GM-lncLoc: LncRNAs subcellular localization prediction based on graph neural network with meta-learning
BMC GENOMICS(2023)
摘要
In recent years, a large number of studies have shown that the subcellular localization of long non-coding RNAs (lncRNAs) can bring crucial information to the recognition of lncRNAs function. Therefore, it is of great significance to establish a computational method to accurately predict the subcellular localization of lncRNA. Previous prediction models are based on low-level sequences information and are troubled by the few samples problem. In this study, we propose a new prediction model, GM-lncLoc, which is based on the initial information extracted from the lncRNA sequence, and also combines the graph structure information to extract high level features of lncRNA. In addition, the training mode of meta-learning is introduced to obtain meta-parameters by training a series of tasks. With the meta-parameters, the final parameters of other similar tasks can be learned quickly, so as to solve the problem of few samples in lncRNA subcellular localization. Compared with the previous methods, GM-lncLoc achieved the best results with an accuracy of 93.4 and 94.2% in the benchmark datasets of 5 and 4 subcellular compartments, respectively. Furthermore, the prediction performance of GM-lncLoc was also better on the independent dataset. It shows the effectiveness and great potential of our proposed method for lncRNA subcellular localization prediction. The datasets and source code are freely available at https://github.com/JunzheCai/GM-lncLoc .
更多查看译文
关键词
lncRNA,Subcellular localization,Graph neural network,Meta-learning,Classification
AI 理解论文
溯源树
样例
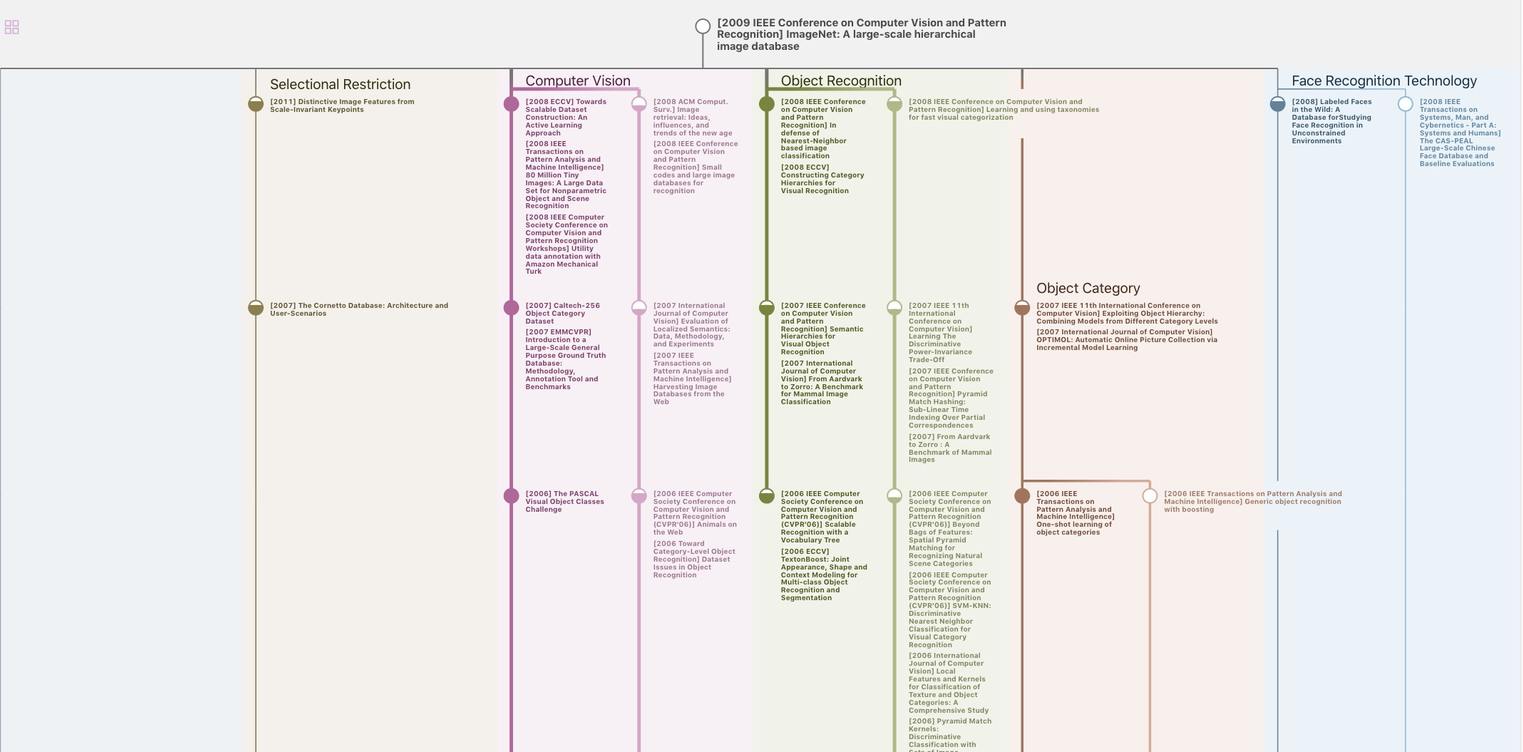
生成溯源树,研究论文发展脉络
Chat Paper
正在生成论文摘要