Nonlinear industrial process fault diagnosis with latent label consistency and sparse Gaussian feature learning
JOURNAL OF CENTRAL SOUTH UNIVERSITY(2023)
摘要
With the increasing complexity of industrial processes, the high-dimensional industrial data exhibit a strong nonlinearity, bringing considerable challenges to the fault diagnosis of industrial processes. To efficiently extract deep meaningful features that are crucial for fault diagnosis, a sparse Gaussian feature extractor(SGFE) is designed to learn a nonlinear mapping that projects the raw data into the feature space with the fault label dimension. The feature space is described by the one-hot encoding of the fault category label as an orthogonal basis. In this way, the deep sparse Gaussian features related to fault categories can be gradually learned from the raw data by SGFE. In the feature space, the sparse Gaussian (SG) loss function is designed to constrain the distribution of features to multiple sparse multivariate Gaussian distributions. The sparse Gaussian features are linearly separable in the feature space, which is conducive to improving the accuracy of the downstream fault classification task. The feasibility and practical utility of the proposed SGFE are verified by the handwritten digits MNIST benchmark and Tennessee-Eastman (TE) benchmark process, respectively.
更多查看译文
关键词
nonlinear fault diagnosis,multiple multivariate Gaussian distributions,sparse Gaussian feature learning,Gaussian feature extractor
AI 理解论文
溯源树
样例
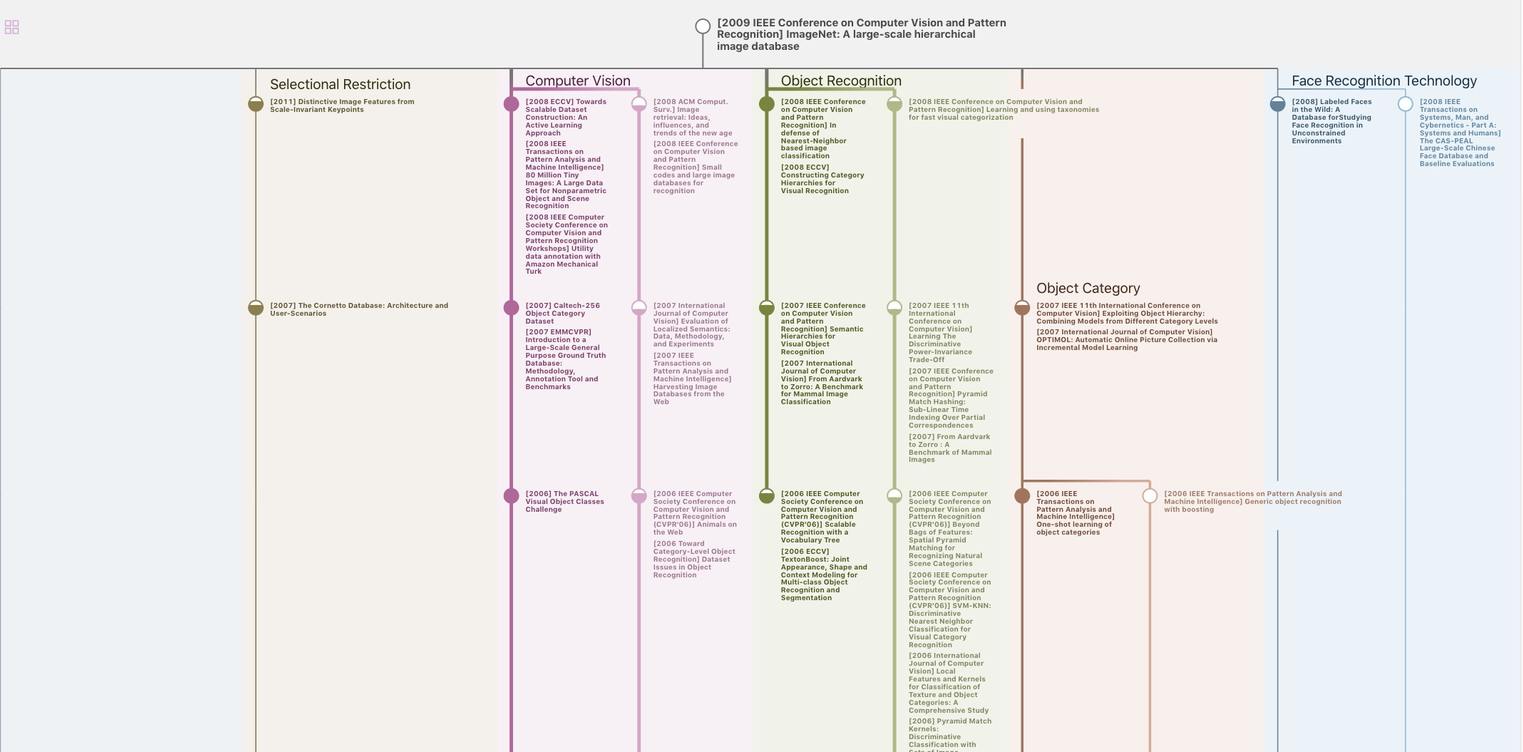
生成溯源树,研究论文发展脉络
Chat Paper
正在生成论文摘要