Knowledge-Aware Topological Networks for Recommendation
KNOWLEDGE GRAPH AND SEMANTIC COMPUTING: KNOWLEDGE GRAPH EMPOWERS THE DIGITAL ECONOMY, CCKS 2022(2022)
摘要
Knowledge graphs (KGs) play a critical role in recommender systems, aiming to provide diverse, accurate, and explainable recommendations to users. Enhanced with KGs, recommender systems are able to leverage valuable auxiliary information, which is beneficial to predict new user-item interactions. Specifically, the connectivity between relations and entities in a KG can reveal the structural and semantic information, as well as help to provide inferences for user choices. However, the information of the holistic topological structure in KGs has not been fully taken into account in most existing studies. To this end, we propose the Knowledge-aware Topological Recurrent Network (KTRN), an end-to-end network for recommendation with recurrent neural network and knowledge graph embedding. To simultaneously discover sequential dependencies and semantic information in a KG, we consider both relevant paths and triplets. Moreover, we focus on the importance of relation-entity pairs in learning representations, rather than treating relations and entities as independent units. We conduct experiments on three public datasets about movie, book, and music recommendation scenarios, and extensive experimental results show that our method outperforms benchmark approaches.
更多查看译文
关键词
Recommendation system,Knowledge graph,Representation learning
AI 理解论文
溯源树
样例
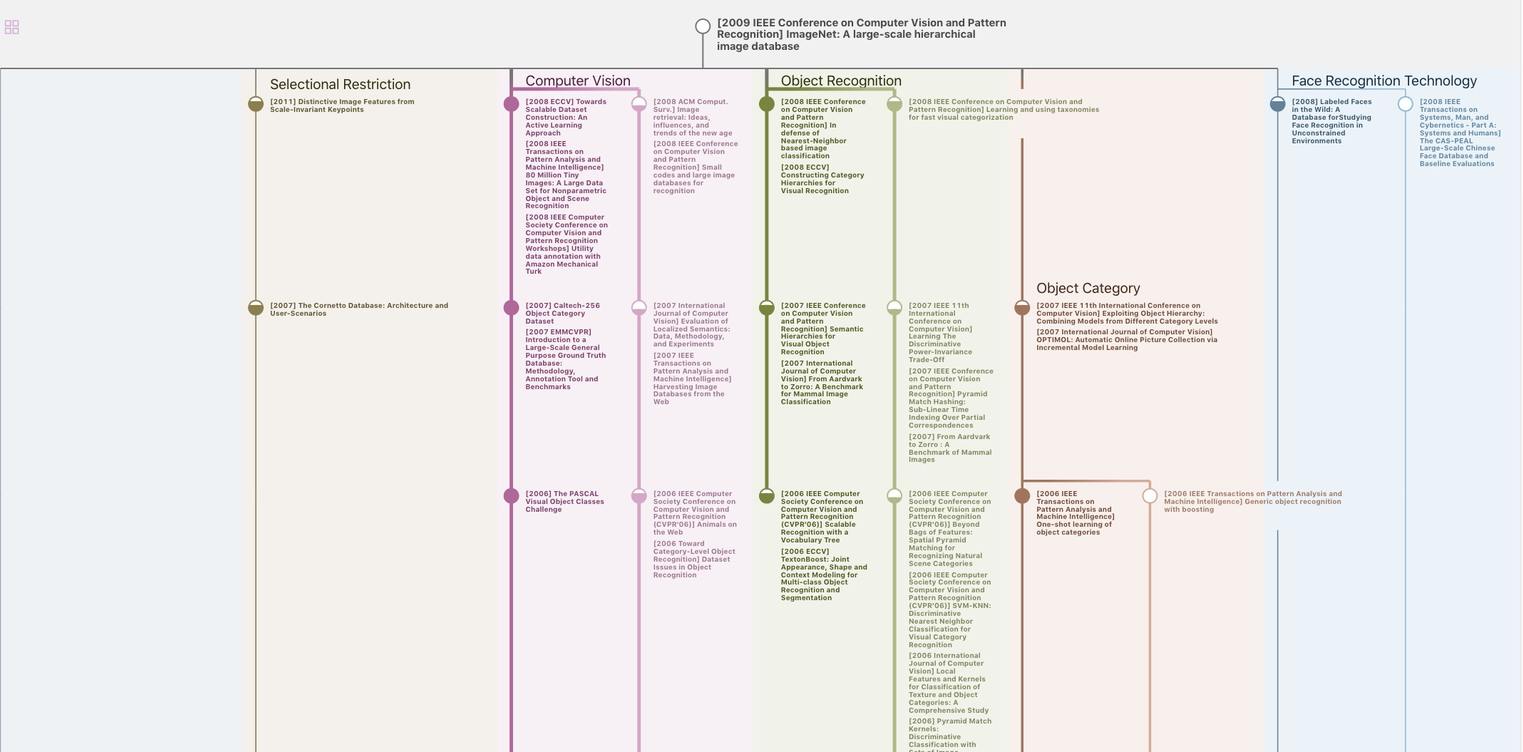
生成溯源树,研究论文发展脉络
Chat Paper
正在生成论文摘要