CARL-YOLOF: A well-efficient model for digital printing fabric defect detection
JOURNAL OF ENGINEERED FIBERS AND FABRICS(2022)
摘要
Deep-learning models have been successfully applied to fabric defect detection fields. However, due to the lack of available dataset for the digital printing fabric defect detection field, the study is still under-explored. Besides, there are two tough challenges that currently existing deep-learning models cannot resolve well. On the one hand, the actual production requires the models to improve its detection accuracy as much as possible while ensuring real-time detection. On the other hand, the objectives of classification and localization are correlated, so the link between classification and localization should be established for an excellent object detection model. To solve these problems, such Classification-Aware Regression Loss (CARL) is embedded into the YOLOF to correlate the classification and localization tasks so that the model is named CARL-YOLOF. The performance of the proposed model has been trained and evaluated by the self-built digital printing fabric defect detection dataset (DPFD-DET). Experimental results demonstrate that CARL-YOLOF achieves 0.54 AP on COCO metrics, which achieves a gain of 0.04 compared with YOLOF. Besides, CARL-YOLOF maintains the speed advantage of YOLOF, which reaches 42 FPS. Therefore, the proposed model is suitable for the real-time digital printing fabric defect detection.
更多查看译文
关键词
Digital printing,fabric defect detection,YOLOF,CARL,deep learning
AI 理解论文
溯源树
样例
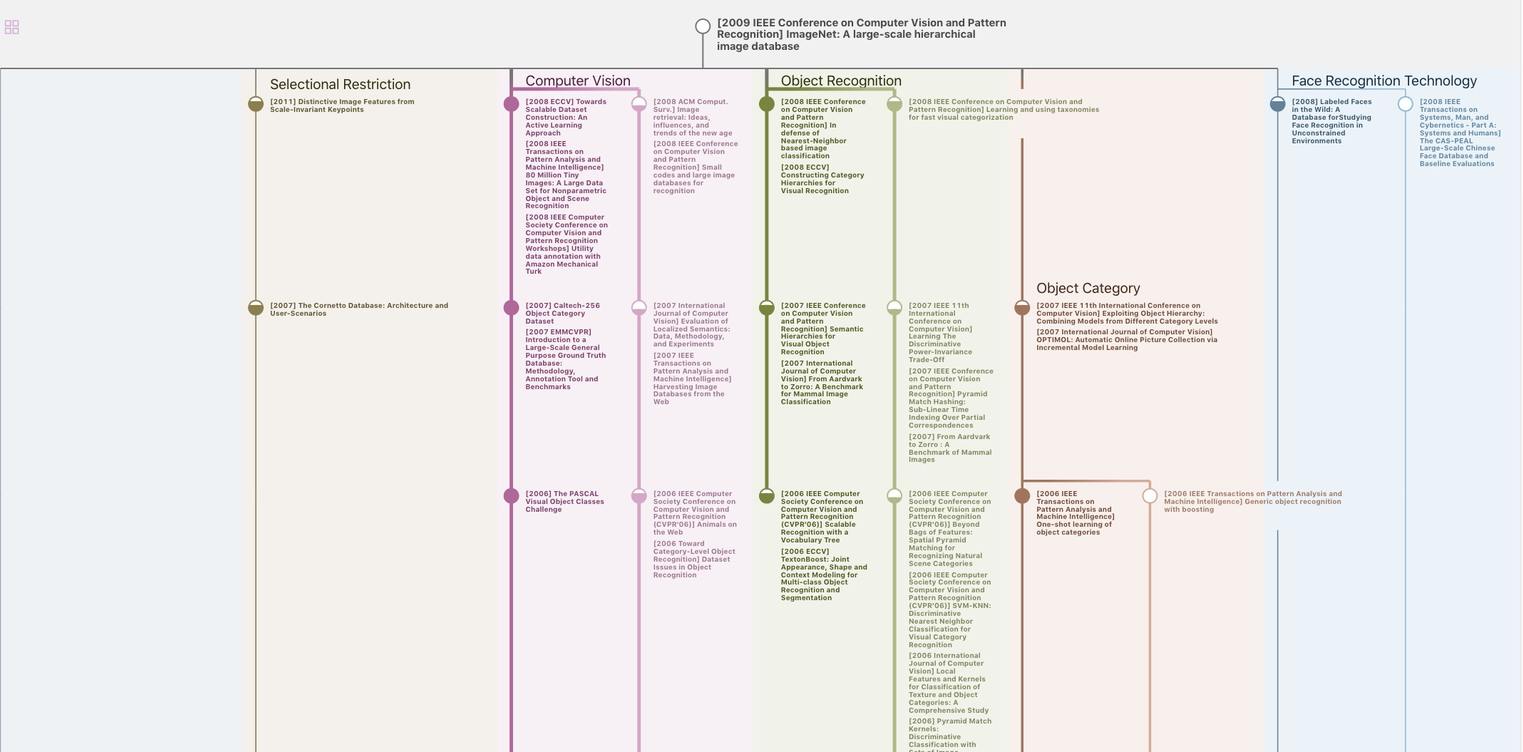
生成溯源树,研究论文发展脉络
Chat Paper
正在生成论文摘要