Novel mathematical and polypharmacology predictions of salicylsalicylic acid: Solubility enhancement through SCCO(2 )system
JOURNAL OF MOLECULAR LIQUIDS(2023)
摘要
Over the last decades, significant drawbacks of organic solvents such as high toxicity have motivated the scientists to find more eco-friendly solvents. Supercritical fluids (SCFs), especially SCCO2, are known as a promising class of solvent, which have shown their indisputable potential of application in industrial-based pharmaceutical activities due to possessing various advantages such as high abundancy, low cost, and insignificant toxicity. Machine Learning (ML) is considered as a numerical approach to estimate drug solubility in pharmaceutical industry. The purpose of this manuscript is to estimate the solubility of sal-icylsalicylic acid in SCCO2 and compare it with experimental data using machine learning (ML) approach. A regression problem with 32 input vectors is the subject of this study, which is being conducted. This dataset contains two input features (P and T) and one output feature. We utilized Decision Tree (DT), K-nearest neighbor (KNN), and Multilayer perceptron (MLP) regression models as the first time for sali-cylsalicylic acid, which had error rates of 1.10E-01, 1.07E-01, and 7.13E-01, respectively, when using the MAPE measure. In addition, the R-squared scores for the DT, KNN, and MLP models are 0.974, 0.996, and 0.809, respectively. The third statistic is MAE, in which the error rates of models are 5.27E-05 for DT, 5.53E-05 for KNN, and 2.61E-04 for MLP. The error rates of DT, KNN, and MLP are all 5.27E-05. Finally, KNN was the most general model, with optimal values of P = 400, T = 338.0, and Y = 0.00388 being obtained.(c) 2022 Elsevier B.V. All rights reserved.
更多查看译文
关键词
Solubility improvement,Machine learning,Simulation,Model prediction
AI 理解论文
溯源树
样例
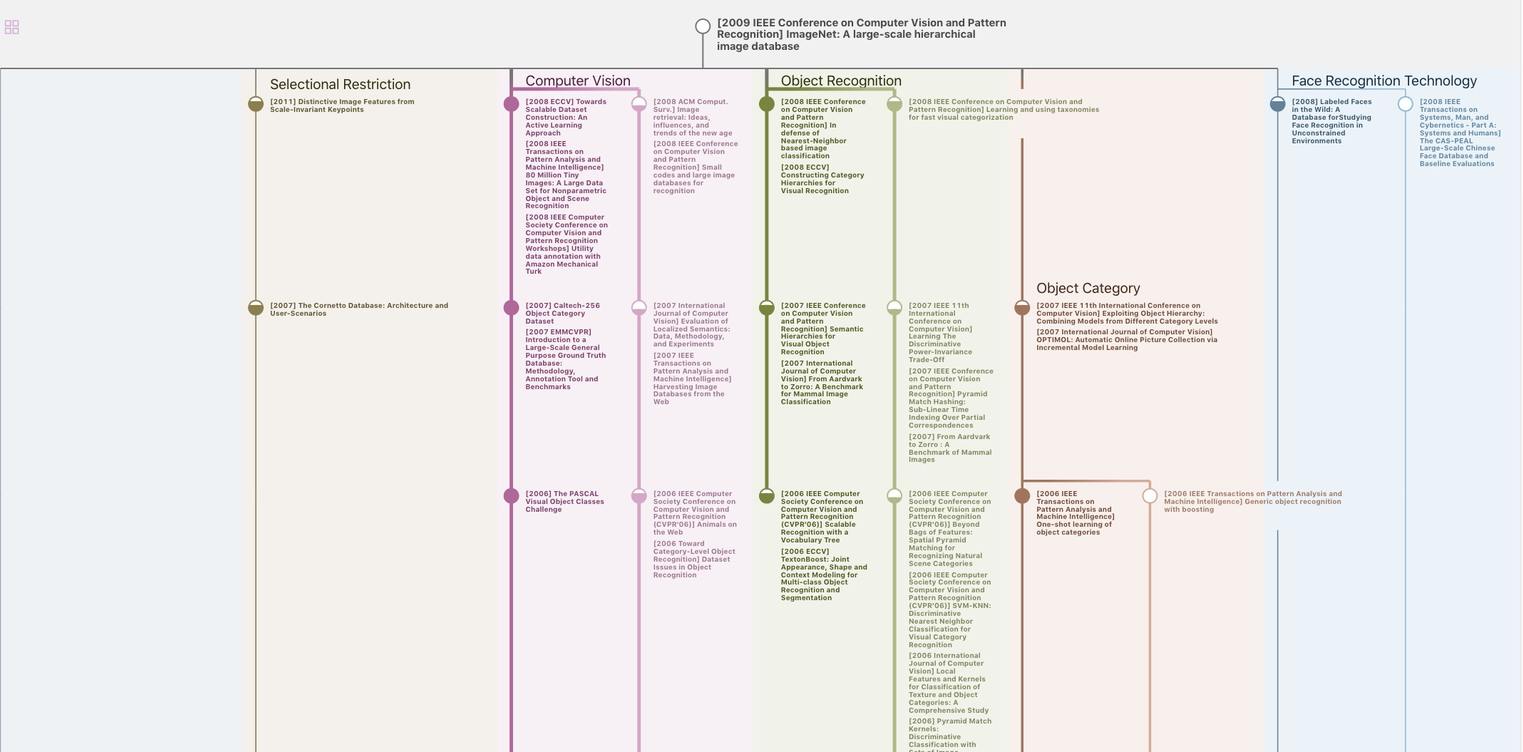
生成溯源树,研究论文发展脉络
Chat Paper
正在生成论文摘要