Research on performance predictions using single-hole film cooling based on PointNet
PHYSICS OF FLUIDS(2023)
摘要
A PointNet-based data-driven neural network model is proposed, which takes the film hole geometry variables and flow conditions as inputs to reconstruct the adiabatic cooling effectiveness distribution. The model aims to realize rapid reconstruction of the film cooling effectiveness field under complex and variable working conditions with a more flexible data organizational form. The dataset is derived from numerical simulations of the jet under crossflow. Select unstructured grid nodes are used to form point clouds for network training. The PointNet architecture includes two modules to extract the global features of the input point cloud and calculate the adiabatic efficiency. The responsiveness of the model to different variables is evaluated from the effectiveness contours, centerline, and laterally averaged effectiveness plots. Furthermore, correlation analysis is used to evaluate the accuracy of model predictions. Over the entire dataset, the mean correlation coefficient is 0.99, indicating that the model has a satisfactory ability to reconstruct and predict the effectiveness field. The main contribution from the area around the film holes to the cooling effectiveness distribution is further confirmed via critical point analysis.
更多查看译文
关键词
performance predictions,single-hole
AI 理解论文
溯源树
样例
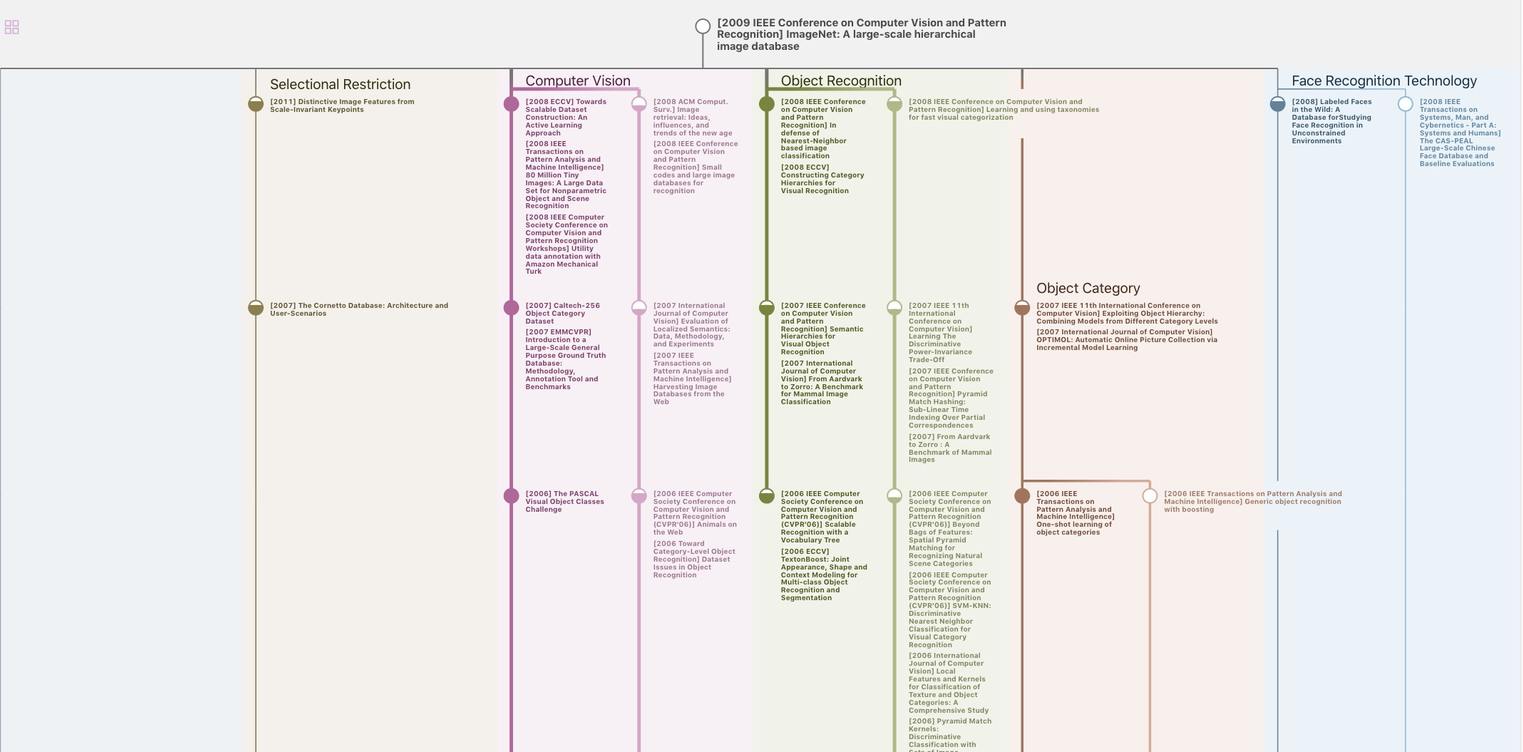
生成溯源树,研究论文发展脉络
Chat Paper
正在生成论文摘要