Review of machine learning methods applied to enhanced geothermal systems
ENVIRONMENTAL EARTH SCIENCES(2023)
摘要
The objective of this study was to summarize the progress in the application of machine learning (ML) to enhanced geothermal systems (EGSs), including the entire process of EGS survey, development, and application. This review formed descriptors after identifying keywords and searched the target literature from two highly-reputed and high-usage databases: Web of Science and Scopus. After filtering the duplicates between these databases, the remaining papers were filtered. Papers not on the application of ML to EGS or hot-dry-rock (HDR) fields were excluded. Subsequently, the remaining literature was read and studied. Papers on ML algorithms that have been applied to EGS systems were analyzed. ML methods have been applied to eight factors influencing EGS. Currently, the most common ML method is the artificial neural network (ANN). The Levenberg–Marguardt algorithm had a slightly better advantage over other neural network algorithms in terms of operation optimization and bottom-hole-temperature prediction for geothermal power stations. In the prediction of influencing factors, such as the reservoir temperature and drill speed, shallow ML models, such as XGBoost model based on the decision tree, random forest model, and support vector machine (SVM), often had better prediction ability than the ANN. More importantly, in reservoir temperature prediction, productivity prediction, and geological logging, deep learning (DL) models outperformed shallow ML models, such as ANN and SVM. Notably, in this study, the DL was only used in the prediction of the aforementioned influencing factors. When applied to other influencing factors under the same experimental conditions, the DL and other ML methods are expected to produce the same prediction results. Many factors influence EGS. This study discussed only eight influencing factors provided in the target literature. Other important factors, such as the prediction of reservoir fracture expansion and water loss, were not discussed because ML methods were not applied to them. The DL method can be used for predictions in the analysis of the complex influencing factors of this highly nonlinear relationship. Based on the application effect of DL in other fields, good prediction results can be expected.
更多查看译文
关键词
Enhanced geothermal system,Machine learning,Hot dry rock
AI 理解论文
溯源树
样例
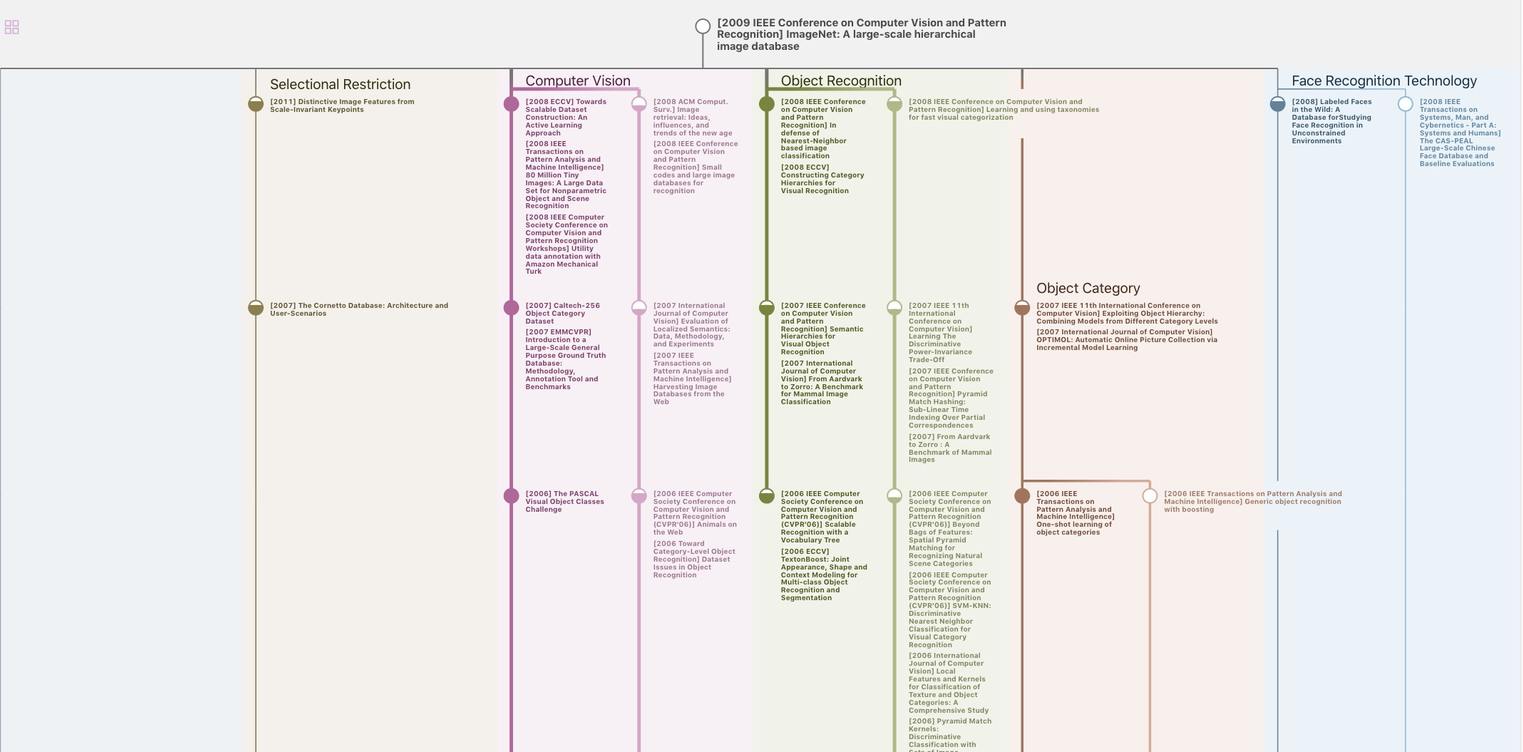
生成溯源树,研究论文发展脉络
Chat Paper
正在生成论文摘要