Heuristics Integrated Deep Reinforcement Learning for Online 3D Bin Packing
IEEE TRANSACTIONS ON AUTOMATION SCIENCE AND ENGINEERING(2024)
摘要
Online 3D Bin Packing Problem (3D-BPP) has a wide range of industrial applications and there is an emerging research interest in learning optimal bin packing policy and deploying it for real logistics applications. From the heuristic methods to the deep reinforcement learning (DRL) methods, the previous works have proposed many solutions to solve the online 3D-BPP. However, none of them have studied what and how heuristics can be modelled into DRL to build a more effective and practical bin packing pipeline. In this work, we thoroughly investigate what heuristics can be used in online 3D-BPP and how to effectively integrate the heuristics with the DRL. First, we design 3 different heuristics based on the physical rules of the real world and the experiences of the human packers, including the Physics-Heuristics, the Packing-Heuristics and the Unpacking-Heuristics. Second, we model the 3 types of heuristics into the DRL framework and propose a novel heuristic DRL method to solve the online 3D-BPP. Extensive experimental results show that our method achieves state-of-the-art bin packing performance and the resulting real-world system is able to reliably finish the bin packing task in real logistics scenarios.
更多查看译文
关键词
Logistics,Three-dimensional displays,Task analysis,Robots,Optimization,Reinforcement learning,Deep learning,Bin packing,robotics,warehouse automation,deep reinforcement learning
AI 理解论文
溯源树
样例
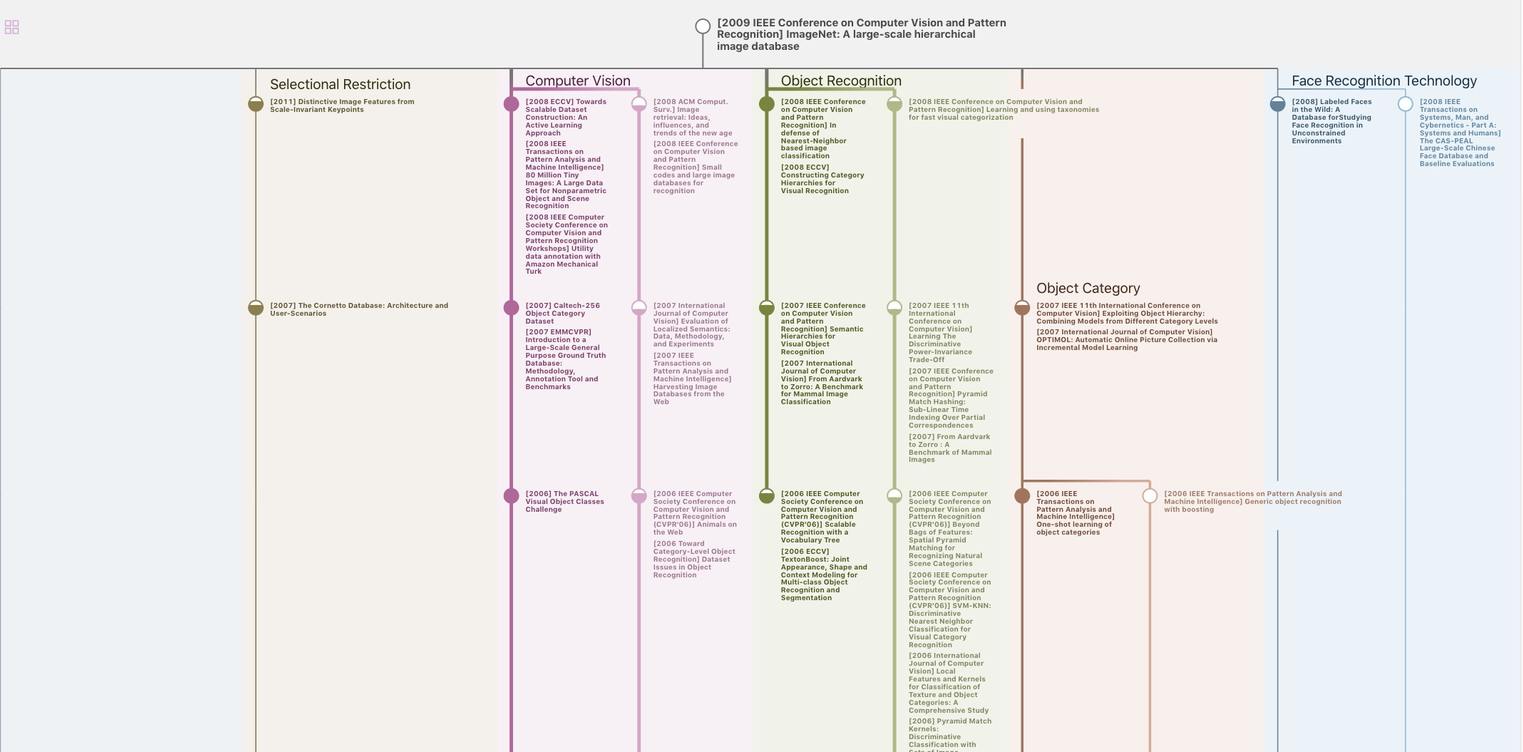
生成溯源树,研究论文发展脉络
Chat Paper
正在生成论文摘要