Underwater Image Detection and Recognition Using Radial Basis Function Neural Networks and Chimp Optimization Algorithm
CIRCUITS SYSTEMS AND SIGNAL PROCESSING(2023)
摘要
Radial basis function (RBF) neural network is one of the most practical tools in underwater image processing problems. Considering the deficiencies of RBF neural networks, such as low accuracy, slow convergence rate, and entrapment in local minima, we use the chimp optimization algorithm (ChOA) to tackle these deficiencies. Two benchmark underwater image datasets are used to evaluate the efficiency of the improved detector. Moreover, a dataset of experimental underwater images is created to test the RBF-ChOA detector’s ability to handle large underwater image datasets. To have a comprehensive approximation, the designed detector is compared to the Harris hawks optimization (HHO), slime mold algorithm (SMA), Kalman filter (KF), and Henry gas solubility optimization (HGSO) approach in terms of the detection accuracy, entrapment in local minima, and the convergence rate. According to the results, the suggested method outperforms previous RBF-based recognizers and, on average, recognizes underwater items 1.91% better than that of the top benchmark model.
更多查看译文
关键词
Underwater image processing,Neural network,Radial basis function,Chimp optimization algorithm
AI 理解论文
溯源树
样例
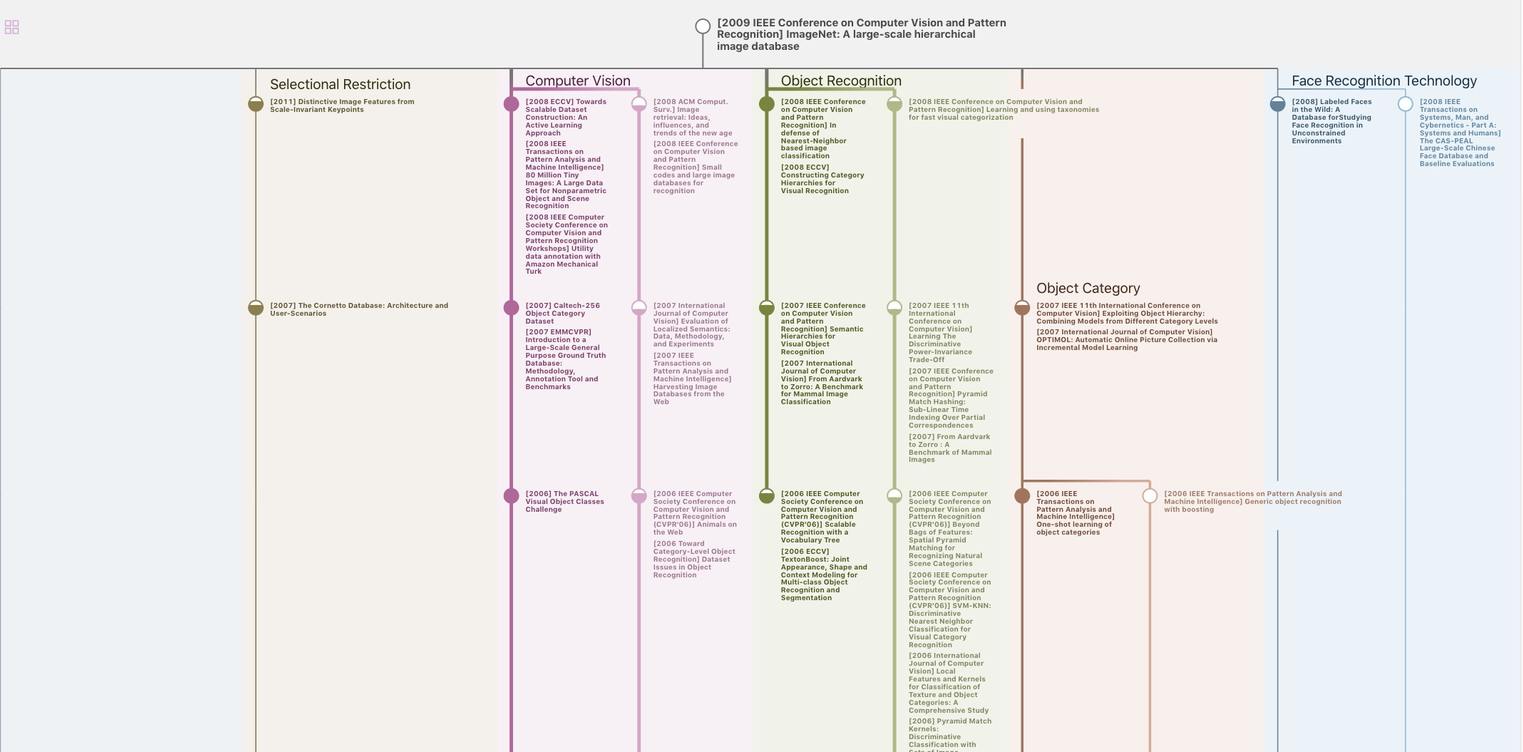
生成溯源树,研究论文发展脉络
Chat Paper
正在生成论文摘要