6G Connected Vehicle Framework to Support Intelligent Road Maintenance Using Deep Learning Data Fusion
IEEE TRANSACTIONS ON INTELLIGENT TRANSPORTATION SYSTEMS(2023)
摘要
The growth of IoT, edge and mobile Artificial Intelligence (AI) is supporting urban authorities exploit the wealth of information collected by Connected and Autonomous Vehicles (CAV), to drive the development of transformative intelligent transport applications for addressing smart city challenges. A critical challenge is timely and efficient road infrastructure maintenance. This paper proposes an intelligent hierarchical framework for road infrastructure maintenance that exploits the latest developments in 6G communication technologies, deep learning techniques, and mobile edge AI training approaches. The proposed framework abides with the stringent requirements of training efficient machine learning applications for CAV, and is able to exploit the vast numbers of CAVs forecasted to be present on future road networks. At the core of our framework is a novel Convolution Neural Networks (CNN) model which fuses imagery and sensory data to perform pothole detection. Experiments show the proposed model can achieve state of the art performance in comparison to existing approaches while being simple, cost-effective and computationally efficient to deploy. The proposed system can form part of a federated learning framework for facilitating large scale real-time road surface condition monitoring and support adaptive resource allocation for road infrastructure maintenance.
更多查看译文
关键词
Roads,Maintenance engineering,Data models,Computational modeling,6G mobile communication,Training,Smart phones,6G,deep learning,mobile edge intelligence,pothole detection,federated learning,intelligent transportation systems
AI 理解论文
溯源树
样例
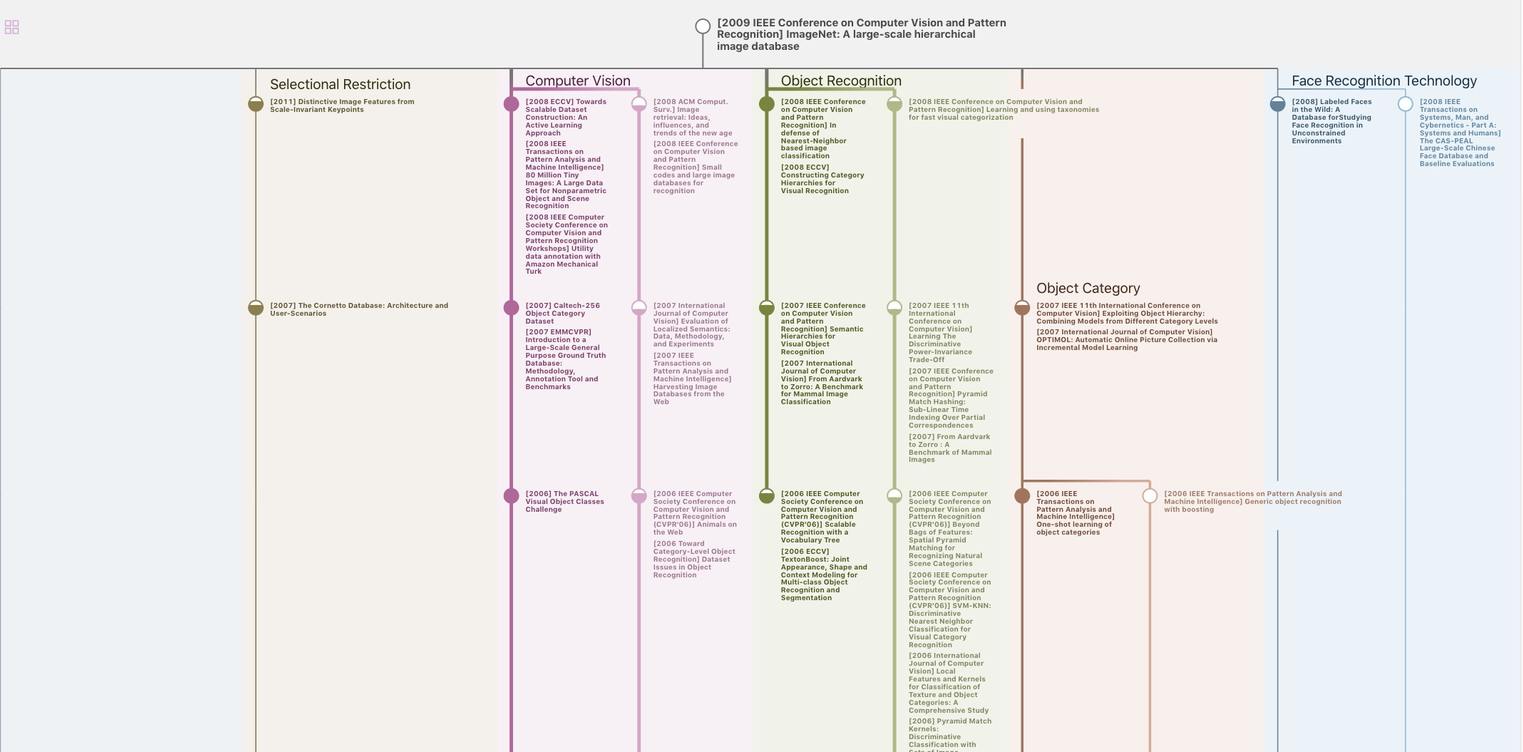
生成溯源树,研究论文发展脉络
Chat Paper
正在生成论文摘要