Vector SENM-HMT-Based Statistical Watermark Decoder in NSST–PLCT Magnitude Domain
CIRCUITS SYSTEMS AND SIGNAL PROCESSING(2023)
摘要
In this paper, we introduce a novel statistical image watermark decoder based on robust nonsubsampled Shearlet transform (NSST)-polar linear Canonical transform (PLCT) magnitude and effective vector shifted exponential normal mixtures-based hidden Markov tree (vector SENM-HMT). We begin with a detailed study on the robustness and statistical characteristics of local NSST–PLCT magnitudes of natural images. This study reveals the excellent robustness, highly non-Gaussian marginal statistics and strong dependencies of local NSST–PLCT magnitudes. We also find that conditioned on their generalized neighborhoods, the local NSST–PLCT magnitudes can be approximately modeled as shifted exponential normal variables. Meanwhile, model parameters can be estimated effectively by using density-preserving hierarchical EM (DPHEM) and upward–downward approach. Based on these findings, we model local NSST–PLCT magnitudes using a vector SENM-HMT that can capture all interscale, interdirection and interlocation dependencies. Finally, we develop a new statistical image watermark decoder using the vector SENM-HMT and maximum likelihood (ML) decision rule. Experimental results demonstrate the efficacy and superiority of the proposed approach.
更多查看译文
关键词
Image watermarking,Local NSST–PLCT magnitude,Vector SENM-HMT,DPHEM and upward–downward approach,ML watermark decoder
AI 理解论文
溯源树
样例
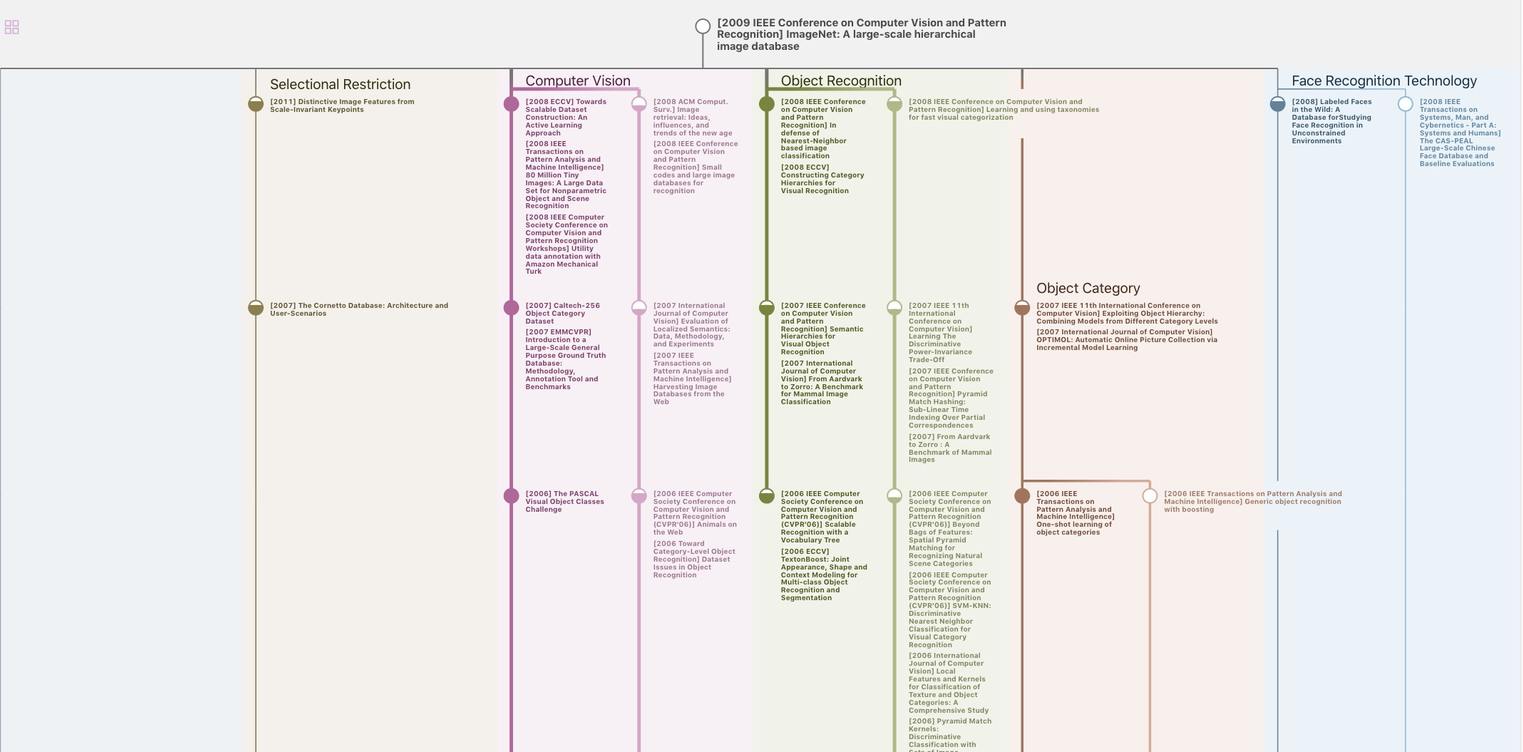
生成溯源树,研究论文发展脉络
Chat Paper
正在生成论文摘要