Spatio-Temporal Contextual Learning for Single Object Tracking on Point Clouds
IEEE TRANSACTIONS ON NEURAL NETWORKS AND LEARNING SYSTEMS(2023)
摘要
Single object tracking (SOT) is one of the most active research directions in the field of computer vision. Compared with the 2-D image-based SOT which has already been well-studied, SOT on 3-D point clouds is a relatively emerging research field. In this article, a novel approach, namely, the contextual-aware tracker (CAT), is investigated to achieve a superior 3-D SOT through spatially and temporally contextual learning from the LiDAR sequence. More precisely, in contrast to the previous 3-D SOT methods merely exploiting point clouds in the target bounding box as the template, CAT generates templates by adaptively including the surroundings outside the target box to use available ambient cues. This template generation strategy is more effective and rational than the previous area-fixed one, especially when the object has only a small number of points. Moreover, it is deduced that LiDAR point clouds in 3-D scenes are often incomplete and significantly vary from frame to another, which makes the learning process more difficult. To this end, a novel cross-frame aggregation (CFA) module is proposed to enhance the feature representation of the template by aggregating the features from a historical reference frame. Leveraging such schemes enables CAT to achieve a robust performance, even in the case of extremely sparse point clouds. The experiments confirm that the proposed CAT outperforms the state-of-the-art methods on both the KITTI and NuScenes benchmarks, achieving 3.9% and 5.6% improvements in terms of precision.
更多查看译文
关键词
Point cloud compression,Target tracking,Proposals,Feature extraction,Object tracking,Laser radar,Task analysis,Computer vision,deep learning,point cloud processing,single object tracking (SOT)
AI 理解论文
溯源树
样例
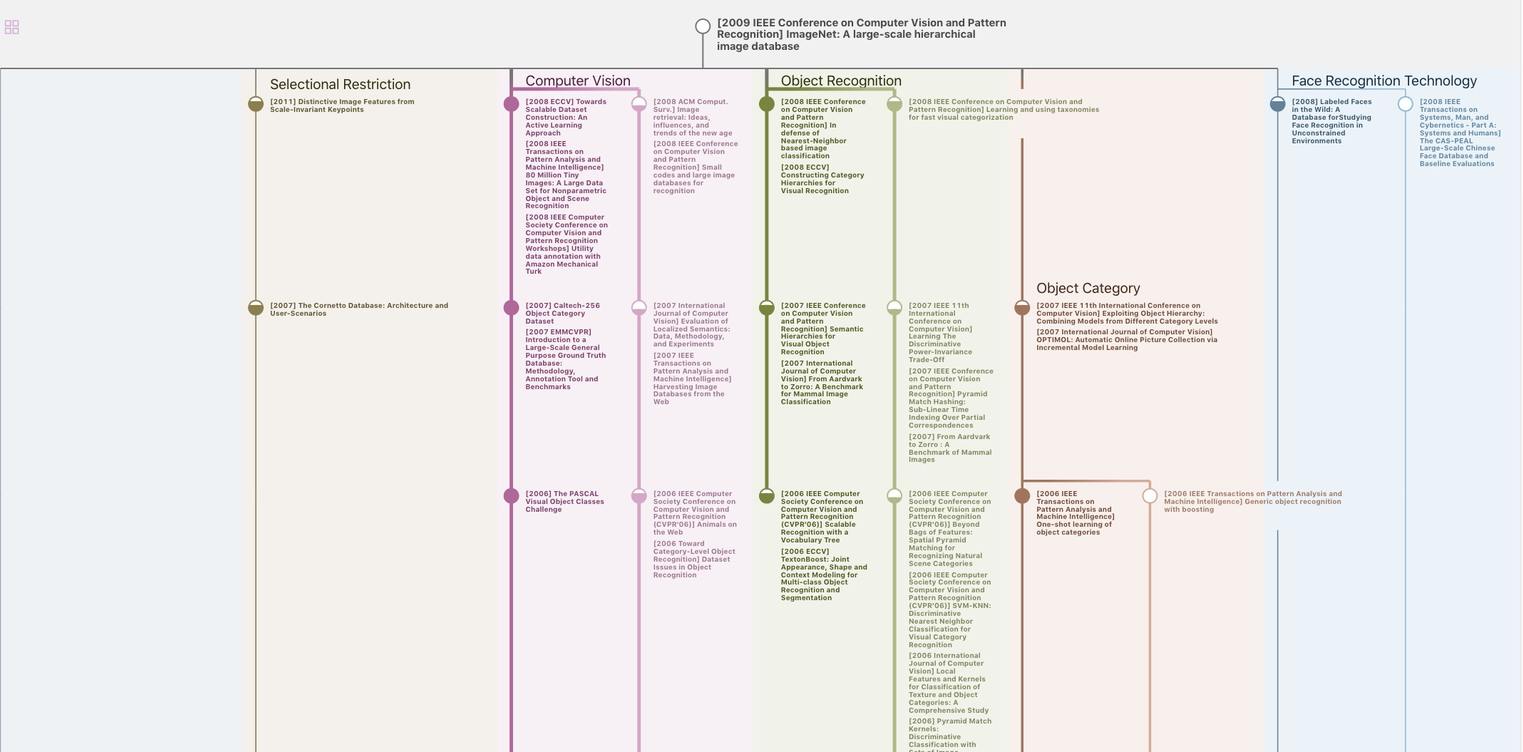
生成溯源树,研究论文发展脉络
Chat Paper
正在生成论文摘要