Cheating your apps: Black-box adversarial attacks on deep learning apps
JOURNAL OF SOFTWARE-EVOLUTION AND PROCESS(2023)
摘要
Deep learning is a powerful technique to boost application performance in various fields, including face recognition, image classification, natural language understanding, and recommendation system. With the rapid increase in the computing power of mobile devices, developers can embed deep learning models into their apps for building more competitive products with more accurate and faster responses. Although there are several works of adversarial attacks against deep learning models in apps, they all need information about the models' internals (i.e., structures and weights) or need to modify the models. In this paper, we propose an effective black-box approach by training substitute models to spoof the deep learning systems inside the apps. We evaluate our approach on 10 real-world deep-learning apps from Google Play to perform black-box adversarial attacks. Through the study, we find three factors that can affect the performance of attacks. Our approach can reach a relatively high attack success rate of 66.60% on average. Compared with other adversarial attacks on mobile deep learning models, in terms of the average attack success rates, our approach outperforms its counterparts by 27.63%.
更多查看译文
关键词
android,black-box attacks,deep learning apps
AI 理解论文
溯源树
样例
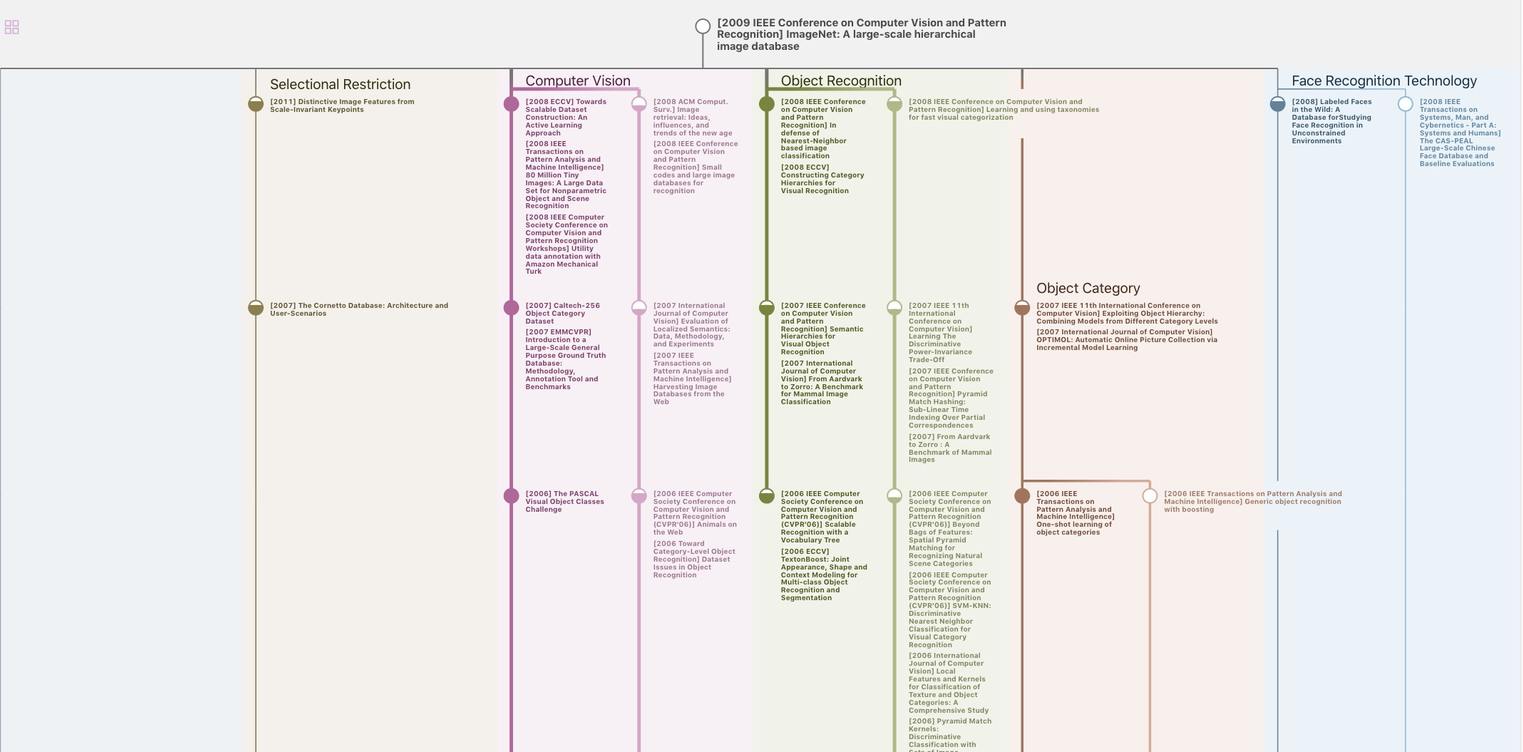
生成溯源树,研究论文发展脉络
Chat Paper
正在生成论文摘要