A comparative approach of ML algorithms to rank irrigation water quality: case of Oriental-Coast shallow aquifer in Cap-Bon, northeastern of Tunisia
MODELING EARTH SYSTEMS AND ENVIRONMENT(2023)
摘要
Groundwater quality evaluation was considered as one of the keys parameters to improve the water resources management, especially in regions where renewable water resources scarcity is a very serious problem. The Oriental Coast aquifer in Cap Bon, Northeastern of Tunisia is one of these regions where groundwater quality is in degradation. This study was carried out to predict Irrigation Water Quality Class (IWQC) using Machine Learning (ML) Algorithms: Support Vector Machines (SVMs), Decision Tree, K-Nearest Neighbor (KNN) and Bagging Classifier. The IWQC prediction was done based on five parameters controlling the overall water quality (pH, SAR, Cl − , HCO 3 − and NO 3 − ). In fact, primary models’ versions did not show reliable results because of overfitting and lack of data. To overcome these problems, data augmentation technique was applied to ameliorate our dataset. As final task, models’ performance was evaluated using 3 indicators: accuracy, recall and precision. The results revealed that data augmentation technique have contributed to enhance models’ performance and the modeling results showed that the Fine KNN model outperformed the other models (Accuracy = 97%, Recall = 0.97 and Precision = 0.92). Indeed, all the algorithms, with the exception of the Coarse KNN, overestimated IWQC.
更多查看译文
关键词
Irrigation water quality classification,Machine learning algorithms,Data augmentation,Model performance,Coastal aquifer
AI 理解论文
溯源树
样例
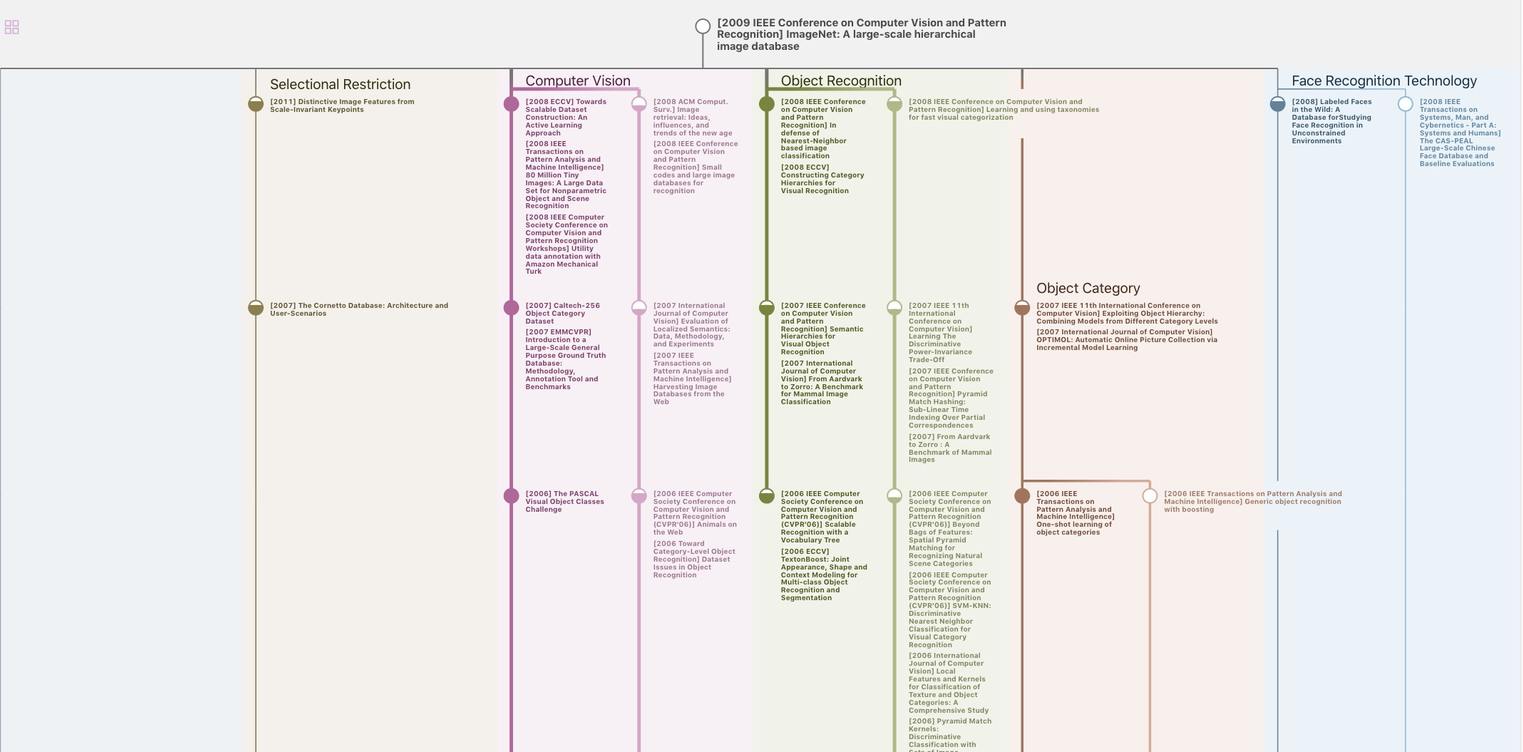
生成溯源树,研究论文发展脉络
Chat Paper
正在生成论文摘要