Analyzing Physics-Inspired Metaheuristic Algorithms in Feature Selection with K-Nearest-Neighbor
APPLIED SCIENCES-BASEL(2023)
摘要
In recent years, feature selection has emerged as a major challenge in machine learning. In this paper, considering the promising performance of metaheuristics on different types of applications, six physics-inspired metaphor algorithms are employed for this problem. To evaluate the capability of dimensionality reduction in these algorithms, six diverse-natured datasets are used. The performance is compared in terms of the average number of features selected (AFS), accuracy, fitness, convergence capabilities, and computational cost. It is found through experiments that the accuracy and fitness of the Equilibrium Optimizer (EO) are comparatively better than the others. Finally, the average rank from the perspective of average fitness, average accuracy, and AFS shows that EO outperforms all other algorithms.
更多查看译文
关键词
optimization,non-traditional algorithms,feature reduction,KNN,algorithms
AI 理解论文
溯源树
样例
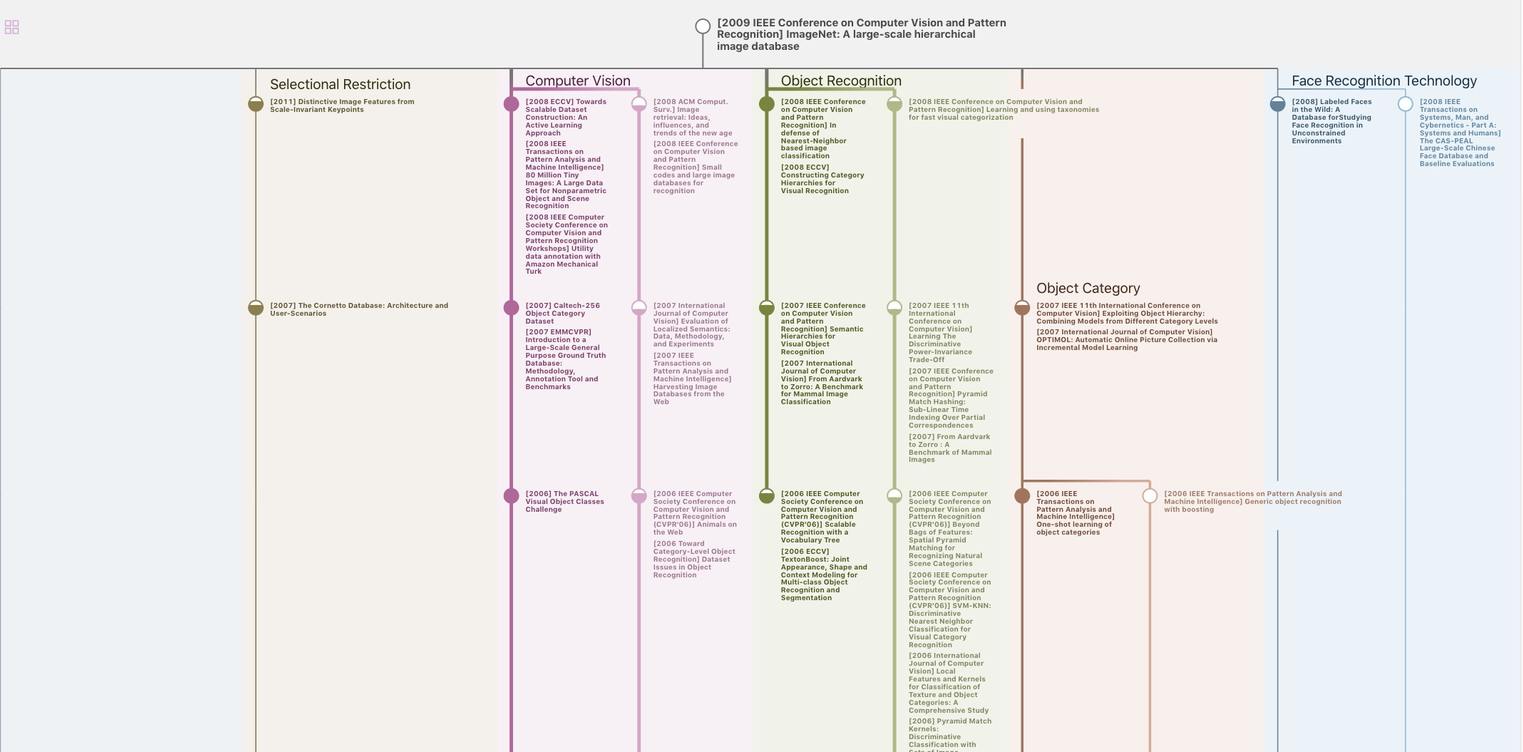
生成溯源树,研究论文发展脉络
Chat Paper
正在生成论文摘要