树,研究论文发展脉络
Chat Paper
正在生成论文摘要